What are Lurking Variables? Definition, Examples, and More
Within analytic and measurable examination, concealing factors stay a hushed yet compelling power decidedly affecting considers legitimacy and translations.
These imperceptible variables, regularly disregarded or unconsidered, can contort genuine relations between considered fixings, bringing about predisposed results and misleading finish.
Confounding variables, additionally called lurking variables, influence both free and subordinate variables being broken down yet stay unequivocally estimated or controlled.
Impacts incorporate extending, debilitating or inverting affiliations, or making false associates. Failing location and administration undermines exactness and trustworthiness over numerous fields.
Key Highlights
- Concealing variables depict unacknowledged impacts distorting examined relations and bringing about predisposed, deluding results.
- They may diminish, reinforce, or even invert watched affiliations, making bogus associates or concealing certifiable impacts.
- Neglecting imperceptible variables and dangerous outcomes like imperfect basic leadership, deficient arrangements, and conceivably dangerous outcomes.
- Distinguishing and overseeing confounding variables is fundamental for ensuring inquiry about exactness and believability.
- Techniques incorporate attentive investigation arrangement, factual techniques like relapse investigation, haphazard experiments, machine learning, and causal deduction structures.
Understanding Lurking Variable: Why They Matter in Research
A concealing variable depicts an unconsidered factor affecting connections between concentrated pivots.
Not incorporating it dangers avoided variable predisposition, wrongly assigning impacts to considered fixes, and distorting outcomes.
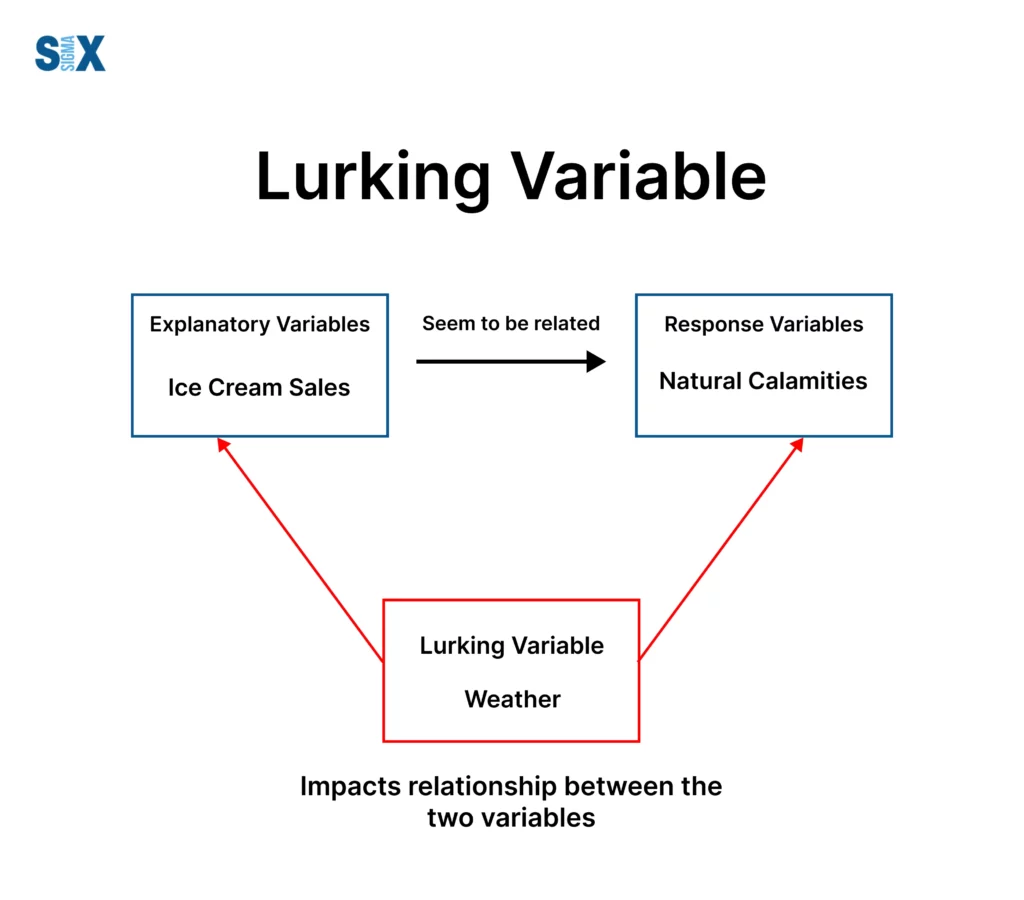
For illustration, a spurious connection happened between ice cream deals and drownings. Warm climate drives both, making this third lurking variable.
Perceptible relationships might fortify, diminish, or even invert. Phantom connects emerge or certifiable impacts mask.
To illustrate this concept, let’s consider an example of a spurious correlation ā a seemingly meaningful relationship between two variables that is caused by a third, lurking variable.
The impact of lurking variables on statistical analysis and interpretation cannot be overstated. These hidden factors can magnify or weaken the observed relationships, change the direction of correlations, or even create phantom associations where none truly exist.
Examples of Lurking Variables in Action
To further illustrate the concept of lurking variables and their potential impact, let’s explore some examples from various disciplines.
- Coffee and heart disease: Researchers conducted a study to determine whether there is a relationship between drinking coffee and heart disease. The study found a positive relationship, suggesting that coffee consumption may increase the risk of heart disease.
However, upon closer examination, it was discovered that the participants who drank the most coffee were also more likely to smoke, which is a well-known risk factor for heart disease. In this case, smoking was the lurking variable that positively biased the correlation between coffee and heart disease.
- Education and income: Analysts aimed to evaluate the relationship between education level and income. The study found a positive correlation, indicating that higher education levels were associated with higher incomes.
However, the researchers failed to account for work experience, which is a known factor that influences income. In this example, work experience was the lurking variable that positively biased the correlation between education and income.
- Activity level and bone density: In a study conducted, it was found that no relationship between activity level and bone density, even though we expected a positive correlation based on theoretical considerations.
However, upon further analysis, we discovered that the more active individuals tended to weigh less, and lower-weight individuals generally had lower bone density. In this case, weight was the lurking variable that negatively biased the correlation between activity level and bone density, initially hiding the genuine positive relationship.
- Population growth and traffic accidents: Studies have shown a strong correlation between popcorn consumption and the number of traffic accidents over the years. While it may seem counterintuitive, this correlation does not imply that higher popcorn consumption causes more traffic accidents or vice versa.
The lurking variable in this case is population growth. As the population increases, both popcorn consumption and the number of traffic accidents tend to rise, creating a spurious correlation.
- Weather and ice cream sales vs. shark attacks: A well-known example of a lurking variable is the positive correlation observed between ice cream sales and shark attacks. While these two variables may appear unrelated, the lurking variable in this case is the weather.
When the weather is warmer, more people tend to buy ice cream and engage in water-related activities, increasing the chances of shark encounters and attacks.
Identifying Lurking Variable: Strategies and Tools
While lurking variables can be challenging to detect, there are several strategies and tools researchers can employ to identify and mitigate their impact.
- Domain expertise and knowledge of potential confounders: One of the most effective ways to identify potential lurking variables is by leveraging domain expertise and knowledge of the subject matter.
Researchers with a deep understanding of the field can anticipate and account for variables that may influence the relationships under investigation. Collaborating with subject matter experts and conducting thorough literature reviews can help uncover potential confounders.
- Regression analysis and residual plots: Statistical techniques like regression analysis can be valuable in detecting the presence of lurking variables.
By examining residual plots, which display the differences between the observed and predicted values, researchers can identify patterns or trends that may indicate the influence of an unaccounted-for variable. If there is a systematic pattern in the residuals, it could suggest the presence of a lurking variable.
- Randomized experimental design: In experimental studies, incorporating a randomized design can help mitigate the impact of lurking variables.
By randomly assigning participants to different treatment groups, researchers can ensure that any potential lurking variables are distributed equally across the groups. This approach helps isolate the effect of the independent variable being studied and minimizes the influence of confounding factors.
While these strategies and tools can assist in identifying lurking variables, it’s important to note that some lurking variables may remain undetected, even with diligent efforts.
Controlling for Lurking Variables
Once lurking variables have been identified, researchers have several options for controlling or mitigating their impact on the study results.
- Inclusion in statistical models: If a lurking variable is known and can be measured, researchers can include it as an additional variable in their statistical models, such as regression analyses.
By explicitly accounting for the lurking variable, its effects can be separated from the variables of interest, reducing the risk of biased results.
- Holding constant or eliminating variables: In some cases, it may be possible to hold a lurking variable constant across all observations or eliminate its influence.
For example, in a study investigating the relationship between exercise and weight loss, researchers could control for dietary factors by providing all participants with the same standardized diet, eliminating diet as a potential lurking variable.
- Randomization in experiments: As mentioned earlier, randomization in experimental studies can help mitigate the impact of lurking variables.
By randomly assigning participants to treatment groups, researchers can ensure that any potential lurking variables are distributed equally across the groups, minimizing their influence on the observed effects.
It’s important to note that while these strategies can help control for known lurking variables, there may still be unknown or unmeasured confounding factors that could influence the results. Researchers should be transparent about the limitations of their studies and consider the potential impact of unaccounted-for factors on their findings.
Consequences of Ignoring Lurking Variable
Biased and misleading results: Lurking variables can introduce bias into statistical analyses, leading to distorted and misleading results. When the effects of these unaccounted-for variables are erroneously attributed to the variables under investigation, researchers may draw incorrect conclusions, potentially leading to flawed decision-making and ineffective policies.
Hiding true relationships: In some cases, lurking variables can mask or obscure genuine relationships between variables. For example, if a lurking variable negatively biases the correlation between two variables that are genuinely positively related, the true association may be hidden, preventing researchers from uncovering important insights.
Creating phantom correlations: Conversely, lurking variables can also create the appearance of correlations where none actually exist. These spurious correlations, such as the infamous example of ice cream sales and drowning incidents, can lead researchers astray, prompting them to investigate and attribute significance to relationships that are merely coincidental.
Invalidating research findings: If the presence and impact of lurking variables are not adequately addressed, the validity and reliability of research findings can be compromised. This can undermine the credibility of the study and call into question the conclusions drawn, potentially leading to wasted resources, misguided efforts, and even harmful consequences in fields like medicine or public policy.
As content strategists, it is crucial to emphasize the significance of these consequences and the importance of addressing lurking variables to ensure the integrity and trustworthiness of research findings.
Examples of Costly Lurking Variable Oversights
- Industry case studies: In the business world, ignoring lurking variables can lead to costly mistakes and missed opportunities. For example, a company may observe a correlation between a marketing campaign and increased sales, attributing the success solely to the campaign. However, if they fail to account for seasonal fluctuations or changes in the competitive landscape as lurking variables, they may make misguided decisions about future marketing strategies.
- Real-world impact: The consequences of overlooking lurking variables can extend beyond financial impacts and have far-reaching implications. In the field of public health, for instance, failing to account for socioeconomic factors or environmental exposures as potential lurking variables can lead to ineffective interventions and policies, potentially exacerbating health disparities or missing opportunities for prevention and treatment.
By highlighting examples of the costly consequences of ignoring lurking variables, we can underscore the importance of addressing these hidden factors and encourage researchers and decision-makers to prioritize rigorous and comprehensive analysis.
Best Practices for Addressing Lurking Variable
- Careful study design and variable selection: One of the most crucial steps in mitigating the impact of lurking variables is to carefully design the study and select relevant variables for investigation.
Conducting thorough literature reviews, consulting with subject matter experts, and considering potential confounding factors can help researchers identify and account for lurking variables from the outset.
- Statistical techniques like regression: Advanced statistical techniques, such as regression analysis, can be powerful tools for identifying and controlling for lurking variables.
By including potential confounding factors as independent variables in regression models, researchers can isolate and account for their effects, reducing the risk of biased results.
- Replication and validation: Encouraging the replication of studies and validating findings across different contexts and populations can help uncover the presence of lurking variables and ensure the robustness of research conclusions.
Consistent results across multiple studies increase confidence in the findings and minimize the impact of unaccounted-for factors.
- Limitations and future research: Researchers need to acknowledge the limitations of their studies and the potential influence of unidentified lurking variables.
By transparently discussing these limitations and suggesting avenues for future research, researchers can contribute to a culture of scientific integrity and encourage further exploration and refinement of their findings.
Emerging Methods and Software Tools
- Machine learning for variable detection: With the rapid advancement of machine learning and artificial intelligence techniques, new opportunities are emerging for the automated detection of lurking variables.
By leveraging large datasets and sophisticated algorithms, machine learning models can identify patterns and relationships that may reveal the presence of unaccounted-for factors, aiding researchers in uncovering potential confounders.
- Causal inference frameworks: Causal inference frameworks, such as structural equation modeling and directed acyclic graphs, provide powerful tools for disentangling causal relationships and accounting for lurking variables.
These methodologies allow researchers to explicitly model and test the influence of potential confounding factors, enhancing the robustness and interpretability of their findings.
- Open data and reproducibility: The growing emphasis on open data and reproducible research practices can contribute to better identification and management of lurking variables.
By making data and analysis scripts publicly available, researchers can facilitate peer review, replication, and collaborative efforts to uncover and address potential confounding factors, fostering transparency and scientific integrity.
Lurking Variables in Context: Ethics and Transparency
While addressing lurking variables is a methodological challenge, it also carries ethical implications and underscores the importance of responsible research practices and transparency.
- Responsible research practices: Researchers have an ethical obligation to design and conduct studies with rigorous methodologies that account for potential confounding factors.
Failing to identify and address lurking variables can lead to flawed conclusions, potentially causing harm or misinforming decision-makers and the public. Adhering to best practices and maintaining a commitment to scientific integrity is crucial. - Disclosing limitations: Transparency in research requires researchers to openly disclose the limitations of their studies, including the potential influence of unidentified lurking variables.
By acknowledging these limitations, researchers demonstrate intellectual honesty and enable others to interpret their findings within the appropriate context, fostering a culture of scientific accountability. - Interdisciplinary collaboration: Addressing lurking variables often requires interdisciplinary collaboration and the integration of diverse perspectives and expertise.
By encouraging collaboration among researchers from different fields, as well as stakeholders and subject matter experts, researchers can gain a more comprehensive understanding of potential confounding factors and develop more robust and reliable studies.
SixSigma.us offers both Live Virtual classes as well as Online Self-Paced training. Most option includes access to the same great Master Black Belt instructors that teach our World Class in-person sessions. Sign-up today!
Virtual Classroom Training Programs Self-Paced Online Training Programs