OFAT (One-Factor-at-a-Time). All You Need to Know
Testing drives advancement in scientific investigation, item improvement, and cycle refining crosswise ventures. Traditionally, specialists utilized One Factor at a Time (OFAT) – fluctuating one variable while maintaining others steady.
Be that as it may, current convoluted innovations and advances undermine this methodology.
Often factors impact one another, and their unified impacts can’t be precisely caught by fluctuating variables independently. What’s more, OFAT consumes assets and occasions and outcomes stay deluding, particularly with non-direct connections or various cooperation factors.
To address these constraints, Design of Experiments (DOE) surfaces as an intense, statistically strong methodology. DOE strategies permit promptly investigating various factors, permitting primary impacts, cooperation impacts, and reaction variable streamlining assessment.
By joining regularization, replication, and hindering standards, DOE provides an organized, productive testing way of bringing progressively dependable and insightful outcomes.
Key Highlights
- OFAT (One Factor at a Time) involves varying variables independently and retaining others continuously.
- OFAT’s downsides number distinguishing collaboration impacts and potential deluding results.
- Design of Experiments (DOE) gives a well-ordered, statistically strong strategy empowering promptly researching numerous factors.
- Techniques (e.g. experimental plans, reaction surface procedures, shortlisting) offer advantages exceeding OFAT.
- DOE permits central impacts, cooperation impacts, and reaction factors streamlining examination through progressed factual investigations.
- Embracing DOE standards and procedures can prompt critical process comprehension, item advancement, and common viability enhancements.
What is OFAT (One Factor at a Time) Experimentation?
One Factor at a Time (OFAT) or classical/hold-one-factor-at-a-time strategies, specialists examine solitary factors’ impacts on maintaining others static.
Trials fluctuate one variable, sustaining others unbending at starting levels. After perception, switch back preceding varying the following.
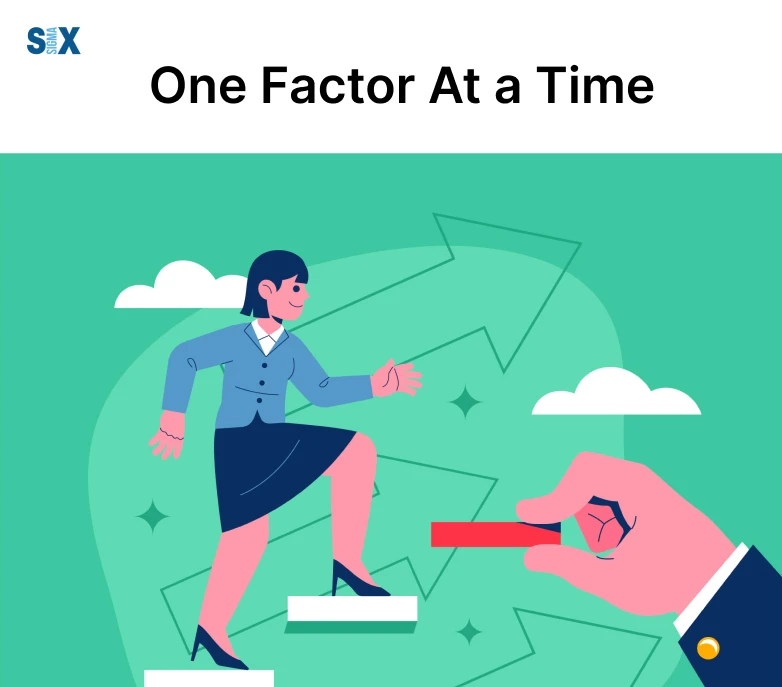
The approach involves:
- Selecting foundations
- Varying focuses independently while holding others static
- Observing reactions
- Circulating factors to beginnings preceding investigating others
This proceeds until all intrigued factors get tried independently. OFAT spares basic comprehensions amid restricted frameworks acquaintance.
Historical Background and Traditional Use of OFAT
The OFAT method has a long history and has been widely used in various fields, including chemistry, biology, engineering, and manufacturing. It was one of the earliest experimental strategies employed by researchers when studying complex systems with multiple factors.
The OFAT approach gained popularity due to its simplicity and ease of implementation. It allowed researchers to isolate the effect of individual factors without the need for complex experimental designs or advanced statistical analysis techniques. This made it a practical choice, especially in the early stages of scientific exploration or when resources were limited.
Traditionally, OFAT experiments were conducted manually, with researchers carefully controlling and adjusting factor levels one at a time.
This approach was instrumental in situations where experiments were time-consuming, expensive, or involved physical setups that required significant effort to modify multiple factors simultaneously.
Limitations and drawbacks of the OFAT approach
While the OFAT method has been widely used and has contributed to many scientific discoveries, it has several significant limitations and drawbacks:
- Failure to capture interaction effects: The OFAT approach assumes that factors do not interact with each other, which is often an unrealistic assumption in complex systems. By varying one factor at a time, it fails to account for potential interactions between factors, which can lead to misleading conclusions.
- Inefficient use of resources: OFAT experiments require a large number of experimental runs, especially when there are many factors involved. This can be time-consuming, costly, and may result in an inefficient use of resources.
- Lack of optimization capabilities: The OFAT method is primarily focused on understanding the individual effects of factors and does not provide a systematic approach for optimizing the response variable or identifying optimal factor combinations.
- Increased risk of experimental error: With a large number of experimental runs required, the risk of experimental error or uncontrolled variability increases, potentially affecting the reliability and reproducibility of the results.
- Limited scope: The OFAT approach is limited in its ability to explore the entire experimental region or factor space, as it only investigates factor levels along a single path or trajectory.
These limitations have led to the development of more advanced experimental design methodologies, such as Design of Experiments (DOE), which address the shortcomings of the OFAT approach and provide a more comprehensive and statistically sound framework for investigating complex systems.
Design of Experiments (DOE) as an Alternative to OFAT
Design of Experiments (DOE) is a systematic and structured approach to investigating the relationship between input factors and output responses.
Unlike the OFAT method, which varies one factor at a time while holding others constant, DOE allows for the simultaneous variation of multiple factors, enabling the study of their main effects and interactions.
DOE methodology is rooted in statistical principles and employs various experimental designs, such as factorial designs, response surface designs, and screening designs, among others.
These designs are carefully constructed to ensure efficient data collection and reliable analysis, minimizing experimental error and maximizing information gain.
Advantages of DOE over OFAT
DOE offers several significant advantages over the traditional OFAT approach:
- Ability to study interactions: DOE enables the investigation of interactions between factors, which are often overlooked in OFAT experiments. These interactions can have a profound impact on the response variable and are crucial for understanding complex systems.
- Improved efficiency: DOE experiments are designed to extract maximum information from a minimal number of experimental runs, resulting in significant time and cost savings compared to the OFAT method, which requires a larger number of runs to achieve similar results.
- Estimation of experimental error: DOE incorporates replication, allowing for the estimation of experimental error and the assessment of the statistical significance of the observed effects. OFAT experiments often lack this capability, leading to potential misinterpretation of results.
- Optimization capabilities: DOE, coupled with response surface methodology (RSM), provides a powerful tool for optimizing process or product parameters, enabling the identification of optimal operating conditions or formulations.
- Robustness and reliability: DOE experiments are designed to be robust against environmental and operational variations, ensuring that the results are reliable and reproducible.
Key principles of DOE (randomization, replication, blocking)
DOE is built upon three fundamental principles: randomization, replication, and blocking.
- Randomization: This principle ensures that the experimental runs are conducted in a random order, minimizing the impact of lurking variables and systematic biases. Randomization enhances the validity of the statistical analysis and the generalizability of the results.
- Replication: Replication involves repeating experimental runs under identical conditions to estimate the experimental error and improve the precision of the estimated effects. Replication is essential for assessing the statistical significance of the observed effects and increasing the reliability of the results.
- Blocking: Blocking is a technique used to account for known sources of variability, such as different operators, machines, or batches. By grouping experimental runs into homogeneous blocks, the impact of these nuisance factors can be isolated and removed from the experimental error, improving the precision of the estimated effects.
By adhering to these principles, DOE experiments provide robust and reliable results, enabling researchers and practitioners to make informed decisions based on sound statistical evidence.
Factorial Designs and Main Effects Analysis against OFAT
One of the key advantages of the Design of Experiments (DOE) over the OFAT approach is the ability to study multiple factors simultaneously using factorial designs. Unlike OFAT, where factors are varied one at a time, factorial designs allow for the investigation of the main effects of each factor as well as their interaction effects.
Factorial designs are constructed by combining all possible combinations of the levels of the factors under study. For example, in a two-factor experiment with two levels for each factor, a full factorial design would consist of four experimental runs (2^2 = 4).
As the number of factors and levels increases, the number of required experimental runs grows exponentially, providing a comprehensive understanding of the system’s behavior across the entire factor space.
Estimating main effects and interaction effects is a crucial aspect of factorial designs. Main effects represent the individual influence of each factor on the response variable, while interaction effects capture the combined effect of two or more factors on the response.
By analyzing these effects, researchers can identify the most significant factors and their interactions, enabling them to optimize the process or system under investigation.
Analysis of variance (ANOVA) is a statistical technique used for hypothesis testing in factorial designs. ANOVA partitions the total variability in the data into components associated with the main effects, interaction effects, and experimental error.
By comparing the mean squares of these components against the mean square of the error term, ANOVA determines the statistical significance of the effects.
The main effects and interaction effects are typically visualized using main effects plots and interaction plots, respectively. These graphical representations provide valuable insights into the nature and magnitude of the effects, aiding in the interpretation of the results.
One of the key advantages of factorial designs is their ability to estimate interaction effects, which are often overlooked or confounded with the main effects in the OFAT approach. Interaction effects can reveal synergistic or antagonistic relationships between factors, leading to a deeper understanding of the underlying process or system.
Furthermore, factorial designs offer improved precision in effect estimation compared to OFAT experiments. By considering all factor combinations simultaneously, factorial designs account for potential interactions and provide more accurate estimates of the main effects.
It is important to note that factorial designs require careful planning and consideration of the number of factors and levels to ensure a manageable number of experimental runs. In cases where the number of factors is large, fractional factorial designs or screening designs can be employed to reduce the experimental effort while still capturing the most significant effects.
Overall, factorial designs and the analysis of main effects and interaction effects through ANOVA provide a powerful and efficient approach to understanding complex systems and optimizing processes, overcoming the limitations of the OFAT method.
Response Surface Methodology (RSM) and Optimization
Response Surface Methodology (RSM) is a powerful statistical technique used in the design of experiments (DOE) for modeling and optimizing response variables.
It is particularly useful when the goal is to understand the relationship between multiple input factors and one or more response variables, as well as to identify the optimal settings of the input factors that maximize or minimize the response(s).
RSM for modeling and optimizing response variables
RSM involves fitting a mathematical model, typically a polynomial equation, to the experimental data obtained from a well-designed set of experiments. This model describes the behavior of the response variable(s) as a function of the input factors.
Once the model is fitted, it can be used to predict the response values for any combination of factor levels within the experimental region. Additionally, the model can be analyzed to locate the factor settings that optimize the response(s), either by maximizing, minimizing, or achieving a specific target value.
The key steps in RSM include:
- Designing the experiments using appropriate experimental designs (e.g., central composite designs, Box-Behnken designs).
- Conducting the experiments and collecting the response data.
- Fitting a mathematical model (e.g., quadratic model) to the data using regression analysis.
- Analyzing the model to identify significant factors, interactions, and curvature effects.
- Optimizing the response(s) by locating the factor settings that maximize, minimize, or achieve a desired target value.
- Validating the optimal solution through additional experimental runs.
Central composite designs and Box-Behnken designs
Central composite designs (CCDs) and Box-Behnken designs are two widely used experimental designs in RSM. These designs are specifically constructed to fit second-order (quadratic) models, which can capture curvature and interaction effects between factors.
Central composite designs consist of a factorial or fractional factorial design (to estimate main effects and interactions), axial points (to estimate quadratic effects), and center points (to estimate pure experimental error). CCDs are efficient designs that require fewer experimental runs than a full factorial design while still providing valuable information about the response surface.
Box-Behnken designs are another class of RSM designs that are particularly useful when the experimental region is limited or when certain factor combinations are impossible or impractical to run.
These designs do not include axial points, making them more economical in terms of the number of experimental runs required. Box-Behnken designs are also well-suited for situations where the factors have different units or scales.
Regression analysis and model fitting
In RSM, regression analysis is used to fit the mathematical model to the experimental data. The most common approach is to use least squares regression, which aims to minimize the sum of squared differences between the observed and predicted response values.
The choice of the regression model depends on the experimental design and the desired level of accuracy. For central composite designs and Box-Behnken designs, a second-order (quadratic) polynomial model is typically used:
y = β0 + Σ βi xi + Σ βii xi^2 + Σ Σ βij xi xj + ε
where y is the response variable, xi, and xj are the input factors, β0 is the constant term, βi are the linear coefficients, βii are the quadratic coefficients, βij are the interaction coefficients, and ε is the random error term.
Once the model is fitted, various diagnostic tools (e.g., residual analysis, lack-of-fit tests) are used to assess the model’s adequacy and ensure that the underlying assumptions of regression analysis are met. If the model is deemed satisfactory, it can be used for prediction, optimization, and further analysis.
By leveraging RSM techniques, researchers and practitioners can gain valuable insights into the relationships between input factors and response variables, identify optimal operating conditions, and ultimately improve process performance and product quality.
Screening Designs and Effect Sparsity Principles
When dealing with a large number of potential factors that may influence a process or product, it is often necessary to employ screening designs to identify the most significant factors before proceeding with further experimentation. Screening designs are a type of fractional factorial design that allows for the efficient investigation of many factors with a relatively small number of experimental runs.
Screening designs for identifying significant factors
Screening designs are particularly useful when there are many factors to consider, and it is impractical or too costly to run a full factorial experiment. These designs are constructed in such a way that they can estimate main effects while sacrificing the ability to estimate interaction effects. Common screening designs include Plackett-Burman designs, Fractional Factorial designs, and Definitive Screening Designs (DSDs).
The primary objective of screening designs is to identify the vital few factors that have a substantial impact on the response variable(s) of interest. This information can then be used to focus subsequent experimentation efforts on the most promising factors, saving time and resources.
Effect Sparsity and its Implications
Effect sparsity is a principle that suggests that in many real-world systems, only a relatively small number of factors significantly influence the response variable(s). This principle is based on the observation that most systems are dominated by a few key factors, while the remaining factors have negligible or no effect.
The concept of effect sparsity has important implications for experimental design and analysis. If effect sparsity holds, it means that screening designs can effectively identify the vital few factors without the need for a full factorial experiment, which would require a much larger number of experimental runs.
By leveraging effect sparsity, researchers can focus their efforts on the most influential factors, leading to more efficient experimentation and faster progress toward process understanding and optimization.
Fractional Factorial Designs and Resolution
Fractional factorial designs are a type of experimental design in which only a carefully chosen fraction of the complete factorial design is run. These designs are particularly useful when the number of factors is large, and running a full factorial design would require an impractically large number of experimental runs.
In fractional factorial designs, the main effects and lower-order interactions are estimated, but higher-order interactions are confounded or aliased with each other. The resolution of a fractional factorial design refers to the degree to which main effects and lower-order interactions are aliased with higher-order interactions.
For example, a resolution III design ensures that the main effects are not aliased with any two-factor interactions, but two-factor interactions may be aliased with each other. A resolution IV design guarantees that main effects are not aliased with two-factor interactions, but two-factor interactions may be aliased with each other and with higher-order interactions.
The choice of resolution depends on the experimental objectives and the assumptions made about the relative importance of different effect orders. Higher-resolution designs provide more detailed information but require more experimental runs.
By carefully selecting the appropriate screening design and leveraging effect sparsity principles, researchers can efficiently identify the most significant factors influencing their process or product, paving the way for further optimization efforts.
Comparison of OFAT and DOE results
Numerous studies have compared the results obtained from the traditional One Factor at a Time (OFAT) approach and the Design of Experiments (DOE) methodology. The findings consistently demonstrate the superiority of DOE over OFAT in terms of efficiency, accuracy, and insights gained.
- Efficiency: DOE allows for the simultaneous investigation of multiple factors, requiring fewer experimental runs compared to OFAT. This results in significant time and cost savings, especially in resource-intensive industries.
- Accuracy: DOE accounts for interactions between factors, which are often overlooked in OFAT. By considering these interactions, DOE provides a more accurate representation of the process or system under study, leading to better decision-making.
- Insights: DOE provides a comprehensive understanding of the process or system by quantifying the main effects and interactions of factors. This allows for the identification of critical factors and their optimal settings, enabling more effective process optimization and product development.
- Robustness: DOE incorporates principles like randomization, replication, and blocking, which enhance the robustness and reliability of the results. OFAT, on the other hand, is more susceptible to experimental errors and biases.
Numerous case studies have demonstrated that DOE often leads to superior solutions compared to OFAT, with improvements in product quality, process efficiency, and cost savings.
Parting Notes & Future Outlook of OFAT
As we conclude our exploration of OFAT (One Factor at a Time) experimentation and the powerful alternative of Design of Experiments (DOE), it’s important to emphasize the significance of adopting a structured and statistically sound approach to experimentation and process optimization.
The limitations of OFAT have been well-documented, and the advantages of DOE methodologies, such as factorial designs, response surface methodology, and screening designs, are evident.
By considering multiple factors simultaneously, accounting for interactions, and leveraging principles like randomization, replication, and blocking, DOE offers a more efficient and insightful approach to understanding complex systems and optimizing processes.
While the initial learning curve for DOE may seem steep, the long-term benefits of improved process understanding, reduced experimental effort, and increased confidence in results make it a worthwhile investment. Furthermore, with the availability of user-friendly software tools and expert guidance, implementing DOE has become more accessible than ever before.
As we look to the future, the integration of DOE with emerging technologies, such as artificial intelligence, machine learning, and advanced data analytics, holds immense potential. These synergies can further enhance the power of DOE by automating design generation, facilitating complex model building, and enabling real-time optimization in dynamic environments.
Additionally, the increasing emphasis on sustainability and environmental consciousness has led to a growing interest in incorporating sustainability considerations into experimental design and optimization efforts.
DOE methodologies can play a crucial role in developing more eco-friendly processes, products, and systems by efficiently identifying optimal operating conditions that minimize environmental impact while maintaining desired performance levels.
Ultimately, the adoption of DOE principles and the abandonment of the outdated OFAT approach will be crucial for organizations seeking to remain competitive in an ever-changing landscape.
By embracing a culture of data-driven experimentation and continuous improvement, companies can unlock new levels of innovation, efficiency, and success in their respective industries.
SixSigma.us offers both Live Virtual classes as well as Online Self-Paced training. Most option includes access to the same great Master Black Belt instructors that teach our World Class in-person sessions. Sign-up today!
Virtual Classroom Training Programs Self-Paced Online Training Programs