Quantitative Risk Analysis: A Lean Six Sigma Approach to Effective Risk Management
Quantitative risk analysis sits at the core of modern decision-making with its quantitative approach to turning uncertainty into measurable, actionable data points.
Breaking Down Quantitative Risk Analysis (QRA)?
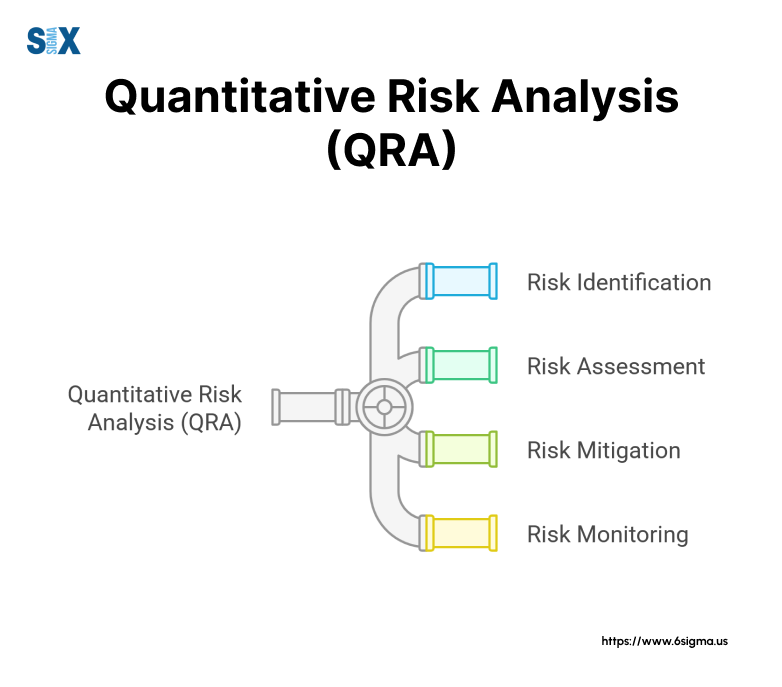
Quantitative risk analysis (QRA) helps evaluate project and business risks with a numerical approach. It provides concrete numbers and probabilities to drive effective decision-making in comparison to qualitative methods that use subjective assessment(s).
The Importance of Lean Six Sigma and Modern Business
You can’t run a business on just gut feelings, you need an approach that can quantify potential risks that can be translated into probability and impact. Allowing precise resource allocation and risk mitigation strategies.
Quantitative risk analysis extends beyond mere number-crunching. It serves as the bridge between raw data and strategic decision-making.
Quantitative Risk Analysis (QRA) in the DMAIC Framework
QRA integrates with the Define-Measure-Analyze-Improve-Control (DMAIC) to drive great results. Here’s how it typically flows:
- Define: We identify potential risks and establish measurement criteria
- Measure: We gather historical data and current metrics
- Analyze: We apply statistical methods to quantify risk probabilities
- Improve: We implement data-driven risk mitigation strategies
- Control: We monitor and adjust our risk management approach based on ongoing analysis
I remember a particular project where we implemented this framework to assess supply chain risks. By quantifying the probability and impact of various disruption scenarios, we developed a robust risk management strategy that saved the company millions in potential losses.
Fundamentals of Quantitative Risk Analysis
Understanding the fundamentals of quantitative risk analysis is crucial for successful project outcomes.
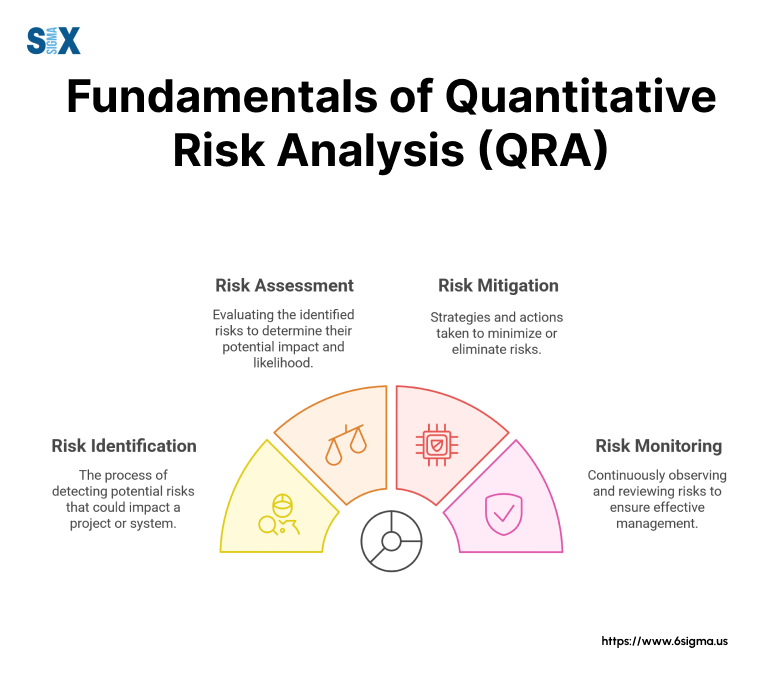
Key Principles of QRA
The foundation of quantitative risk analysis rests on three core principles: measurability, reproducibility, and objectivity.
Use a structured approach that transforms abstract risks into measurable metrics. This involves assigning numerical values to both the probability of risk occurrence and its potential impact on project objectives.
For instance, a major telecommunications company assessing their supply chain risks used historical data and statistical modeling to quantify the likelihood of delivery delays and their financial impact.
This quantitative assessment enabled them to make data-driven decisions about inventory levels and supplier diversification.
Differences between Qualitative and Quantitative Risk Analysis
One of the most common questions is about choosing between qualitative and quantitative approaches. While qualitative risk analysis relies on descriptive terms like “high,” “medium,” or “low,” quantitative risk assessment provides specific numerical values and probabilities.
In my experience, start with qualitative assessment for initial screening, then apply quantitative analysis to critical risks that warrant deeper investigation.
For example, when evaluating manufacturing process improvements, we might qualitatively identify potential failure modes, but then use quantitative methods to calculate specific probabilities and financial impacts.
When to Use Quantitative Risk Analysis in Lean Six Sigma Projects
The timing of quantitative risk analysis is crucial for project success. Based on my experience implementing Six Sigma across various organizations, I recommend performing quantitative analysis during these key phases:
- Early project planning: When establishing project feasibility and resource requirements
- Before major investment decisions: To evaluate ROI and potential losses
- During process improvement initiatives: To measure the impact of proposed changes
- In continuous monitoring: To track risk metrics and trigger response plans
I recall a particular project where we initially skipped quantitative analysis during a major supply chain transformation. The oversight led to unexpected delays and costs.
After implementing proper quantitative risk assessment, we were able to identify and mitigate similar risks in subsequent phases, saving millions in potential losses.
The key is to match the depth of analysis with the decision’s importance. Develop and use a tiered approach: using basic quantitative methods for routine decisions and more sophisticated analysis for strategic initiatives.
This balanced approach ensures efficient resource utilization while maintaining robust risk management.
Quantitative Risk Analysis Techniques in Lean Six Sigma
Mastering quantitative risk analysis methods is crucial for project success. Let me share some of the most effective techniques.
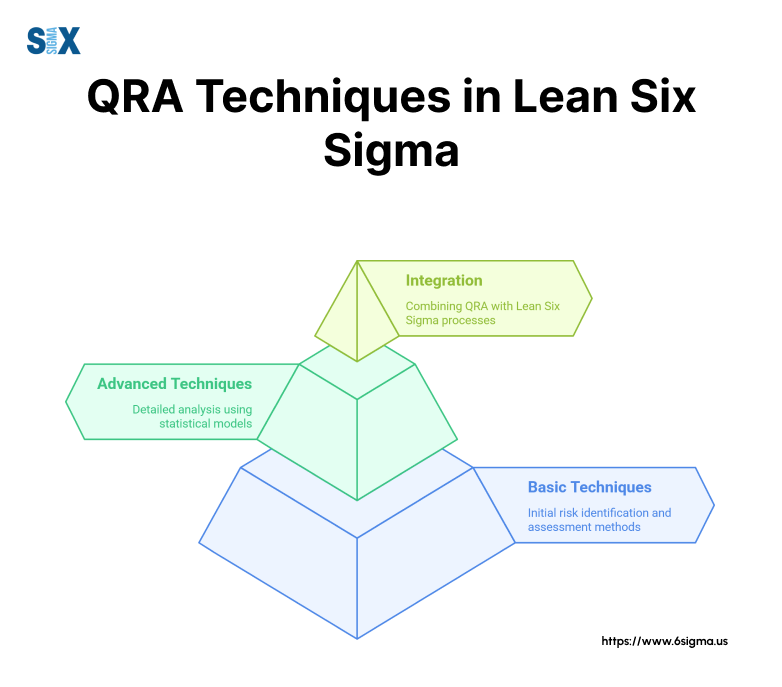
Failure Mode and Effects Analysis (FMEA)
FMEA stands as one of the most powerful quantitative risk analysis methods in the Lean Six Sigma toolkit. The approach involves quantifying three critical factors: severity, occurrence, and detection.
To conduct an FMEA effectively, follow these steps:
- Identify potential failure modes
- Determine severity ratings (1-10)
- Assess occurrence probability (1-10)
- Evaluate detection capability (1-10)
- Calculate Risk Priority Number (RPN) = Severity × Occurrence × Detection
I recall a particular project where FMEA helped us identify a critical design flaw that could have cost millions in warranty claims. By quantifying the risks early, we implemented preventive measures before production began.
Monte Carlo Simulation
Monte Carlo simulation represents a sophisticated approach to quantitative risk analysis. This technique uses repeated random sampling to obtain numerical results and predict project outcomes.
The process involves:
- Defining the parameter ranges and distributions
- Running thousands of iterations
- Analyzing the probability distribution of outcomes
During a recent semiconductor manufacturing project, we used Monte Carlo simulation to optimize production parameters, resulting in a 30% reduction in defect rates. The key is to ensure your input distributions accurately reflect real-world conditions.
Decision Tree Analysis
In my experience leading international projects, decision tree analysis has proven invaluable for complex decision-making scenarios. This quantitative method helps visualize and calculate the expected value of different decision paths.
When I worked with GE’s power division, we used decision trees to evaluate investment options for new turbine technologies. The process involves:
- Mapping out decision points and possible outcomes
- Assigning probabilities to each branch
- Calculating expected values for each path
- Comparing alternative scenarios
Expected Monetary Value (EMV) Analysis
EMV analysis forms the backbone of quantitative risk assessment in financial decision-making. Here’s a straightforward formula:
EMV = Probability of Event × Impact Value
For instance, EMV analysis can be used to prioritize investments across different regions. The key is to gather accurate probability estimates and impact values through historical data and expert judgment.
Let’s take an example of deciding between two suppliers, each with different risk profiles. Using EMV analysis:
Supplier A: (0.9 × $1M) + (0.1 × -$2M) = $700,000
Supplier B: (0.95 × $800K) + (0.05 × -$1M) = $710,000
This analysis helped us choose Supplier B, despite its lower potential upside, due to its better risk-adjusted return.
Implementing Quantitative Risk Analysis in Lean Six Sigma Projects
Implementing Six Sigma across global organizations, I’ve found that successful quantitative risk analysis requires a structured approach aligned with the DMAIC methodology. Let’s walk through how to effectively perform quantitative risk assessment at each phase.
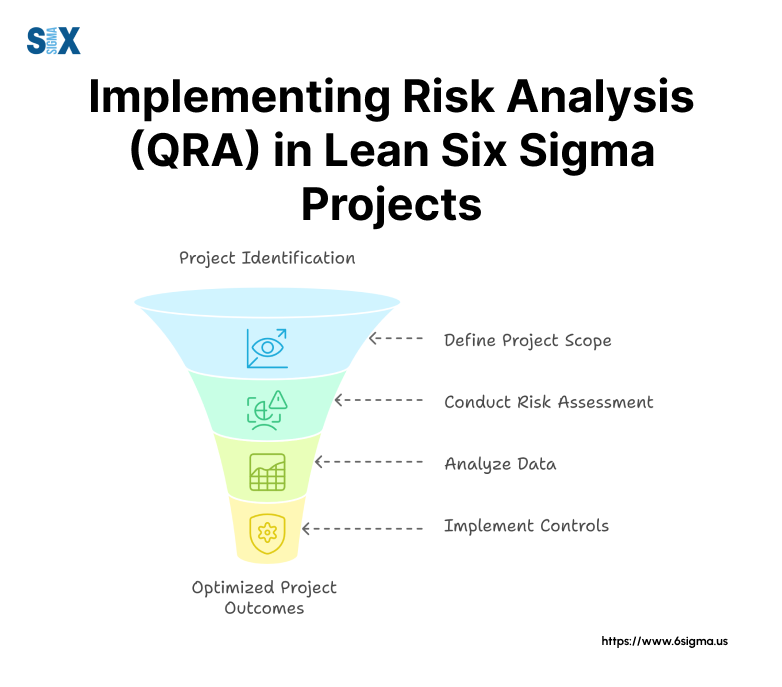
Define Phase: Identifying Risks
The foundation of effective quantitative risk analysis begins with proper risk identification. For this, use a systematic approach to risk identification that combines historical data analysis with stakeholder input.
Start by clearly defining your project scope and objectives, then identify potential risks that could impact these goals.
Measure Phase: Quantifying Risks
In the measure phase, we transform identified risks into quantifiable metrics. This involves collecting historical data, establishing measurement systems, and defining risk parameters. A robust measurement framework includes:
- Probability of occurrence (based on historical data)
- Impact severity (in terms of cost, time, or quality)
- Detection capability (measured through process controls)
Analyze Phase: Prioritizing Risks
The analyze phase is where quantitative risk analysis truly shines. Using statistical tools and probability theory, we evaluate and prioritize risks based on their expected impact.
For this, implement a risk prioritization matrix that combines probability scores with impact values to generate risk priority numbers (RPNs).
Improve Phase: Developing Risk Mitigation Strategies
With prioritized risks in hand, the improve phase focuses on developing and implementing mitigation strategies. Use cost-benefit analysis to evaluate different mitigation options. The key is to ensure that the cost of mitigation does not exceed the expected value of risk reduction.
Control Phase: Monitoring and Reviewing Risks
The final step in quantitative risk analysis isn’t just monitoring – it’s establishing a dynamic risk management system. Implement a continuous monitoring framework that includes:
- Regular risk metric reviews
- Threshold-based trigger points
- Automated data collection systems
- Periodic reassessment of risk priorities
This system can help identify emerging risks before they become critical issues, saving millions in potential losses.
When implementing quantitative risk analysis in your Lean Six Sigma projects, focus on creating sustainable processes that can be maintained long after the initial implementation. This ensures that risk management becomes an integral part of your organization’s operational excellence journey.
Using Quantitative Risk Analysis in a Lean Six Sigma Project
Proper risk assessment can transform project outcomes and deliver significant business value. Let’s get ahead and know more about how it should be implemented.
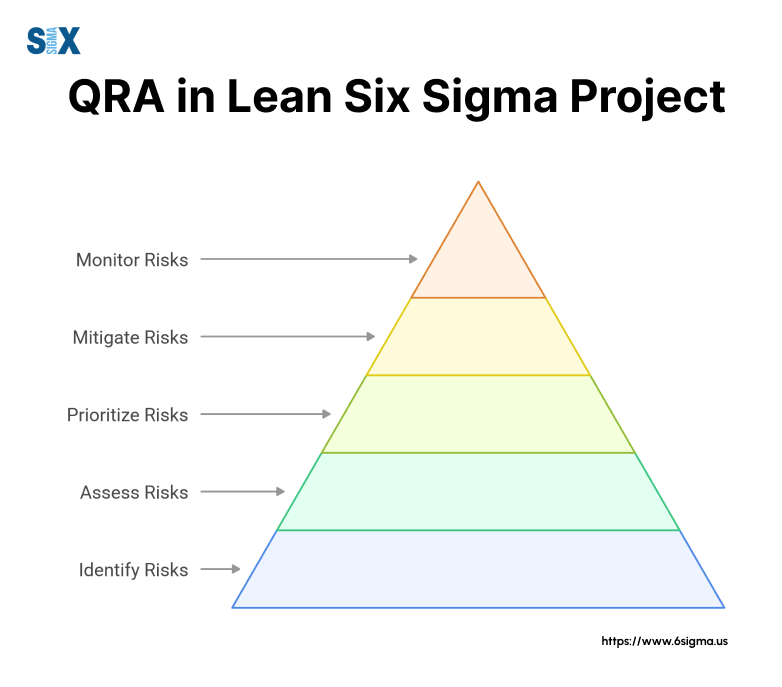
Project Background
During my consulting work, we faced a critical challenge in their microprocessor production line. The facility was experiencing unexpected downtime that cost approximately $100,000 per hour. The project goal was to reduce downtime by 50% within six months while maintaining product quality standards.
This presented an ideal opportunity to demonstrate the power of quantitative risk assessment in a high-stakes environment. The complexity of the manufacturing process, combined with the significant financial implications, made it essential to develop a data-driven approach to risk management.
Risk Identification and Quantification
Begin by identifying potential risks through a structured process that combines historical data analysis with expert interviews. We identified three primary quantifiable risks:
- Equipment Failure Risk
- Historical frequency: 3 failures per month
- Average downtime: 4 hours per failure
- Cost impact: $300,000 per incident
- Quality Control Issues
- Defect rate: 2.5% above target
- Rework costs: $550,000 monthly
- Customer impact: $1.2 million in potential penalties
- Supply Chain Disruptions
- Probability of critical component delay: 15%
- Average delay impact: $1 million per occurrence
Analysis and Decision-Making
Using Monte Carlo simulation, we modeled various scenarios to understand the potential impact of different risk mitigation strategies. Our quantitative risk analysis revealed that equipment failure posed the highest expected monetary value of risk at $3 million annually.
We developed a decision matrix that compared three potential solutions:
- Enhanced preventive maintenance program ($600,000 investment)
- Redundant equipment installation ($1.5 million investment)
- Hybrid approach combining both strategies ($2 million investment)
The quantitative analysis showed that the enhanced preventive maintenance program offered the best return on investment, with an expected risk reduction of 65% and a payback period of four months.
Results and Lessons Learned
The implementation of our chosen strategy, guided by quantitative risk assessment, delivered remarkable results:
- 60% reduction in unplanned downtime
- $3.2 million annual cost savings
- 40% decrease in quality-related issues
Key lessons learned from this project include:
- The importance of comprehensive data collection in quantifying risks
- The value of using multiple risk assessment techniques
- The need for regular reassessment of risk parameters
This case study demonstrates how quantitative risk analysis can drive concrete business improvements. By transforming abstract risks into measurable metrics, we enabled better decision-making and achieved significant cost savings.
Tools and Technologies for Quantitative Risk Analysis
I’ve seen the evolution of risk management tools from basic spreadsheets to sophisticated software solutions. While some might find quantitative analysis intimidating, the right tools can significantly simplify the process.
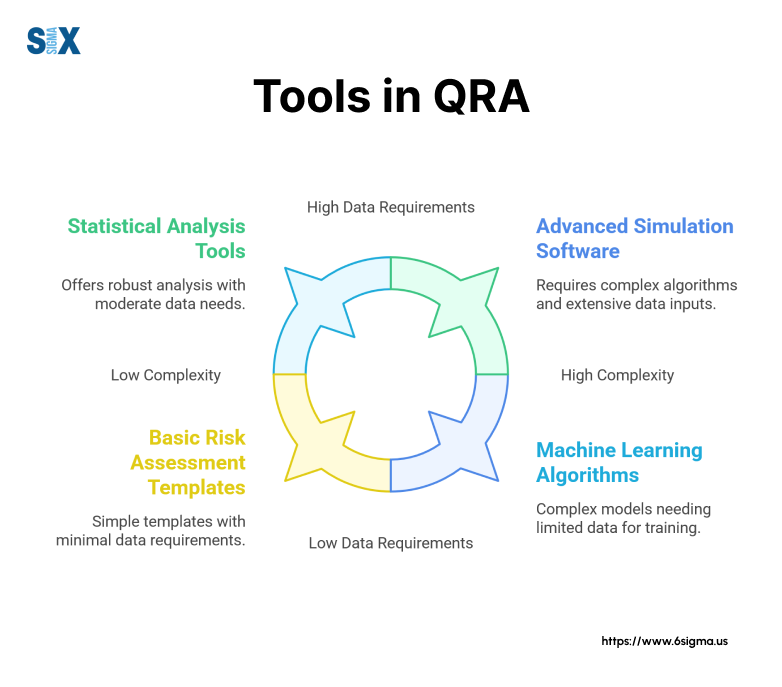
Lean Six Sigma-specific Software Tools
I’ve worked extensively with specialized Six Sigma software tools that integrate quantitative risk analysis capabilities. Minitab has been particularly valuable for its statistical analysis features and risk modeling capabilities.
Another powerful tool I’ve implemented across multiple organizations is JMP, developed by SAS. The visual analytics capabilities made it easier for teams to understand complex risk patterns and relationships.
General-purpose Statistical Software
For broader statistical analysis needs, I’ve found tools like R and Python increasingly valuable in quantitative risk analysis. You can develop custom R scripts for Monte Carlo simulations that help evaluate complex supply chain risks.
While these tools have a steeper learning curve, they offer unparalleled flexibility and power for sophisticated risk modeling.
SPSS and SAS Enterprise are other robust options. These platforms prove invaluable for analyzing clinical trial risks and regulatory compliance issues.
Spreadsheet Applications for QRA
Don’t underestimate the power of well-designed spreadsheet applications. Start with Excel-based tools because they’re accessible and familiar to most professionals.
You can create a comprehensive risk assessment dashboard in Excel that includes:
- Risk probability matrices
- Impact calculations
- Automated risk scoring
- Trend analysis charts
The key to successful tool implementation lies not in choosing the most sophisticated option, but in selecting the right tool for your organization’s needs and capabilities. I’ve seen companies waste resources on complex software solutions when simpler tools would have sufficed.
Is quantitative analysis hard?
In my experience, the challenge isn’t in the analysis itself but in choosing the right tools and ensuring proper training. I always advise organizations to start with simpler tools and gradually progress to more sophisticated solutions as their team’s capabilities grow.
Remember, tools are only as effective as the people using them. I’ve found that successful quantitative risk analysis depends more on understanding fundamental principles than on mastering complex software. Start with the basics, ensure your team is comfortable with the selected tools, and build complexity gradually.
Best Practices for Quantitative Risk Analysis in Lean Six Sigma
I’ve identified certain best practices that consistently lead to successful outcomes. These insights, gathered from my experience have proven invaluable in maximizing the benefits of quantitative assessment while avoiding common pitfalls.
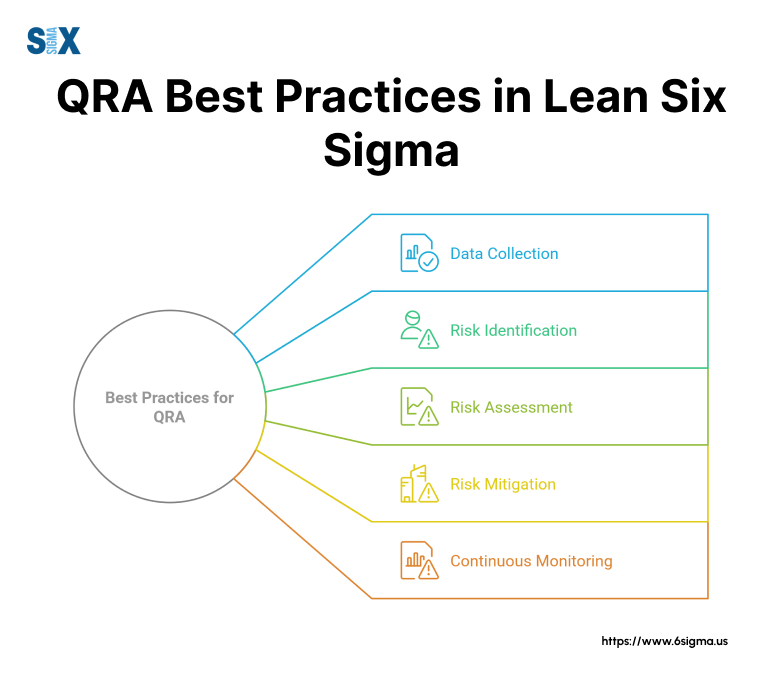
Data Collection and Validation
The foundation of effective quantitative risk management lies in robust data collection and validation processes. The key is to ensure data quality at every step.
Implement a structured data validation protocol that includes:
- Source verification
- Statistical testing for data quality
- Cross-functional data review sessions
- Historical trend analysis
This systematic approach to data management improves risk prediction accuracy.
Stakeholder Involvement
One of the most valuable lessons I’ve learned is that successful quantitative risk analysis requires active stakeholder engagement. Establish a stakeholder communication matrix that ensures all relevant parties contribute their expertise to the risk assessment process.
By involving stakeholders from engineering, finance, and operations, you can identify critical risks that might have been missed with a siloed approach. This comprehensive stakeholder involvement leads to more accurate risk quantification and better-informed decision-making.
Continuous Monitoring and Updating
Quantitative risk analysis isn’t a one-time exercise. Through my experience implementing Six Sigma methodologies, I’ve found that continuous monitoring and regular updates are crucial for maintaining effectiveness.
Implement a dynamic risk monitoring system that includes:
- Regular review cycles
- Real-time risk metric tracking
- Automated alert mechanisms
- Periodic reassessment of risk parameters
Integration with Other Lean Six Sigma Tools
The true power of quantitative risk analysis emerges when it’s integrated with other Lean Six Sigma tools. For instance, we combined quantitative risk analysis with process capability studies and value stream mapping to create a comprehensive improvement framework.
This integrated approach delivered significant benefits:
- More accurate risk assessments
- Better resource allocation
- Improved project outcomes
- Enhanced decision-making capability
The benefits of quantitative assessment extend beyond mere risk management. This approach leads to a reduction in project failures and an improvement in resource utilization.
Remember, the goal isn’t just to generate numbers but to create actionable insights that drive better business decisions.
Success lies in maintaining a balance between rigorous analysis and practical application. As I often tell participants in my workshops, “The most sophisticated analysis is worthless if it doesn’t lead to better decisions.” Focus on creating sustainable processes that deliver real value to your organization.
Common Challenges and Solutions in QRA for Lean Six Sigma
Numerous challenges can hinder successful implementation. Let’s look at some common pitfalls and the effective solutions to address them.
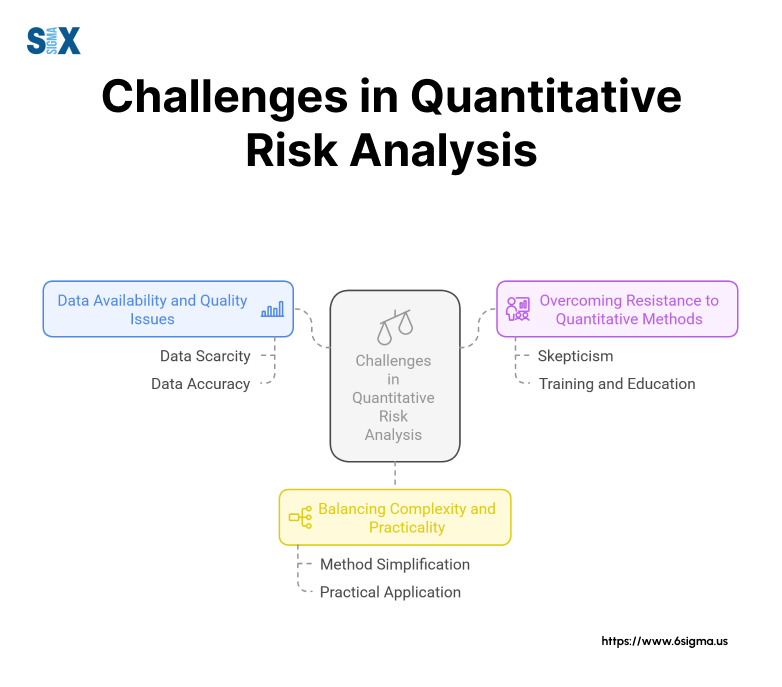
Data Availability and Quality Issues
One of the most persistent challenges in quantitative risk assessment is obtaining reliable, comprehensive data. The key to overcoming this challenge lies in developing robust data collection frameworks.
I recall a particular project where we faced significant data quality issues. Our solution involved implementing a three-tier data validation system:
- First, we established clear data collection protocols and standardized measurement systems.
- Next, we implemented automated data validation checks to flag anomalies.
- Finally, we created cross-functional review teams to verify data integrity.
This systematic approach improved our data quality by 55% within three months.
Overcoming Resistance to Quantitative Methods
Resistance to quantitative methods often stems from unfamiliarity and perceived complexity. To address this challenge, use a staged implementation approach:
- Started with simple risk quantification methods
- Demonstrated early wins through pilot projects
- Provided hands-on training and mentoring
- Gradually introduced more sophisticated techniques
This approach helped us achieve over 90% stakeholder buy-in across multiple projects.
Balancing Complexity and Practicality
One of the most critical challenges in risk quantification is finding the right balance between analytical rigor and practical applicability. Excessive complexity could paralyze decision-making rather than enhance it.
The solution lies in the “practical precision principle.” You can implement this approach by:
- Focusing on critical risks with significant impact
- Using appropriate levels of analytical detail
- Ensuring results are actionable
- Maintaining clear communication channels
This balanced approach can help reduce analysis time while maintaining accuracy in risk assessment.
Common Pitfalls to Avoid
I’ve identified several recurring pitfalls:
- Over-reliance on historical data without considering future scenarios
- Failing to update risk parameters as conditions change
- Ignoring qualitative inputs that could inform quantitative analysis
- Using overly complex models when simpler ones would suffice
The key to successful quantitative risk analysis lies not in avoiding these challenges but in addressing them systematically. Use a comprehensive framework for managing these issues, which should include regular review cycles, stakeholder feedback loops, and continuous improvement mechanisms.
Remember, the goal of quantifying risk isn’t to achieve perfect precision but to make better-informed decisions. As I often tell participants in our classes, “It’s better to be approximately right than precisely wrong.”
Going Ahead
The journey from basic risk assessment to sophisticated quantitative analysis represents a fundamental shift in how organizations approach uncertainty and manage their operations.
Recap of Key Points
The key takeaways from our discussion include the importance of:
- Data-driven decision-making in risk assessment
- Integration of quantitative methods with Lean Six Sigma principles
- Balanced approach to complexity and practicality
- Continuous monitoring and adaptation of risk parameters
The Role of QRA in Successful Lean Six Sigma Implementation
I see quantitative risk analysis becoming increasingly central to Lean Six Sigma’s success. Organizations that effectively integrate quantitative risk analysis into their Six Sigma initiatives consistently achieve superior results.
As we continue to face increasingly complex business challenges, the ability to quantify and manage risks effectively will become even more crucial for organizational success.
SixSigma.us offers both Live Virtual classes as well as Online Self-Paced training. Most option includes access to the same great Master Black Belt instructors that teach our World Class in-person sessions. Sign-up today!
Virtual Classroom Training Programs Self-Paced Online Training Programs