Multivariate SPC: Advanced Process Monitoring & Control Techniques
Quality observation methods sometimes fall short addressing intricate links and interdependencies between numerous workflow aspects. Multivariate SPC addresses this.
Multivariate monitoring addresses intricate data realities, correlations, and nonlinear motions, cultivating deeper comprehension and management within complex manufacturing environments.
Key Highlights
- Multivariate SPC (MSPC) is a collection of advanced techniques for monitoring and controlling batch
- Multivariate SPC (MSPC) reduces the dimensionality of process data, capturing the most relevant information
- Key Multivariate SPC (MSPC) techniques
- Multivariate SPC (MSPC) enables effective fault detection, diagnosis, and quality control
- Ongoing research focuses on handling non-linearity and non-Gaussianity and integrating process knowledge with hybrid modeling approaches.
Understanding Multivariate Quality Observation
Multivariate Statistical Process Control (MSPC) refers to a set of advanced techniques used for monitoring and controlling the performance of complex industrial processes involving multiple interrelated variables.
MSPC methods analyze the relationships and correlations among process variables, enabling effective fault detection, diagnosis, and quality control in high-dimensional data environments.
Traditional quality methods concentrating singularly prove limited amid correlations within modern coordination.
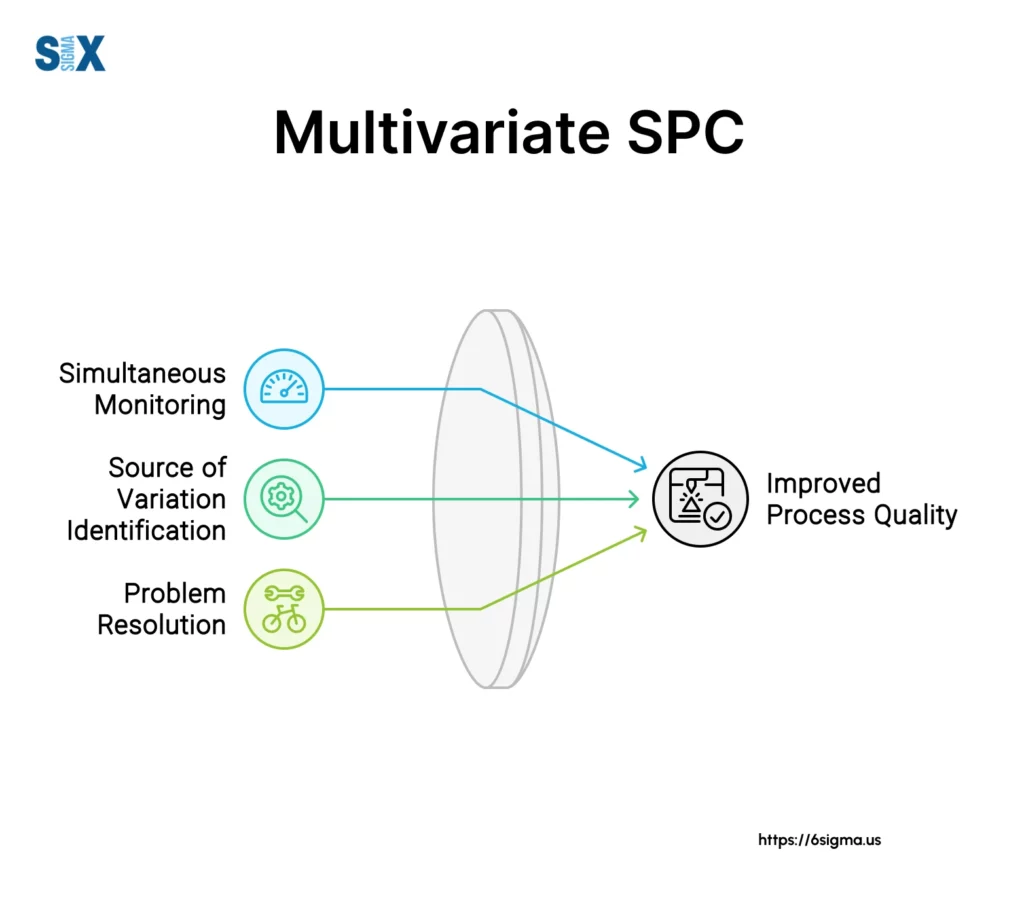
This realization birthed Multivariate Quality Observation – a versatile set of advanced techniques for lucidly tracking and coordinating batch/uninterrupted workflows’ performances.
For prudent caretakers committed to performance and relationships safeguarded through challenges jointly overcome, Multivariate Quality Observation introduces promising gifts. Progress springs from insights navigating complexity’s dances for reliable discoveries.
Importance of Multivariate SPC in Batch Processes
Batch processes are prevalent in various industries, including chemicals, pharmaceuticals, food production, textiles, metals, and semiconductors.
In these processes, raw materials or reactants are charged into a vessel or reactor and subjected to a series of controlled conditions over a defined period, resulting in the desired end product.
Effective monitoring and control of batch processes are crucial for ensuring product quality, consistency, and compliance with regulatory standards.
Multivariate SPC techniques are particularly well-suited for batch processes because they can handle the vast amount of data generated during a single batch run, which often involves hundreds of measurements for dozens of process variables.
By collectively analyzing these interdependent variables, Multivariate SPC (MSPC) methods can extract meaningful information, identify potential issues, and provide a framework for continuous process improvement.
Challenges in Traditional SPC Methods
While traditional univariate SPC methods have proven valuable in many applications, they face significant limitations when applied to modern batch processes.
One major challenge is the inability to effectively monitor and interpret the relationships among multiple correlated process variables.
By treating each variable independently, crucial information about the underlying process dynamics may be overlooked.
Furthermore, as the number of process variables increases, the complexity of monitoring individual control charts becomes increasingly cumbersome and prone to errors.
Traditional SPC methods also struggle to detect small or moderate shifts in the process mean, which can be masked by noise or correlations among variables, leading to delayed fault detection or missed opportunities for corrective action.
Another limitation arises from the assumption of independence and multiformity, which may not hold for many real-world processes.
Violating these assumptions can lead to inaccurate control limits and unreliable monitoring, compromising the effectiveness of the SPC system.
Dimensionality Reduction Techniques in Multivariate SPC
To address the challenges posed by high-dimensional and correlated process data, Multivariate SPC (MSPC) employs a range of dimensionality reduction techniques.
These methods aim to extract the most relevant information from the original data while reducing its complexity, enabling effective monitoring and interpretation.
By projecting the data onto a lower-dimensional subspace, Multivariate SPC (MSPC) methods can reveal underlying patterns, identify deviations from normal operating conditions, and facilitate fault diagnosis.
Multiway Principal Component Analysis (MPCA)
One of the foundational techniques in Multivariate SPC (MSPC) is Multiway Principal Component Analysis (MPCA).
Developed by Nomikos and MacGregor in the 1990s, MPCA extends the widely used Principal Component Analysis (PCA) method to handle three-way arrays of batch process data.
By unfolding the three-dimensional array into a two-dimensional matrix, MPCA can extract principal components that capture the most significant variations in the data.
The MPCA model is typically built using historical data from normal batch runs, serving as a reference for monitoring future batches.
Key statistics, such as Hotelling’s T-squared and the Squared Prediction Error (SPE), are calculated and plotted on control charts, enabling the detection of deviations from normal operating conditions.
MPCA offers several advantages, including dimensionality reduction, noise filtering, and the ability to visualize batch trajectories.
However, it relies on the assumption of linearity and may struggle with non-linear or non-Gaussian data structures.
Multiway Partial Least Squares (MPLS) within Multivariate SPC
Complementing MPCA, Multiway Partial Least Squares (MPLS) is another powerful dimensionality reduction technique in Multivariate SPC (MSPC). Like its counterpart, MPLS operates on unfolded three-way arrays of batch process data.
However, unlike MPCA, which focuses solely on maximizing the explained variance in the predictor variables, MPLS also considers the relationship between predictor and response variables.
In batch processes, MPLS can be employed to predict final product quality measurements or other critical response variables based on the trajectories of process variables during the batch run.
This predictive capability makes MPLS particularly valuable for online monitoring and quality control, as it enables proactive adjustments to ensure desired product specifications are met.
MPLS models are constructed using historical data, with the process variables serving as predictors and the quality variables as responses.
By maximizing the covariance between these two sets of variables, MPLS identifies latent structures that capture the most relevant information for prediction.
Structuration à Trois Indices de la Statistique (STATIS)
Structuration à Trois Indices de la Statistique (STATIS) is a multivariate data analysis method developed by L’Hermier des Plantes and later extended by Escoufier and others.
It is particularly useful for exploring and analyzing the structure of multiple data tables obtained under different scenarios, such as batch processes with varying durations.
STATIS operates on three-way arrays of batch data by performing a series of interstructure and intrastructure analyses.
The interstructure step identifies similarities and differences among the individual batches, while the intrastructure step constructs a common structure that represents the overall data set.
This approach allows for effective visualization and interpretation of batch trajectories while preserving the multiway nature of the data.
One of the key advantages of STATIS is its ability to handle batches of variable durations without the need for complex preprocessing steps.
Additionally, the method provides valuable insights into the relationships among process variables and their contributions to batch-to-batch variations.
Parallel Factors Analysis (PARAFAC/CANDECOMP) within Multivariate SPC
Parallel Factors Analysis (PARAFAC), also known as Canonical Decomposition (CANDECOMP), is a powerful dimensionality reduction technique for three-way arrays.
Developed independently by Harshman Carroll and Chang in the 1970s, PARAFAC decomposes a three-way array into a sum of rank-one tensors, each representing a trilinear component.
In the context of batch processes, PARAFAC can be applied directly to the three-way array of process data, without the need for unfolding.
The decomposition yields a set of score vectors and loading matrices that capture the underlying patterns and relationships among batches, variables, and time points.
Tucker3
Introduced by Ledyard R. Tucker in 1966, Tucker3 is a generalization of the PARAFAC model that allows for greater flexibility in capturing complex data structures.
While PARAFAC decomposes a three-way array into a sum of rank-one tensors, Tucker3 incorporates an additional core array that captures potential interactions among the components.
In the context of batch processes, Tucker3 decomposes the three-way array of process data into a core array and three loading matrices, one for each mode (batches, variables, and time points).
This decomposition enables the extraction of meaningful patterns and relationships while accounting for potential non-trilinear structures in the data.
Tucker3 offers several advantages over PARAFAC, including the ability to handle more complex data structures and the flexibility to choose different numbers of components for each mode.
However, this increased flexibility comes at the cost of more challenging interpretation and the potential for non-unique solutions.
When applied to batch process data, Tucker3 can provide valuable insights into the underlying factors driving process variability, facilitate fault detection and diagnosis, and support process optimization efforts.
Multiset Canonical Correlation Analysis (MCCA)
Multiset Canonical Correlation Analysis (MCCA) is a powerful technique that extends the traditional Canonical Correlation Analysis (CCA) to handle multiple sets of variables simultaneously.
In the context of batch processes, MCCA can be employed to analyze the relationships among different sets of process variables or between process variables and quality variables.
Unlike CCA, which is limited to two sets of variables, MCCA can identify canonical variables that maximize the correlations among multiple sets.
This capability makes MCCA particularly useful for monitoring parallel-running batch processes or processes with multiple stages or units.
Non-Dimensionality Reduction Techniques
While dimensionality reduction techniques are widely used in Multivariate SPC (MSPC), there are situations where preserving the original dimensionality of the data may be advantageous.
Non-dimensionality reduction techniques aim to extract meaningful information from the high-dimensional process data without sacrificing its inherent complexity.
These methods can provide complementary insights and serve as valuable alternatives or supplements to dimensionality reduction approaches.
Multiway Independent Component Analysis (MICA)
Multiway Independent Component Analysis (MICA) is a non-dimensionality reduction technique that extends the widely used Independent Component Analysis (ICA) method to handle three-way arrays of batch process data.
Unlike MPCA, which assumes Gaussian distributions and linear relationships, MICA is particularly effective when dealing with non-Gaussian and non-linear data structures
MICA operates by decomposing the three-way array into a set of independent components that are statistically independent from one another.
This decomposition is achieved by maximizing a non-Gaussianity measure, such as kurtosis, and iteratively extracting components that are decorrelated from the previously extracted ones.
By capturing non-Gaussian and non-linear patterns in the data, MICA can provide valuable insights into the underlying process dynamics and facilitate the identification of complex fault conditions.
While MICA can be computationally intensive, particularly for large datasets, its ability to handle non-Gaussian and non-linear data structures makes it a valuable addition to the Multivariate SPC (MSPC) toolkit, complementing dimensionality reduction techniques like MPCA and MPLS.
Multiway Slow Features Analysis (MSFA) in contrast with Multivariate SPC
Multiway Slow Features Analysis (MSFA) is a non-dimensionality reduction technique inspired by the Slow Features Analysis (SFA) method.
SFA aims to find a set of instantaneous scalar input-output mapping functions that vary as slowly as possible over time, capturing the underlying dynamics of the process.
In the context of batch processes, MSFA operates on the unfolded three-way array of process data, extracting slow features that represent the most slowly varying patterns in the data.
These slow features can provide valuable insights into the long-term trends and underlying dynamics of the batch process, facilitating effective monitoring and fault detection.
Parallel Coordinates
Parallel Coordinates is a powerful visualization technique that allows for the simultaneous representation of high-dimensional data in a two-dimensional space.
In the context of Multivariate SPC (MSPC), Parallel Coordinates can be used to visualize and analyze the trajectories of process variables across multiple batches, without the need for dimensionality reduction.
While Parallel Coordinates can be challenging to interpret for very high-dimensional datasets, it remains a valuable tool for visualizing and understanding batch process data, complementing other dimensionality reduction and non-dimensionality reduction techniques in the MSPC toolbox.
Monitoring and Control Charts in Multivariate SPC
At the heart of any Statistical Process Control (SPC) system lies the concept of control charts, which provide a visual representation of the process behavior and enable the detection of deviations from normal operating conditions.
Hotelling’s T-squared and Squared Prediction Error (SPE)
Two of the most widely used control charts in Multivariate SPC (MSPC) are Hotelling’s T-squared (T²) and the Squared Prediction Error (SPE), also known as the Q statistic.
These charts are typically used in conjunction with dimensionality reduction techniques like MPCA and MPLS.
Hotelling’s T² statistic measures the variation in the score space, representing the overall deviation of a batch from the normal operating conditions as defined by the reference model.
By plotting T² values on a control chart, significant deviations can be detected, indicating potential issues or abnormalities in the batch process.
Fault Detection and Diagnosis in Multivariate SPC
One of the primary objectives of Multivariate SPC (MSPC) is to enable effective fault detection and diagnosis, which is crucial for maintaining process stability, product quality, and overall operational efficiency.
Control charts, such as Hotelling’s T² and SPE, serve as the frontline for detecting deviations from normal operating conditions, triggering further investigation and corrective actions.
However, simply detecting a fault is often insufficient; identifying the root cause and diagnosing the specific variables or process stages responsible for the deviation is equally important.
Semiconductor Manufacturing Processes
The semiconductor industry, with its highly complex and tightly controlled manufacturing processes, has also embraced the power of Multivariate Statistical Process Control (MSPC).
In this industry, even minor deviations from optimal conditions can result in costly product defects or yield losses, making effective process monitoring and control paramount.
Quality Control and Process Optimization in Multivariate SPC
While MSPC techniques are invaluable for monitoring and fault detection, their applications extend beyond these core functions.
By providing a comprehensive understanding of the interactions among process variables and their impact on product quality, Multivariate Statistical Process Control (MSPC) can play a crucial role in quality control and process optimization initiatives.
Future Directions
While Multivariate Quality Observation gifted multidisciplinary partnerships greatly, plenty remain imperfect and opportunities ripe.
As coordination evolves intricately, its techniques must adapt resourcefully to modern coordination and oversight demands.
While patterns emerge clearly from insights, effectiveness grows through domain-specific understanding integration and hybrid modeling.
Engineers and specialists offer crucial comprehension of physics, chemistry, and interactions to enhance interpretation for reliable discoveries.
Weaving such understanding within its guidance – relationships, principles, or constraints recognized – models acuity multiplies. This supports prudent challenge-finding, root discovery, and advancements wherever performance impacts meaningful relationships.
Progress proves unending as comprehension and cooperation evolve jointly. May share insights through challenges overcome sustain all journeying together wherever quality and safety matter profoundly.
SixSigma.us offers both Live Virtual classes as well as Online Self-Paced training. Most option includes access to the same great Master Black Belt instructors that teach our World Class in-person sessions. Sign-up today!
Virtual Classroom Training Programs Self-Paced Online Training Programs