Statistical Tolerancing: Optimizing Quality & Manufacturing Efficiency
Quality, reliability, and cost-efficiency matter greatly, so the ability to accurately foresee and govern production process variation proves pivotal. Statistical tolerancing, rooted in sophisticated statistical techniques, offers a powerful toolkit helping engineers and quality experts meet this challenge head-on.
Through complex analyses and simulations, it enables comprehending and quantifying dimensional and geometric variation impacts on performance, assembly, and quality overall.
At its core, statistical tolerancing presents a systematic optimization method for tolerance specifications – allowed deviations from nominal dimensions or characteristics.
While conventional tolerancing analysis provides value, it often falls short of capturing modern manufacturing’s true intricacies.
Statistical tolerancing however embraces inherent variability in processes and materials, accounting for probabilistic variation nature and interconnected impacts.
By applying a more precise and holistic statistical approach, opportunities emerge to enhance outputs, streamline operations, and deliver stronger overall results.
Key Highlights
- Understand statistical tolerancing concepts, methods, and applications
- In-depth coverage of techniques such as 1D tolerance stack up, worst-case analysis, RSS (Root Sum Squared), and second-order tolerance analysis
- Exploration of the role of statistical tolerancing in Six Sigma and Design for Six Sigma (DFSS) initiatives
- Discussion of tolerance optimization strategies, including the Taguchi loss function method
- Insights into integrating statistical tolerancing with product design, assembly process optimization, and quality control
- Overview of software tools and their capabilities in tolerance analysis, variation simulation, and cost analysis
- Best practices for effective implementation, including early incorporation, cross-functional collaboration, and continuous improvement
- Case studies and examples from various industries, demonstrating quantifiable benefits
- Future trends and research areas, such as advanced tolerance analysis techniques, and Industry 4.0 integration.
What is Statistical Tolerancing and Why is it Important?
Statistical tolerance analysis emerges as a powerful technique to quantify and control variability.
Statistical tolerance analysis is a rigorous method that takes into account the inherent variations in input parameters and calculates the expected distribution of a critical output or performance characteristic.
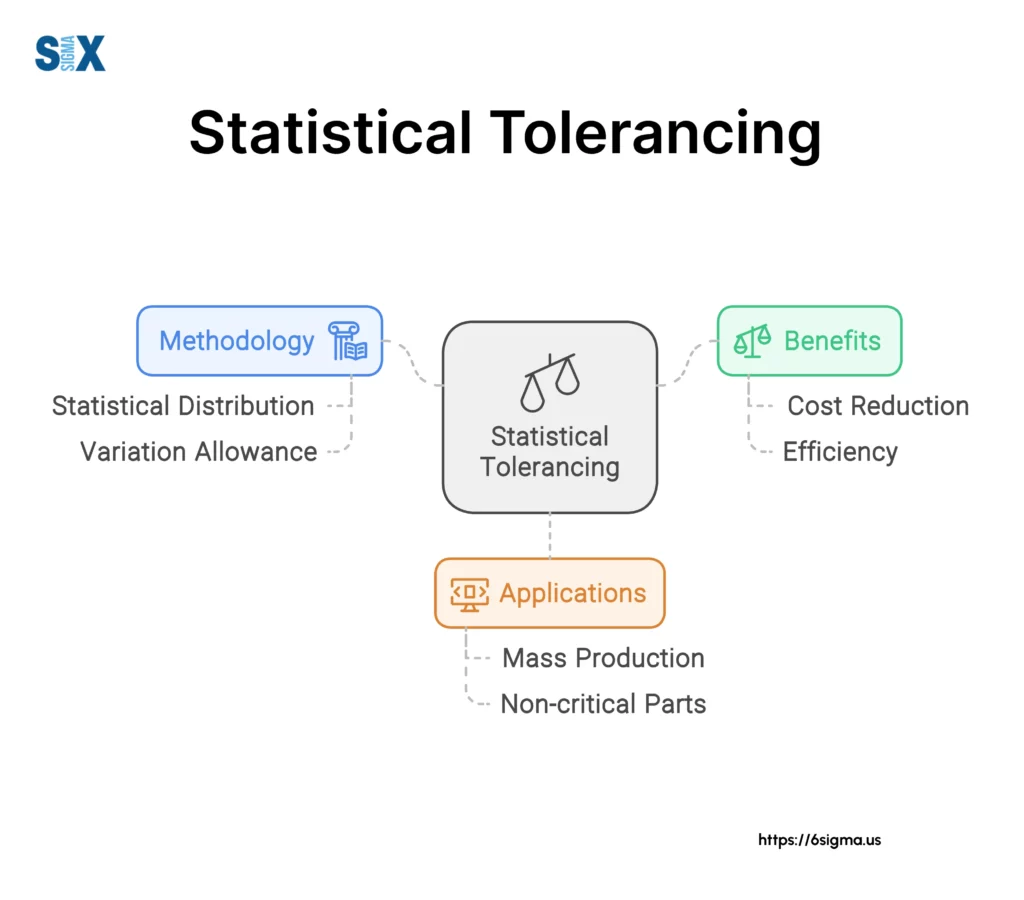
In the context of mechanical engineering, where products are composed of intricate assemblies with numerous features and tolerances, this analysis becomes indispensable.
Each component dimension or geometric characteristic is subject to some degree of variation due to manufacturing processes, material properties, and environmental factors.
Statistical tolerance analysis allows us to understand how these individual tolerances propagate and accumulate, ultimately impacting the overall product quality and functionality.
The benefits of statistical tolerancing are multifaceted, but two key advantages stand out:
Improved Product Quality
By accurately predicting the cumulative effects of variations, statistical tolerancing enables engineers to optimize tolerance specifications.
This optimization ensures that critical features and assemblies remain within acceptable limits, translating to higher product quality and reliability.
Reduced Defects
Traditional tolerance analysis methods often rely on worst-case scenarios, which can lead to overly conservative specifications and unnecessary costs.
Statistical tolerancing, on the other hand, provides a more realistic representation of the variation landscape, allowing for appropriate tolerance allocations.
This, in turn, minimizes the likelihood of defects and nonconformities, resulting in fewer rejects and reworks.
Key Concepts and Methods in Statistical Tolerancing
One of the foundational concepts in statistical tolerancing is the 1D tolerance stackup analysis.
1D tolerance Stackup analysis
This technique focuses on analyzing variations along a single linear dimension or axis.
By creating a cross-sectional view of the product or assembly, engineers can identify the critical features and tolerances that contribute to the overall dimensional variation.
The 1D tolerance stack-up analysis involves algebraically summing the individual tolerance values along the chosen axis, taking into account their respective distributions and interactions.
This approach provides a straightforward method for understanding how tolerances accumulate and impact the final dimension of interest.
Worst-case tolerance analysis
While not strictly a statistical tolerancing method, worst-case tolerance analysis is a valuable technique that serves as a baseline for comparison.
In this approach, each dimension or feature is assigned its maximum and minimum acceptable values, representing the extremes of the tolerance range.
By combining these worst-case scenarios, engineers can determine the maximum potential deviation or stackup for the assembly or product.
Although conservative, this method provides a quick assessment of whether the design can accommodate the most extreme variations without compromising functionality or performance.
Root Sum Squared (RSS)
The Root Sum Squared (RSS) statistical analysis is a widely used method in statistical tolerancing that accounts for the probabilistic nature of variations.
Unlike worst-case analysis, which considers only the extremes, RSS incorporates the distribution of each contributing dimension or feature.
The RSS method assumes that the input variations follow a normal (Gaussian) distribution and that the relationship between inputs and outputs is linear.
Six Sigma and Tolerance Design
In the context of Six Sigma methodologies, the defects per million opportunities (DPMO) metric plays a crucial role in assessing the performance of processes and products.
Defects per million opportunities (DPMO) metric
DPMO quantifies the average number of defects or non-conformities expected per million opportunities for a defect to occur.
This metric is closely tied to tolerance design, as the defect rate is directly influenced by how well the product or process adheres to specified tolerance limits.
Optimizing tolerance specifications
By optimizing tolerance specifications, manufacturers can effectively reduce the DPMO and achieve higher levels of Six Sigma performance, ultimately leading to improved quality and customer satisfaction.
Tolerance optimization is a fundamental aspect of tolerance design and is closely aligned with the principles of Six Sigma.
The goal of tolerance optimization is to strike a balance between achieving the desired functional requirements and minimizing the associated costs and risks.
Conventional tolerance design methods
Conventional tolerance design methods, such as the algebraic sum or worst-case analysis, have been widely used in industry for many years.
While these methods offer simplicity and a conservative approach, they often lead to overly restrictive tolerances and higher manufacturing costs.
Loss function method (Taguchi approach)
The loss function method, also known as the Taguchi approach, is a more sophisticated technique for tolerance design that aligns with the principles of Six Sigma.
This method incorporates the concept of quality loss and associated costs, recognizing that even conforming products may incur losses due to variability within the tolerance limits.
By quantifying the costs associated with deviations from the target value, the loss function method enables engineers to optimize tolerance specifications based on minimizing the overall quality loss and associated costs.
This approach provides a more comprehensive and data-driven framework for tolerance design, often resulting in more efficient and cost-effective solutions.
Applications of Statistical Tolerancing
Statistical tolerancing plays a crucial role in the product design and development phase, enabling engineers to proactively address potential quality issues and optimize tolerance specifications.
By incorporating statistical tolerancing analyses early in the design cycle, manufacturers can evaluate the impact of variations on product performance, identify critical dimensions and features, and make informed decisions regarding tolerance allocations.
This proactive approach not only enhances product quality and reliability but also streamlines the development process, reducing the need for costly design iterations and prototyping cycles.
Software Tools for Statistical Tolerancing
Modern statistical tolerancing software is often integrated with computer-aided design (CAD) systems, allowing for seamless data exchange and analysis directly within the 3D modeling environment.
This integration enables engineers to leverage geometric dimensioning and tolerancing (GD&T) information from the CAD models, streamlining the process of tolerance specification and analysis.
By working within the CAD environment, engineers can visualize and annotate critical dimensions and tolerances, facilitating effective communication and collaboration among cross-functional teams.
At the core of statistical tolerancing software are powerful tolerance analysis and variation simulation capabilities.
These tools allow engineers to model the propagation of variations through complex assemblies, accounting for the statistical distributions of individual component tolerances and their interactions.
By simulating thousands or millions of assembly scenarios, these software solutions can provide comprehensive insights into the expected range of variations in critical outputs, such as dimensional deviations, clearances, or interference risks.
This information is invaluable for optimizing tolerance allocations, identifying potential quality issues, and making informed design decisions.
Statistical Tolerancing optimization and cost analysis
By integrating cost models and quality loss functions, these software solutions can identify the optimal tolerance specifications that balance functional requirements, manufacturing capabilities, and associated costs.
This data-driven approach to tolerance optimization not only enhances product quality but also drives cost savings and improved competitiveness.
Several commercially available software solutions offer comprehensive statistical tolerancing capabilities.
Sigmetrix CETOL, Tecnomatix TS, and VisVSA
Sigmetrix CETOL (Cyber Tolerance Analysis and Optimization) is a widely recognized tool in the industry, providing advanced tolerance analysis, simulation, and optimization features for mechanical assemblies.
Tecnomatix Tolerance Analysis (TS) from Siemens PLM Software is another powerful solution that integrates with various CAD platforms and offers capabilities for tolerance stack-up analysis, variation simulation, and cost-based optimization.
VisVSA (Visual Variation Analysis and Optimization) from Variation Systems Analysis (VSA) is a specialized software package designed for statistical tolerancing in the automotive industry, with a focus on body assembly and dimensional quality control.
These software tools, along with others in the market, enable manufacturers to leverage the full potential of statistical tolerancing, driving quality improvements, cost savings, and increased competitiveness across various industries.
Best Practices and Implementation of Statistical Tolerancing
One of the key best practices in statistical tolerancing is to incorporate it early in the product design and development cycle.
By considering tolerance analysis and optimization from the outset, engineers can proactively address potential quality issues, minimize the need for costly redesigns, and streamline the overall development process.
Effective implementation of statistical tolerancing requires close collaboration between cross-functional teams, including design engineers, manufacturing experts, and quality professionals.
Each team brings unique perspectives and expertise, contributing to a holistic understanding of the product, manufacturing processes, and quality requirements.
By fostering open communication and knowledge sharing, these teams can collectively identify critical tolerances, assess manufacturing capabilities, and develop robust tolerance optimization strategies.
This collaborative approach ensures that design decisions are aligned with manufacturing realities and quality objectives, minimizing the risk of costly misalignments or quality issues down the line.
Accurate statistical tolerancing analyses and simulations rely heavily on high-quality data regarding manufacturing processes and material variations.
To ensure the reliability and validity of tolerance optimization efforts, it is crucial to conduct comprehensive data collection and process capability studies.
These studies involve collecting and analyzing data from actual production runs, measuring variations in critical dimensions, and quantifying the impact of factors such as tool wear, operator differences, and environmental conditions.
By incorporating this real-world data into statistical tolerancing models, manufacturers can achieve more realistic and effective tolerance allocations, ultimately leading to improved product quality and reduced costs.
Statistical tolerancing is not a one-time effort but rather an ongoing process of continuous improvement and refinement.
As manufacturing processes evolve, new materials are introduced, or product designs are modified, tolerance specifications and allocations may need to be revisited and optimized.
By embracing a culture of continuous improvement, manufacturers can regularly review and refine their tolerance optimization strategies, leveraging lessons learned, best practices, and the latest advancements in statistical tolerancing techniques.
This iterative approach ensures that tolerance specifications remain aligned with changing conditions, enabling sustained quality improvements and cost savings over the product lifecycle.
Case Studies for Statistical Tolerancing
Numerous companies across various industries have successfully implemented statistical tolerancing initiatives, yielding significant benefits in terms of product quality, cost savings, and competitive advantage.
Case studies from automotive manufacturers, for example, showcase how statistical tolerancing analyses enabled optimized tolerance allocations for critical engine components, leading to improved fuel efficiency, reduced emissions, and enhanced reliability.
In the aerospace sector, statistical tolerancing has played a pivotal role in ensuring the structural integrity and aerodynamic performance of aircraft components, contributing to increased safety and operational efficiency.
Electronics manufacturers have also leveraged statistical tolerancing to optimize tolerances in printed circuit board (PCB) designs, resulting in improved signal integrity, reduced rework, and enhanced product functionality.
While statistical tolerancing offers numerous benefits, its successful implementation requires careful planning and attention to potential pitfalls.
Lessons learned from real-world projects highlight the importance of comprehensive data collection, cross-functional collaboration, and a thorough understanding of manufacturing processes and their inherent variations.
Future Trends and Research in Statistical Tolerancing
As production grows sophisticated and design pushes innovation boundaries, advanced tolerance examination needs to increase too.
Researchers actively explore capturing non-linear ties, intricate material behaviors, and geometries’ complex interlinking.
Emerging techniques may involve simulation unification – computational fluid dynamics, and finite element analysis illuminating tolerance propagation intricacies.
Industry 4.0 drives a manufacturing paradigm shift toward connectivity, automation, and data-led decisions.
Statistical tolerancing stands to play a pivotal role through smart manufacturing sync, leveraging connected systems’ vast data.
Constant monitoring and controlling by Internet of Things, advanced sensors permits dynamic optimization and tolerance change guided by variation and capability updates.
3D printing gains ground for geometries’ complexity and customization abilities hampered less by past limitations.
Tailored statistical tolerancing studies consider factors like orientation, supports, and post-steps.
Robust design insights into crucial tolerances’ propagation through systems emerge too.
Integrating statistical analyses and optimization algorithms with techniques like Taguchi facilitates quality-focused, variation-resilient products lowering overly tight tolerance costs.
In conclusion, statistical tolerancing powerfully connects engineering, production, and quality management.
Cutting-edge software and statistical techniques unlock unprecedented quality, efficiency, and competitiveness.
Future possibilities through deeper analytics, technology-meshing, and robust design-linking promise ever-smarter solutions amid rising demands.
Statistical tolerancing indispensably enables makers to navigate precision, dependability, and novelty global marketplace demands.
SixSigma.us offers both Live Virtual classes as well as Online Self-Paced training. Most option includes access to the same great Master Black Belt instructors that teach our World Class in-person sessions. Sign-up today!
Virtual Classroom Training Programs Self-Paced Online Training Programs