Mastering Parametric Estimation: A Comprehensive Guide for Project Managers
Project managers and cost estimators face a constant challenge: predicting project costs and timelines accurately.
While various estimation methods exist, parametric estimation stands out as a data-driven approach that delivers reliable results through statistical analysis and historical data.
Parametric estimation uses mathematical models and statistical relationships to calculate project costs, duration, and resource requirements.
Key Highlights
- The mechanics of parametric estimation and its role in project planning
- Step-by-step process for implementing parametric estimates
- Statistical formulas and real-world calculation examples
- Modern tools and software that streamline estimation
- Future developments in estimation technology
What is Parametric Estimation?
Parametric estimation transforms project planning through statistical analysis and historical data.
This method calculates project costs, duration, and resource needs using mathematical relationships between project variables.
For example, construction firms use cost-per-square-foot data to estimate expenses for new buildings, while software companies calculate development hours based on the number of user stories.
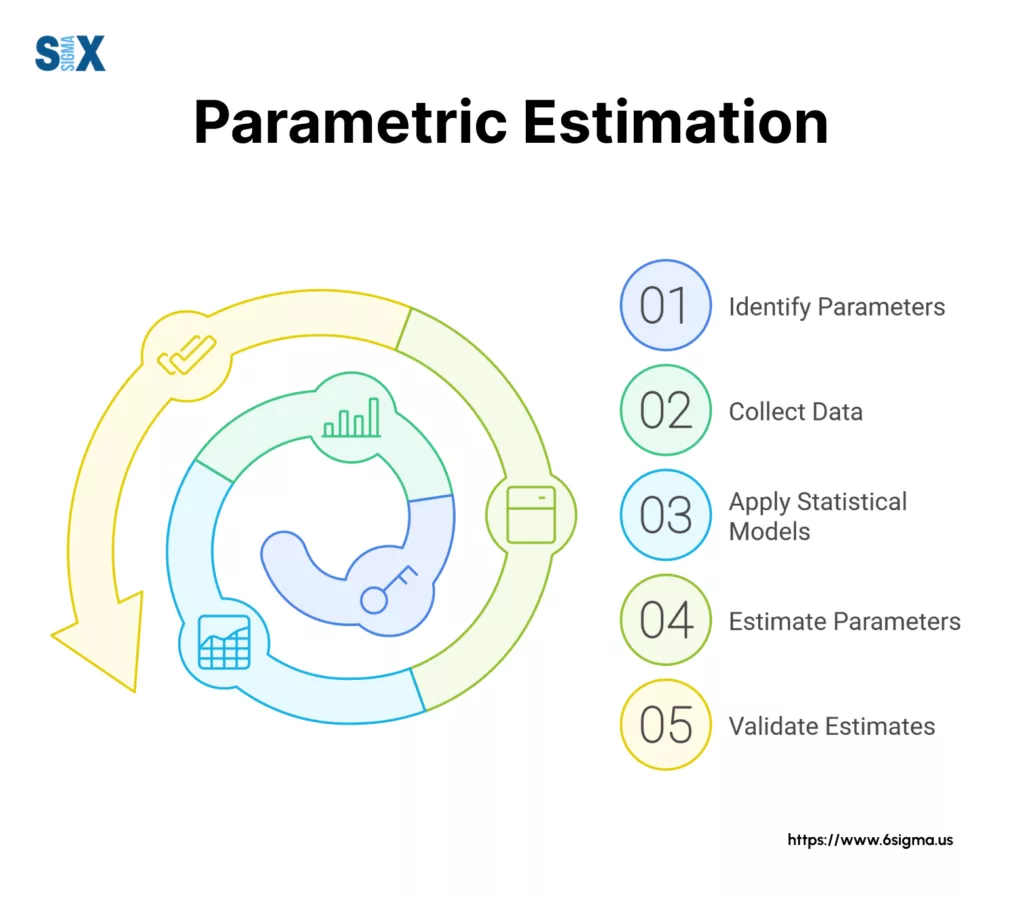
Essential Components Of The Parametric Estimation Process
The foundation of parametric estimation rests on three key elements: historical data, statistical relationships, and scaling factors.
Project teams gather data from past projects, analyze patterns between variables, and apply these relationships to new projects.
A manufacturing company might track the correlation between production volume and labor hours, then use this data to forecast staffing needs for larger orders.
Master the Define Phase and Project Selection with our comprehensive training program
How Parametric Estimation Differs From Other Estimation Methods
Unlike analogous estimating, which simply compares similar projects, parametric estimation breaks down projects into measurable units.
While expert judgment relies on experience, parametric methods use proven statistical correlations.
Bottom-up estimating examines individual tasks, but parametric estimation focuses on broader, measurable parameters that drive project outcomes.
Selecting The Right Time For Parametric Methods
Organizations benefit most from parametric estimation when they have:
- Reliable historical project data
- Measurable project parameters
- Similar types of recurring projects
- Need for scalable estimates
Manufacturing plants often employ parametric estimation because their projects share common characteristics yet vary in scale.
IT departments use this method to estimate software development efforts across different modules or features.
Construction firms apply parametric techniques when estimating costs for buildings with similar purposes but different sizes.
The success of parametric estimation depends on data quality and proper parameter selection.
The Parametric Estimation Process
Successful parametric estimation follows a structured approach that transforms historical project data into reliable forecasts.
Project managers who master this process gain a powerful tool for accurate project planning and resource allocation.
Gathering Quality Historical Data
The first step involves collecting detailed records from previous projects. Organizations should document key metrics such as actual costs, durations, resource usage, and project characteristics.
For instance, a software development team might track story points completed per sprint, while construction firms record labor hours per square foot of building space.
Project databases must maintain consistency in data collection methods.
Teams should standardize their reporting formats and ensure all relevant project parameters are captured. This standardization makes future analysis more reliable and helps identify patterns across different projects.
Selecting Relevant Project Parameters
Project parameters serve as the building blocks of estimation models.
These measurable characteristics directly influence project outcomes. Software projects might focus on function points or user stories, while manufacturing projects track production volumes and material quantities.
The key lies in choosing parameters that show strong correlations with project outcomes. Statistical analysis helps identify these relationships, revealing which factors most accurately predict costs and duration.
Building The Estimation Model
Once parameters are selected, teams develop mathematical models that express relationships between inputs and outputs.
These models range from simple equations to complex statistical frameworks. Construction estimators might use square footage multipliers, while IT projects employ function point analysis formulas.
Implementation On Current Projects
Applying the model requires careful measurement of current project parameters. Teams input these values into their estimation formulas to generate initial forecasts.
A manufacturing estimator might enter production quantities to calculate required labor hours and material costs.
Validation And Refinement
Model validation compares estimated values against actual results. Teams track estimation accuracy and identify areas for improvement.
Regular refinement keeps models aligned with changing business conditions and technological advances.
Modern estimation tools automate many aspects of this process. Software platforms can store historical data, perform statistical analysis, and generate estimates automatically.
These tools help teams maintain consistency while reducing the time required for calculations.
Regular model updates ensure continued accuracy as organizations evolve. Teams should review and adjust their estimation parameters as new technologies emerge or business practices change.
This ongoing refinement process helps maintain the reliability of parametric estimates over time.
Enhance your estimation skills with our Introduction to Graphical Analysis with Minitab course
Essential Formulas For Parametric Estimation
The foundation of parametric estimation lies in its mathematical approach to project forecasting.
Understanding these formulas enables project managers to generate accurate estimates consistently.
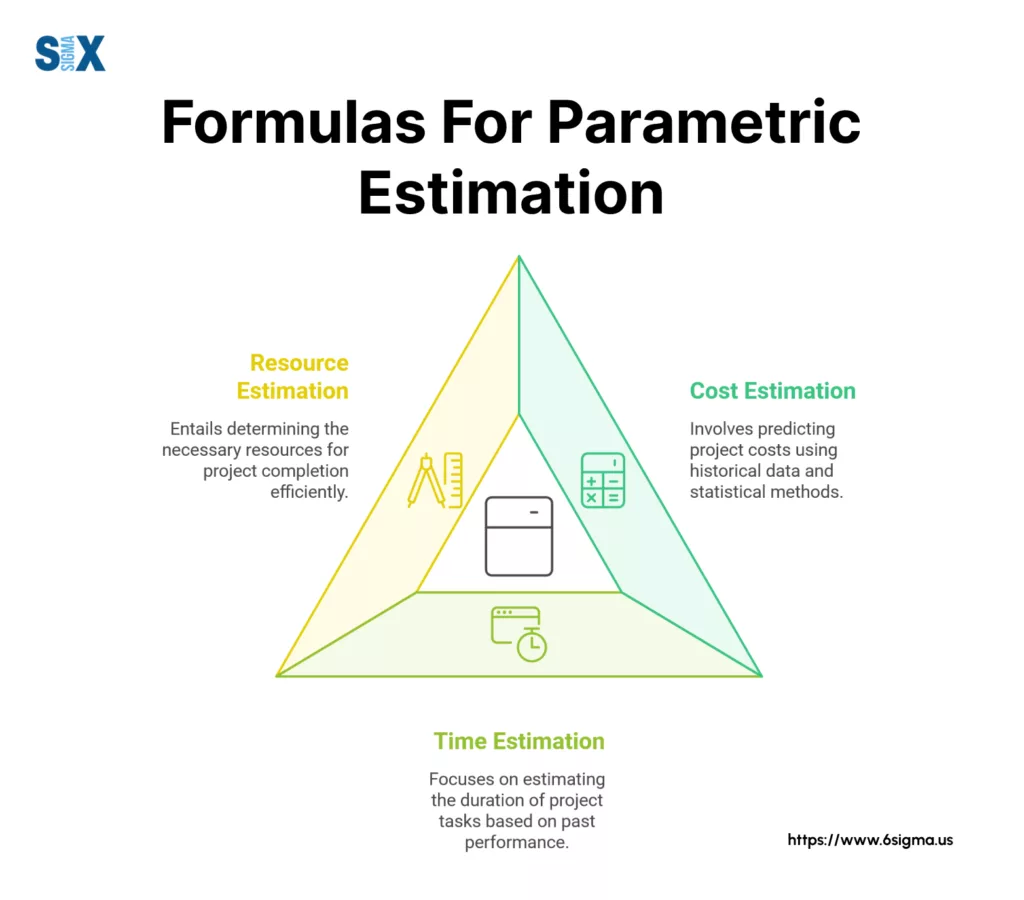
The Basic Formula
The standard parametric estimation formula follows this structure:
Estimate = (Historical Cost/Historical Parameter) × Current Parameter
For example, if a previous software development project required 100 hours to complete 20 function points, the formula would estimate 150 hours for a new project with 30 function points: (100/20) × 30 = 150 hours.
Industry-Specific Applications of the Parametric Estimation
Different industries adapt the basic formula to their specific needs. Construction projects often use multiple parameters:
Construction Cost = (Base Cost/Square Foot) × Total Square Footage × Complexity Factor
IT projects might incorporate additional variables:
Development Effort = (Historical Hours/Function Point) × Number of Function Points × Team Experience Factor
Manufacturing estimates frequently include production volumes:
Production Cost = (Historical Unit Cost × Production Volume) + Setup Cost
Ensuring Calculation Accuracy
Accurate calculations depend on several key factors. Project managers should normalize historical data to account for inflation and regional cost differences.
Regular validation of calculation results against actual project outcomes helps refine the formulas over time.
Statistical adjustments improve estimate reliability. Adding contingency factors based on risk analysis helps account for project uncertainties.
Teams should document their calculation methods and assumptions to maintain consistency across projects.
Modern estimation software streamlines these calculations while reducing human error. These tools can automatically adjust for various factors and provide confidence intervals for estimates.
However, project managers must still understand the underlying formulas to interpret results effectively.
Take your project management skills to the next level with Define Phase certification
Advanced Techniques In Parametric Estimation
Modern parametric estimation has evolved beyond basic calculations to incorporate sophisticated statistical methods.
These advanced techniques enable project managers to generate more accurate estimates and better understand project uncertainties.
Statistical Analysis For Better Accuracy in Parametric Estimation
Statistical analysis strengthens parametric estimation by revealing hidden patterns in project data.
Teams use correlation analysis to identify relationships between project variables and outcomes.
For example, construction firms analyze the correlation between building height and foundation costs to refine their estimates.
Variance analysis helps teams understand the reliability of their estimates.
By measuring the spread of historical data, project managers can determine confidence levels for their predictions. This information proves valuable when communicating estimate ranges to stakeholders.
Monte Carlo Simulation In Practice
Monte Carlo simulation takes parametric estimation further by modeling multiple project scenarios.
This technique runs thousands of calculations using different parameter combinations to show possible outcomes.
A software development team might simulate various team sizes and productivity rates to understand potential project durations.
The power of Monte Carlo simulation lies in its ability to account for uncertainty.
Rather than producing single-point estimates, it generates probability distributions that show the likelihood of different results.
Project managers use these distributions to make more informed decisions about schedules and budgets.
Regression Analysis For Complex Projects
Regression analysis helps teams handle projects with multiple interacting parameters.
Linear regression identifies how changes in one variable affect project outcomes, while multiple regression examines the combined impact of several variables.
Engineering firms often use regression analysis to estimate costs when dealing with new technologies or materials.
This technique creates mathematical models that predict project outcomes based on historical relationships.
Teams can test different scenarios by adjusting input variables, helping them understand how changes might affect their projects.
These advanced methods require proper data management and statistical expertise.
However, modern software tools make these techniques more accessible by automating complex calculations and providing user-friendly interfaces.
Project managers should focus on understanding the principles behind these methods to interpret results effectively and make sound decisions.
Essential Tools For Parametric Estimation
Modern parametric estimation software streamlines the estimation process while reducing calculation errors.
Leading tools like Oracle Primavera and Microsoft Project now include dedicated parametric estimation modules.
These platforms help teams manage historical data, perform calculations, and generate detailed reports.
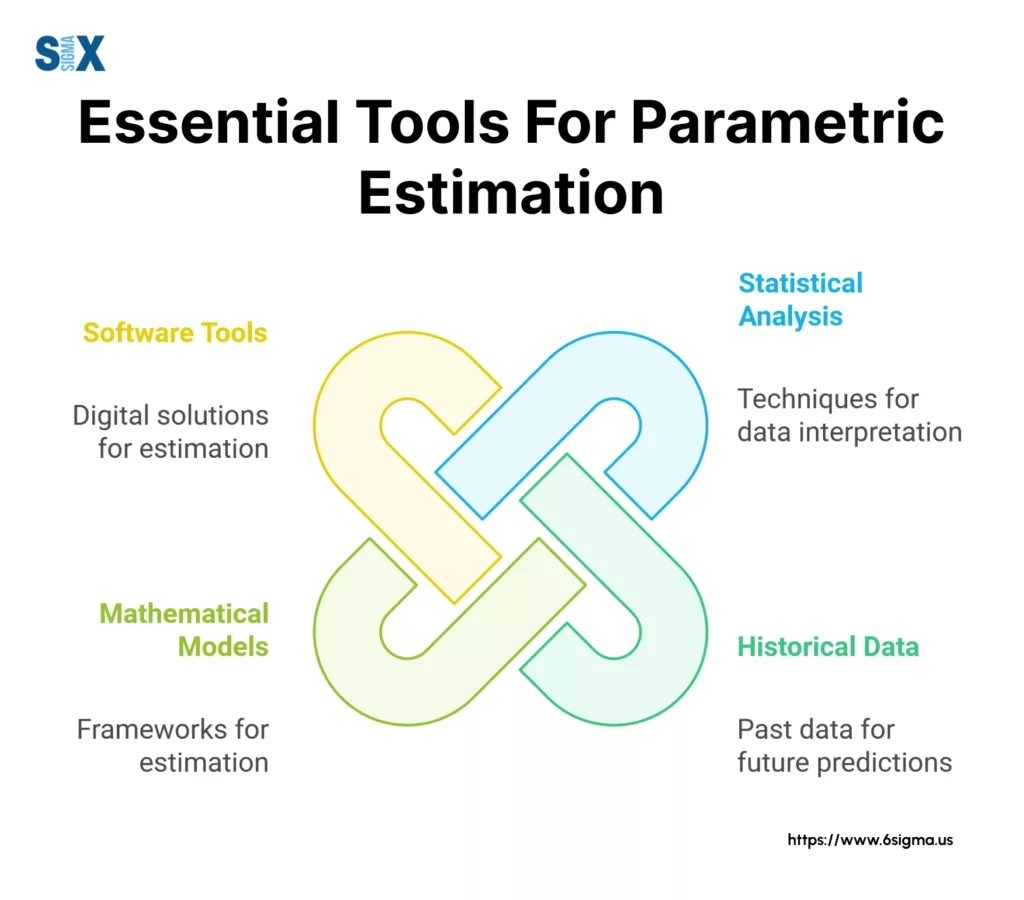
Key Features To Consider for Parametric Estimation
The most effective estimation tools offer database management for historical project data, statistical analysis capabilities, and customizable reporting options.
Advanced features include Monte Carlo simulation, regression analysis, and integration with project management systems.
Some tools also provide industry-specific templates and workflows tailored to construction, IT, or engineering projects.
Selecting The Right Software Solution
Organizations should evaluate software based on their specific needs, data volume, and technical capabilities.
Smaller teams might prefer cloud-based solutions with basic features, while large enterprises often need robust platforms with advanced analytics capabilities.
Avoiding Common Parametric Estimation Mistakes
Many organizations face challenges when implementing parametric estimation methods. Excessive reliance on historical data without considering current market conditions can lead to inaccurate estimates.
Teams must balance historical trends with present-day factors such as technological advances and changing market conditions.
Project-specific elements often get overlooked in the estimation process. Each project brings unique challenges and requirements that may not align perfectly with historical data.
Regular estimate updates throughout the project lifecycle help maintain accuracy as conditions change.
Moving Forward With Parametric Estimation
Parametric estimation continues to evolve as technology advances.
The integration of artificial intelligence and machine learning promises more accurate predictions and automated analysis.
Project managers who stay current with these developments position themselves for success in an increasingly data-driven field.
Success in parametric estimation requires ongoing commitment to data quality, regular model updates, and proper tool selection.
Organizations that invest in training and maintain robust estimation processes see improved project outcomes and more reliable forecasts.
SixSigma.us offers both Live Virtual classes as well as Online Self-Paced training. Most option includes access to the same great Master Black Belt instructors that teach our World Class in-person sessions. Sign-up today!
Virtual Classroom Training Programs Self-Paced Online Training Programs