Normal Distribution in Lean Six Sigma. Learn the Bell Curve
Six Sigma is a quality management system that aims to decrease variance and errors in business processes.
The strategy makes use of statistical analysis and a data-driven methodology to reduce inefficiencies in the process and attain enduring outcomes.
Become a data-driven problem-solving expert
Master the Bell Curve, your gateway to process excellence with Lean Six Sigma Green Belt Certification.
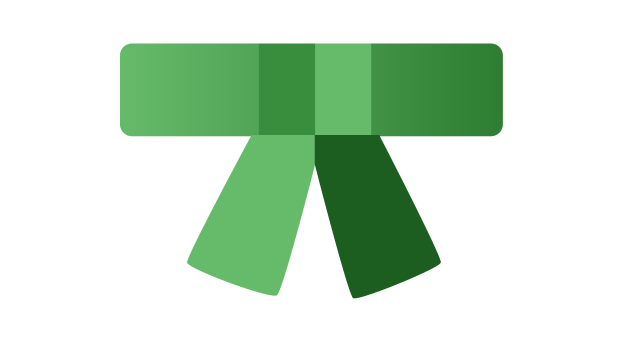
A key concept in Six Sigma is the normal distribution or bell curve.
The bell curve helps Six Sigma practitioners link process metrics to customer requirements. It visually depicts how variation affects process yield and capabilities.
By understanding specs and tolerances, processes can be optimized to deliver “perfect” output.
Key Highlights of Six Sigma Bell Curve
- The Six Sigma methodology relies heavily on statistical analysis and normal distribution. Mastering the bell curve is critical for effective implementation, which is a key focus of Six Sigma certification programs.
- While the bell curve sets challenging benchmarks, its principles serve as catalysts to drive process innovation and improvement across the organization.
- The journey towards Six Sigma levels of performance is gradual. However, each small gain compounds over time to achieve quantum leaps in quality.
- With robust data-driven approaches for defect reduction and variation control, the bell curve enables fact-based decision-making.
The Six Sigma approach
Achieving a defect level of 3.4 defects per million opportunities (DPMO) is the main objective of Six Sigma. Six Sigma programs use the five-phase improvement cycle of define, measure, analyze, improve, and control (DMAIC) to strive toward this aim.
Statistical methods and a range of quality management instruments are employed at every stage to comprehend process capability and pinpoint the underlying causes of errors.
Role of Normal Distribution / Six Sigma Bell Curve
The normal distribution, also known as the Six Sigma bell curve or Gaussian distribution, is a fundamental concept in Six Sigma. It describes how process metrics like cycle times, temperatures, pressures, concentrations, etc. are distributed in a stable process.
The bell curve plots the occurrence of different values, with the center representing the average value. The width of the bell indicates the amount of variation.
So the bell curve visually depicts both process center and spread. By fitting data to normal distributions, Six Sigma helps predict how often defects could occur at the tails.
This guides goal-setting for significant defect reduction. Understanding variance through the bell curve allows for improving process capability over time.
Learn the structured approach to reduce defects and optimize performance
Turn complex processes into streamlined success stories with Lean Six Sigma Green Belt Certification.
What is a Normal Distribution?
- Also called Gaussian distribution or bell curve
- A probability distribution that is symmetric about the mean
- Shows how data is distributed about the average
- Used to represent process performance data
- Helps determine process stability and capability
Properties and characteristics
- Bell-shaped symmetric curve
- Mean, median, and mode coincide in the center of the curve
- Area under curve equals 1 representing 100% probability
- Exact shape defined by mean and standard deviation
- 68% of data falls within 1 standard deviation of mean
- 95% within 2 standard deviations
- 99.7% within 3 standard deviations
Reason for bell shape
- Central limit theorem in statistics
- Means of random samples follow normal distribution
- Sample means cluster around the true population mean
- Variation in data leads to a bell curve shape
- The smaller the variation, the taller and skinner the bell
- The larger the variation, the shorter and wider the bell
Key Components of Normal Distribution
The normal distribution, also known as the Gaussian distribution, is characterized by some key components that define its shape and properties.
Understanding these components is essential for properly leveraging the normal distribution or bell curve in statistical analysis and quality improvement initiatives like Six Sigma.
Mean
The mean, commonly denoted by the Greek letter mu (μ), defines the central tendency of the normal distribution. It indicates the location of the peak and can be thought of as the “center” of the distribution.
The mean is calculated by taking the sum of all observations and dividing it by the total number of observations.
In the context of Six Sigma, the mean represents the average value or central tendency of a process.
Standard Deviation
The standard deviation, denoted by the Greek letter sigma (σ), defines the amount of variation present in the normal distribution. It is a measure of how dispersed the observations are about the mean.
A small standard deviation indicates that the data points are clustered closely around the mean, while a large standard deviation indicates the data is more spread out.
The standard deviation is used to calculate the sigma levels in Six Sigma.
Sigma Levels
In Six Sigma, sigma levels indicate how much variation exists in a process relative to customer requirements or specifications.
The higher the sigma level, the less variation and defects there are. Industry benchmark sigma levels include:
- Six Sigma = 3.4 defects per million opportunities
- Five Sigma = 233 defects per million opportunities
- Four Sigma = 6,210 defects per million opportunities
- Three Sigma = 66,807 defects per million opportunities
By understanding the key components of mean, standard deviation, and sigma levels, we can properly utilize the normal distribution to analyze process performance and guide improvement initiatives.
Statistical process control techniques leverage these metrics to reduce variation and drive toward Six Sigma quality levels.
Using Six Sigma Bell Curve
The normal distribution curve, or bell curve, is a key tool used in the 6 Sigma methodology to analyze process performance against stringent quality standards.
By linking process metrics to the bell curve, we can define quantifiable capability metrics for the process.
Linking Six Sigma Bell Curve to Process Performance
The bell curve allows us to model process performance and visualize how output values are distributed.
The curve represents the frequency of occurrence of different output values from a process. By fitting process data to the bell curve model, we can define metrics like process mean and standard deviation.
This builds the foundation for advanced analysis.
Most processes will have a distribution of output values that approximates a normal distribution or bell curve, due to underlying statistical principles.
The output values may represent characteristics like product dimensions, material strength, purity levels, defects, errors, etc.
By relating process data to bell curve models we can measure central tendency and dispersion.
Defining Process Capability Metrics with Six Sigma Bell Curve
Process capability quantifies how well a process meets specifications. Key metrics are derived from the normal distribution – process capability indices Cp and Cpk. A Cp or Cpk greater than 1.33 is desirable.
At 6 sigma quality, it corresponds to 3.4 defects per million opportunities.
The ‘sigma levels’ marked on the x-axis of the curve denote how much spread of values is present relative to the mean. So +3 sigma means range from mean to upper specification limit contains 99.7% of values. Higher sigma levels indicate lower variation and greater stability.
The goal of 3.4 Defects Per Million Opportunities
The 6 Sigma methodology targets processes operating with no more than 3.4 defects per million opportunities. This corresponds to 6 sigma quality or 99.99966% good quality output.
To achieve this, processes must show capability indices higher than 2.
Certified Six Sigma professionals, such as those with Green Belt certification and Black Belt certification, are equipped with the skills to lead such improvement projects.
They should exhibit stability with minimal variation around the mean. By using normal distribution models and tracking metrics over time, processes can be continuously improved.
Performing Statistical Analysis
Statistical analysis is a critical component of Six Sigma methodologies. By collecting and analyzing process data, variations, and stability issues can be identified to drive targeted improvements.
Understanding Variation – Stable vs Unstable Processes
A key concept within Six Sigma is understanding variation in process performance. Variation occurs naturally in all processes and the goal is to control and reduce variation rather than eliminate it.
Statistical analysis defines the difference between stable and unstable process variation.
Stable Process Variation: This is normal or expected variation that exists in a state of statistical control.
The high and low data points fall within the expected distribution and predictability is possible.
Unstable Process Variation: This is an abnormal or unexpected variation where patterns show the process going out of control. The data shows unpredictable swings and trends rather than a balanced distribution. Unstable processes have excess variation.
Using Statistical Process Control (SPC)
SPC techniques are utilized in Six Sigma to track process metrics over time and distinguish between stable and unstable variation. Control charts are the most common SPC tool, skills that are extensively covered in Six Sigma Black Belt certification programs.
Control limits are defined to identify data points falling outside the norm so assignable causes can be investigated. This provides objective guidance for determining process stability.
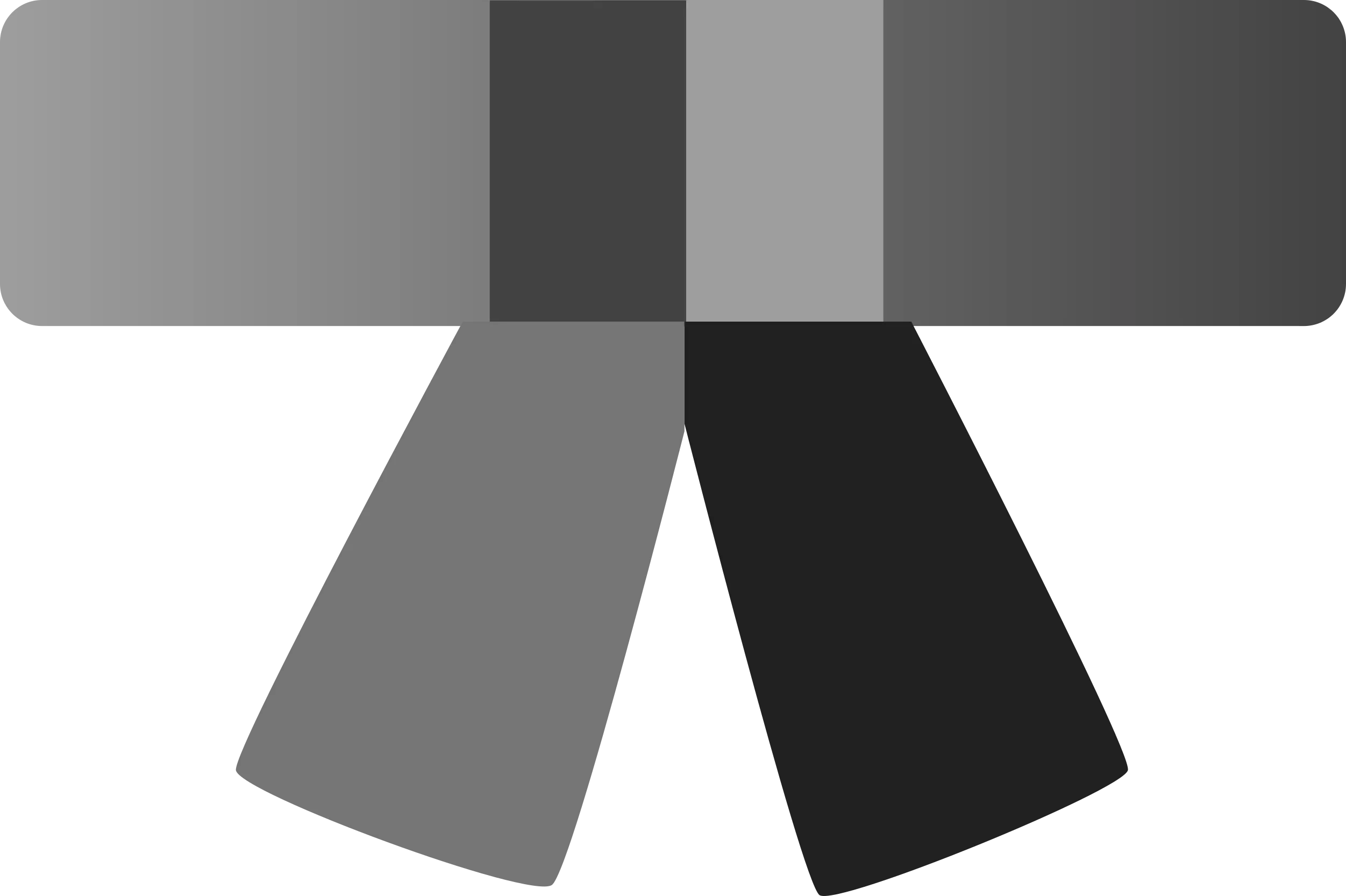
Dive deep into statistical process control and root cause analysis
Become the go-to expert for process stability and improvement with Lean Six Sigma Black Belt Certification.
Tracking Process Metrics Over Time
Tracking key process metrics is essential within the DMAIC methodology. Critical to Quality metrics that impact customer requirements are defined and monitored to identify improvement opportunities.
This includes defects, yield, capability, accuracy, time, cost, and other parameters. Control charts demonstrate capability and variation reduction achieved through Six Sigma projects and initiatives. This allows for ongoing, sustainable process control.
6 Sigma Bell Curve – Driving Process Improvements
A critical goal of 6 Sigma is to drive significant improvements in key processes to reduce defects, lower costs, increase customer satisfaction, and achieve sustainable competitive advantage.
This requires a strong focus on understanding process performance deeply using statistical analysis, and taking data-driven actions to enhance process capability.
Reducing Defects and Variation with 6 Sigma Bell Curve
The bell curve helps set precise targets for reducing defects and variation in a process. As process performance shifts towards the right under the bell curve, it indicates that the mean is moving towards specification limits while variation is decreasing at the same time.
The goal is to achieve performance at the 6 Sigma level, which translates to 3.4 defects per million opportunities. This is achieved through an array of process improvement and optimization initiatives.
Some examples include error-proofing processes to prevent defects, setting up visual controls and standard operating procedures to reduce variation, automation to minimize human errors, and continuous process monitoring to detect issues early.
Statistical process control charts are invaluable for studying trends in key process metrics and identifying special causes vs common causes of variation.
This aids root cause analysis and helps control processes better.
Improving Process Capability
Process capability quantifies the performance of a process versus its specification limits. Metrics like Cp and Cpk capture process variation and indicate whether the process meets customer requirements.
As process inputs and other parameters are optimized, capability improves. This is reflected in metrics like reduced defects, faster speeds, improved first-pass yield, and greater consistency of outputs.
For example, in manufacturing processes, key inputs like temperature, pressure, flow rates, voltages, speeds, etc. are controlled more tightly to influence mean and variability.
In transactional processes, steps are eliminated or automated to minimize manual errors. These examples enhance process capability by shifting the mean towards the target and reducing variation.
Achieving Process Excellence
While operational excellence focuses on individual processes, process excellence takes a systemic view to connect processes enterprise-wide and optimize the whole value chain.
It aligns people, processes, tools, and metrics seamlessly towards strategic business objectives.
Some hallmarks of process excellence include cross-functional collaboration, data-driven culture, automation with real-time controls, and continuous improvement culture across all layers.
As organizations mature in their process excellence journey, they reorient structure, leadership focus, individual KPIs, and competencies to create customer-centric processes.
With 6 Sigma driving rapid improvements across critical processes, they can exceed customer expectations consistently. This earns them loyalty, growth, and competitive differentiation in their marketplace.
Applications and Examples of Six Sigma Bell Curve
The normal distribution and bell curve have widespread applications across various industries and domains.
Understanding how the bell curve links to process performance metrics allows organizations to statistically analyze their processes and drive impactful improvements.
Manufacturing Processes
The normal distribution is extremely useful in manufacturing for quality control and process capability analysis.
By collecting process data and plotting metrics on the bell curve, manufacturers can identify production defects, assess process stability, capability, and consistency.
This allows them to find root causes of issues, reduce variability, and improve yields.
Some Examples of Six Sigma Bell Curve include:
- Monitoring the diameter size of bearings produced and reducing variability to meet specifications
- Tracking defects per unit and improving processes to reach 6 Sigma capability
- Analyzing equipment precision and accuracy to improve process centering and predictability
Business Processes
Service and transactional business processes can also leverage normal distribution and statistical analysis. Examples include:
- Measuring call center response times and improving customer service levels
- Reducing order processing defects to improve order accuracy metrics
- Analyzing employee turnover rates and improving talent retention
Healthcare Processes
Healthcare providers use normal distribution and 6 sigma to control clinical and administrative processes. For example:
- Tracking surgical complications data to minimize patient risk
- Improving hand hygiene compliance to reduce hospital infections
- Reducing medical claims processing errors to improve accuracy and efficiency
The bell curve provides a statistical foundation to measure process quality, analyze variation, and drive process excellence across diverse sectors and processes.
Organizations across manufacturing, business services, and healthcare have applied its concepts to achieve process capability and improvements.
Parting Notes
The Six Sigma bell curve and normal distribution play a pivotal role in the Six Sigma methodology for process improvement. By leveraging statistical analysis, the bell curve helps set process performance benchmarks and capability targets.
The bell curve allows the calculation of vital Six Sigma metrics like process capability index, defects per million opportunities, sigma levels, etc. Based on these metrics, the process performance is quantified.
Tracking metrics over time and performing statistical process control reveals whether a process is stable and predictable. Variation reduction is a core focus area.
As process metrics reach world-class levels, the rightward shift of the bell curve denotes significant defect reduction and improvements in quality.
The bell curve provides actionable insights to further optimize processes, reduce waste, increase efficiency, and achieve operational excellence.
SixSigma.us offers both Live Virtual classes as well as Online Self-Paced training. Most option includes access to the same great Master Black Belt instructors that teach our World Class in-person sessions. Sign-up today!
Virtual Classroom Training Programs Self-Paced Online Training Programs