Design of Experiments: Plackett–Burman designs
Many scientific trials involve various influences potentially shaping results. Screening tactics like Plackett-Burman designs optimize probing them when budgets and hours prove limited.
Plackett-Burman emerged through statisticians’ work in the ‘40s as a proficient, economical technique within the Design of Experiments (DoE) pinpointing prime movers.
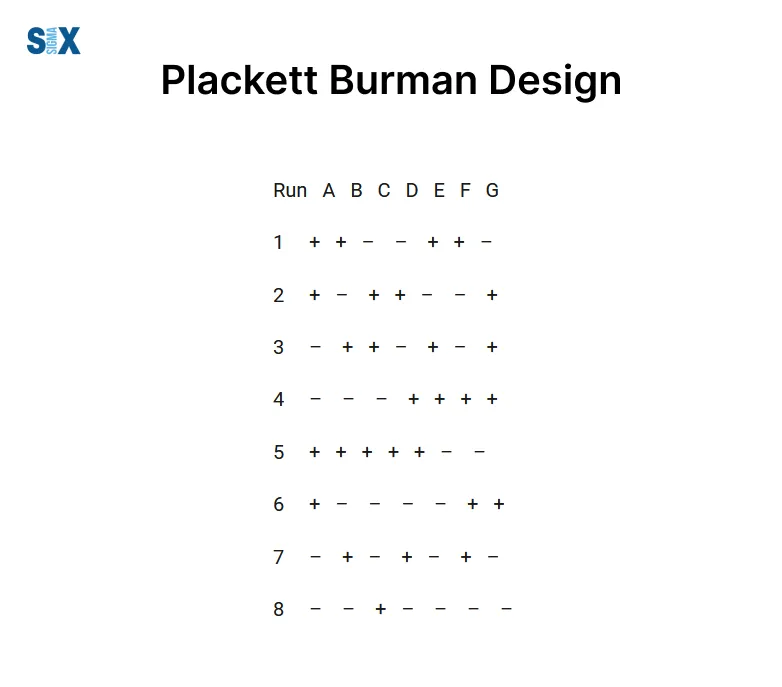
It lets researchers expeditiously gauge numerous determinants utilizing comparatively slight repeats. Particularly helpful amid abundance awaiting assessment, its end flags the foremost few necessitating investigation.
By this first-pass filtering, concentration shifts optimally. The main effects dominating interactions chiefly at screening stages underlie Plackett-Burman’s presumptions.
None optimize alone amid evolving imperatives – our joined comprehension elevates every pursuit. By cooperative modernizations amid unforeseen complexities ahead, all champion prosperity upholding benefit for all. Progress indeed follows shared betterment paths as one.
Key Highlights
- Plackett-Burman screening’s deftness lies in pinpointing primaries through quicker, thriftier trials. Its efficiency uncovers the most influential factors among many through compressed tests.
- Pinpointing the impactful few remains its aim where abundance potentially influences results. Calculated impartiality against interference lets main impacts isolation without other element convolutions.
- Plackett-Burman main effect derivation and vitals branding employ stat examinations. While unable to estimate interplays and assuming three-variable interaction insignificance, first-pass filtration upgrades remain its mastery.
- None optimize alone amid evolving needs – united comprehension elevates proficiency. Their criteria and restraints acknowledged such designs prove helpful harbinger works.
- Future betterments unlock through cooperation, as impediments ahead surface jointly surmounted. Progress pursues cooperation paths, serving people’s latest demands through persistently improving means collaboratively.
What is Plackett-Burman Designs?
Plackett-Burman trials present a screening approach within the Design of Experiments (DoE). Their economic examination of factors up to k=N-1 among N repeats (when evenly divisible by four) renders them popular.
Screenings including Plackett-Burman seek the strong few from many likely influencers. They efficiently explore an abundance through limited repeats, pinpointing primaries deserving focused optimization.
Developed by statisticians Robin Plackett and J.P. Burman in ’46, these designs reliably and affordably gauge the extent of variables. Especially valuable with numerous potentials and limited budgets, their resolution ensures distinct main effects.
Common applications involve:
- Key manufacturing parameters
- Formulation’s critical components
- Top quality determinants
- Foremost chemical reactions
- Important biological drivers
Acknowledging restraints, these screenings prove helpful starting points. Future refinement unlocks through cooperation; none improves alone amid evolving needs. Together, understanding lifts enterprises to serve people continually.
Plackett-Burman Design Basics
The Plackett-Burman design is a type of two-level fractional factorial design used in screening experiments. It allows you to study up to N-1 factors in N experimental runs, where N is a multiple of 4. The design is very economical and efficient for screening a large number of factors to identify which ones have significant main effects.
The key characteristics of a Plackett-Burman design include:
- Two-Level Factors: Each factor is tested at two levels, a high (+1) and low (-1) setting. This allows you to estimate the main effects but not the interaction effects.
- Resolution III Design: It is a resolution III fractional factorial design. This means main effects are not confounded or aliased with any other main effect, but they are aliased with two-factor interactions.
- Foldover Pairs: The design matrix is constructed from the first N runs and then “folded over” to get the remaining N runs by reversing the signs. This creates foldover pairs of runs.
- Design Generators: The design generators determine how the runs are aligned with the two-factor interactions. Proper selection of generators is important.
The design matrix of a Plackett-Burman experiment has N rows (runs) and N-1 columns representing the factors being screened. The entries are simply +1 and -1, indicating the high and low levels for each factor and run combination.
While economical, Plackett-Burman designs do have limitations in that they cannot estimate interaction effects and can only screen several factors equal to one less than a multiple of 4. However, they are very useful for initial factor screening when many factors are involved.
Creating a Plackett-Burman Design
The Plackett-Burman design allows you to efficiently study up to k=N-1 factors in N experiment runs, where N is a multiple of 4. This makes it a very economical screening design when you have a large number of potential factors to investigate.
To create a Plackett-Burman design, you first need to determine the appropriate design size N based on the number of factors k you want to estimate the main effects for. Common design sizes are N=8 runs for 7 factors, N=12 runs for 11 factors, and N=20 runs for 19 factors.
Once you have selected the design size N, the design matrix can be generated using what are known as “design generators”. These are specific mathematical algorithms that create the +1 and -1 factor level combinations for each run optimally. The design generators ensure that the design is balanced so that all main effects are uncorrelated and can be estimated independently.
The design matrix will have N runs as the rows, and k+1 columns. The first column is a dummy column of +1s, and the remaining k columns correspond to the k factors being screened. Each column will have an equal number of +1 and -1 entries.
It’s important to properly randomize and replicate the Plackett-Burman design runs to ensure valid conclusions. Randomization protects against any systematic biases, while replication allows you to obtain an estimate of pure experimental error.
In addition to the design generators, there are tables and software that can quickly generate the design matrix for common Plackett-Burman designs. Minitab, JMP, and other DOE software packages have built-in utilities for creating these designs.
Analyzing Plackett-Burman Experiments
Once you have conducted your Plackett-Burman screening experiment, the next step is to analyze the results to determine which factors are potentially important.
The analysis involves calculating the main effects and making judgments about which effects are large enough to be considered active and worthy of further investigation.
Main Effects
The primary analysis for a Plackett-Burman design calculates the main effects, which estimate the impact of each factor averaged across all levels of the other factors.
Main effects are calculated by contrasting the response averages when a factor is at its high and low levels. Larger main effect values indicate factors that are more influential on the response.
Statistical Significance Testing
To judge whether a main effect is large enough to be considered active and worthy of further study, statistical significance testing is used.
The null hypothesis is that the main effect equals zero (the factor has no effect). Effects larger than a critical value are considered statistically significant evidence that the factor is active and impacting the response. The critical value depends on the significance level, number of effects tested, and error degrees of freedom.
Effect Magnitude and Ranking
In addition to statistical significance, the practical magnitude of effects is also considered when identifying active factors.
Effects are typically ranked from largest to smallest in absolute value. The largest effects, regardless of statistical significance, are considered potentially important for further evaluation.
Interaction Effects
Though less common, it is also possible to estimate two-factor interaction effects with a Plackett-Burman design.
However, this requires higher resolution designs that avoid confounding interactions with main effects. Significant interactions indicate that the impact of one factor depends on the level of another factor.
Normal Probability Plots
Normal probability plots of the effects are a useful diagnostic tool. Active effects will be scattered away from the normal line, while inactive effects cluster along the line. This visual analysis can supplement or replace formal statistical tests.
Plackett-Burman designs provide an efficient way to screen many factors simultaneously to identify the few active factors. Proper analysis of the main effects is crucial to take full advantage of this experimental strategy. The identified active factors can then be further explored and optimized through additional experiments.
Plackett-Burman Design Examples
To better understand how Plackett-Burman designs work, let’s walk through a couple of examples.
Example 1: Screening 7 Factors in 8 Runs
Suppose an engineer wants to study the effects of 7 different factors (A, B, C, D, E, F, G) on a particular process output or response.
Using a Plackett-Burman design, this can be accomplished in just 8 experimental runs rather than the 128 runs required for a full 2^7 factorial design.
The design matrix for this 8-run Plackett-Burman design is:
Run A B C D E F G
1 + + – – + + –
2 + – + + – – +
3 – + + – + – +
4 – – – + + + +
5 + + + + + – –
6 + – – – – + +
7 – + – + – + –
8 – – + – – – –
The design is constructed such that each factor is tested at two levels (+/-) across the 8 runs. The plus and minus signs represent the high (+) and low (-) settings for each factor.
By running these 8 experiments and analyzing the results, the engineer can identify which of the 7 factors have a statistically significant effect on the response. The factors found insignificant can be dropped, allowing further experimentation to focus only on the vital few factors.
Example 2: Plackett-Burman for Process Optimization
A food scientist is studying how 11 ingredient and process variables (A-K) impact the taste and texture of a baked good product. To screen all 11 factors using a Plackett-Burman design, a 12-run design is selected.
The design matrix looks like this:
Run A B C D E F G H I J K
1 + + + – – – – + + + +
2 – + + + – – – – + + +
… (10 more runs not shown)
The plus and minus signs represent the high and low settings for each factor tested over the 12 experimental runs.
Analysis of the results identifies factors B, D, G, and J as having a significant impact on the product’s sensory characteristics. Further optimization experiments can then focus just on these 4 key factors.
These examples illustrate how Plackett-Burman screening designs enable efficient testing of many factors in relatively few runs. They allow the vital few factors to be identified from a larger set of potential factors.
Plackett-Burman vs Other DOE Methods
The Plackett-Burman design is just one type of experimental design used in DOE (design of experiments). It’s important to understand how it compares to some other popular DOE techniques:
Plackett-Burman vs Full Factorial
A full factorial design looks at all possible combinations of factor levels. This allows estimation of all main effects and interactions but requires a large number of runs, especially as the number of factors increases. The Plackett-Burman is a fraction of the full factorial that focuses only on the main effects of reducing the number of required runs.
Plackett-Burman vs Fractional Factorial
Like the Plackett-Burman, fractional factorial designs are a fraction of the full factorial experiment.
However, fractional factorials can estimate the main effects and some interactions, while the Plackett-Burman estimates only the main effects. Fractional factorials require more runs than Plackett-Burman for the same number of factors.
Plackett-Burman vs Response Surface Methods
Response surface methods like central composite and Box-Behnken designs are used when the objective is to optimize a response by mapping it over the factor ranges. The Plackett-Burman is mainly used for screening to identify the critical factors before optimization.
Plackett-Burman vs Taguchi Methods
Taguchi methods like the L8, L9, etc. orthogonal arrays are also used for screening and optimizing quality characteristics. Compared to Plackett-Burman, Taguchi designs look at interactions in addition to main effects but require more runs for the same number of factors.
The Plackett-Burman design is an economical screening design well-suited for studying main effects when resources are limited. It complements other DOE methods focused on interactions, optimizing responses, or quality improvement.
Limitations and Assumptions
While Plackett-Burman designs are efficient screening designs, there are some important limitations and assumptions to be aware of:
Assumptions
- The design assumes that there are no active interactions between factors. If interactions do exist, the main effects will be aliased or combined with the interaction effects, making them difficult to interpret.
- It assumes that all main effects are of the same magnitude and follow approximately a normal distribution. Highly skewed or non-normal data can violate this assumption.
- The design assumes that the factors are measured quantitatively and can be set at two levels (high and low).
Limitations
- Plackett-Burman cannot estimate interaction effects. It can only identify the few vital main effects from many potential factors.
- The design has limited flexibility in run sizes. The number of experimental runs is a multiple of 4 rather than the ideal multiple of 2.
- The design has a resolution of III, meaning that the main effects are aliased or combined with two-factor interactions. This confounding pattern limits the ability to study interactions.
- As the number of factors increases, the number of runs required increases rapidly, which can become impractical when studying many factors.
- The design is directional, meaning flipping the high and low levels changes the sign of the estimated effects.
- Plackett-Burman cannot be used for quantitative factor settings or to fit a response surface model.
To address these limitations, fractional factorial or response surface designs may be more appropriate if the objective is to estimate interactions, fit a quadratic model, or study a large number of factors economically.
However, for the screening of many factors to identify the vital few, Plackett-Burman designs provide an efficient way to gather useful information with a relatively small number of runs.
SixSigma.us offers both Live Virtual classes as well as Online Self-Paced training. Most option includes access to the same great Master Black Belt instructors that teach our World Class in-person sessions. Sign-up today!
Virtual Classroom Training Programs Self-Paced Online Training Programs