Exponentially Weighted Moving Average (EWMA) Charts. Everything to Know
Statistical procedure control methods assume basic estimation parts in organizations over industry spectra now. Among systems, Exponentially Weighted Moving Average (EWMA Chart) outlines have significance for distinguishing minor continuous procedure changes better than ordinary control diagrams.
EWMA chart delegate exponentially diminishing loads to past information focuses, concentrating additional on ongoing perceptions when exacting normal.
This unique weighting plan permits speedier reaction to little procedure movements, making it valuable for checking procedures requiring tight control and quick change to divergences.
Created by Stuart W. Roberts in 1959, EWMA charts discover wide utilizations over modern assembling, human services, funds, and quality of the executives.
Its aptitude for distinguishing little and proceeding with improvements quicker than different strategies settling on its top decisions for vital procedures where minuscule deviations carry colossal results.
Key Highlights
- The Exponentially Weighted Moving Average diagram, EWMA chart, is an innovative factual procedure control apparatus for checking little process information changes through time.
- It conveys exponentially diminishing loads to past focuses, concentrating more on ongoing estimations in deciding normal.
- EWMA chart recognizes little proceeding with movements quicker than standard Shewhart diagrams. It discovers wide utilizations over assembling, social insurance, funds, and other businesses for consistent observing and betterment.
- Vital parameters like smoothing constant (Ī») and oversight confines assume basic jobs in outline and execution.
- EWMA chart offers a better chance of discovery while relinquishing some factual execution contrasted and other diagrams.
What are EWMA Charts?
In quality administration and processing oversight, control diagrams assume pivotal parts for checking and distinguishing procedure evolutions or patterns.
Among techniques, Exponentially Weighted Moving Average diagrams (EWMA chart) have significance for quickly distinguishing little movements.
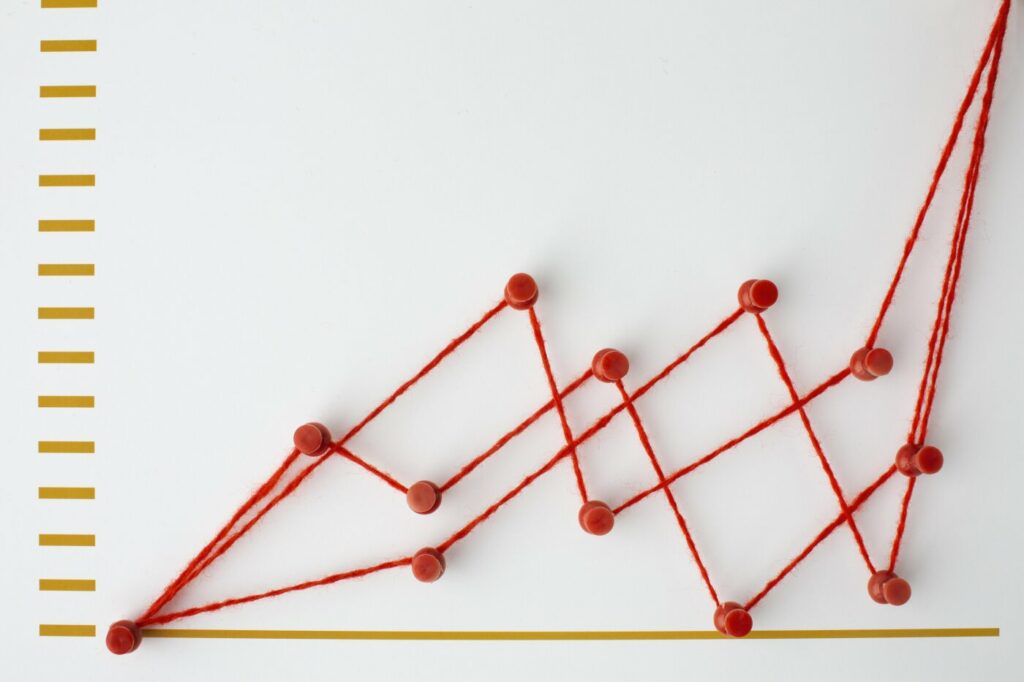
Presented by Roberts in 1959, EWMA chart assigns more weight to ongoing information focuses over more established centers. This aids in observing little stepwise deviations.
Not at all like standard Shewhart diagrams treating all centers similarly, EWMA chart sifts through irregular commotion while highlighting genuine procedure changes.
EWMA discovers employments over chip making, synthetic handling, and medication creation where tight administration decides quality and defect minimization. Applicabilities incorporate administrations, funds and human services.
By joining exponential smoothing, EWMA chart can early distinguish process advances. This permits quick remedy activities upholding non-conformity sparing and connected expenses, and dangers.
Understanding the EWMA Chart Statistic
The exponentially weighted moving average (EWMA) statistic is at the heart of the EWMA control chart. It is calculated recursively, giving more weight to recent data points than older ones. This weighting scheme makes the EWMA chart very responsive to small shifts in the process mean.
The EWMA statistic is defined by the equation:
EWMA(t) = λX(t) + (1-λ)EWMA(t-1)
Where:
X(t) is the current observation
EWMA(t-1) is the previous EWMA value
λ is the weighting factor (0 < λ ⤠1)
The Ī» (lambda) value determines how much weight is assigned to the current observation versus the previous EWMA value. A larger Ī» gives more weight to recent data. Common values for Ī» are 0.2 or 0.3 for detecting small process shifts, and 0.1 or less for detecting larger shifts.
The starting value for the EWMA chart is usually set to the process target (μ0) or a robust estimate of the process mean based on initial data. After calculating the first EWMA value, each subsequent point is found by applying the EWMA equation recursively.
One advantage of the EWMA statistic is that old data continually gets less and less weight, avoiding the situation where a very old data point can still significantly affect the EWMA value as it would in a simple moving average. This makes the EWMA chart very effective for process monitoring and detecting small shifts quickly.
However, the EWMA’s responsiveness comes at the cost of being less mathematically rigorous than other control charts like the Shewhart X-bar chart. Care must be taken in selecting and interpreting EWMA charts, especially for processes with changing variances over time.
Designing and Implementing EWMA Chart
When implementing EWMA chart for process monitoring, there are several key design considerations. First, you must determine an appropriate value for the weighting factor lambda (Ī»).
This smoothing parameter controls how much weight is given to recent data points versus older observations in calculating the EWMA statistic. A larger Ī» value, between 0.2-0.3, will make the EWMA more responsive to shifts but also more susceptible to false alarms from process noise. A smaller Ī», like 0.05-0.1, will smooth out noise but be less sensitive to real process changes.
The lambda value is related to the expected shift size you want to detect quickly. Larger shifts merit a higher Ī», while you would use a lower smoothing constant for monitoring smaller process drifts over time. Many practitioners use a Ī» of 0.2 as a reasonable starting point.
Next, you must establish rational control limits for your EWMA chart. The limits are typically set at ±3 sigma from the center line, though other multipliers like 2.7 or 3.5 sigma may be used. The control limit multiplier impacts the average run length (ARL) performance. Tighter limits detect shifts faster but increase the false alarm risk.
When implementing, it’s critical to obtain a sufficient number of in-control “baseline” observations to estimate the process mean and standard deviation for calculating initial EWMA values and control limits. At least 20-25 subgroups are recommended.
The EWMA is then plotted over time, with any points exceeding the control limits indicating an unstable or out-of-control process requiring investigation.
EWMA charting rules like run tests can enhance change detection sensitivity. For example, you may implement a rule where the chart signals if 8 consecutive points fall on one side of the center line. Supplemental rules improve signal detection but increase complexity.
It’s also important to clearly define rational sampling plans, subgroup sizes, measurement methods, and control procedures. Automation through statistical software can facilitate EWMA implementation, analysis, and response for efficient process monitoring.
EWMA Chart Performance and Comparisons
The EWMA chart offers several performance advantages over other control charting methods like Shewhart charts and CUSUM charts.
It is generally more sensitive at detecting small shifts in the process mean, providing quicker detection of issues compared to Shewhart charts. The EWMA chart is better suited for processes where small shifts are more likely to occur over time.
However, the EWMA chart is less effective than CUSUM charts for detecting large process shifts. The CUSUM method has an advantage for rapidly detecting larger mean shifts. So the relative performance depends on the expected magnitude of process shifts.
Average Run Length (ARL) is a common metric used to evaluate the performance of control charts. The ARL represents the average number of points that must be plotted before an out-of-control condition is detected. Smaller ARLs are desired to quickly identify process issues.
For the EWMA chart, the ARL performance is heavily influenced by the choice of the weighted parameter lambda (Ī»). As lambda increases towards 1, the EWMA becomes more sensitive to small shifts but less robust to noise. Optimal lambda values around 0.2 provide a good balance between shift detection and noise filtering.
Compared to Shewhart X-bar charts, properly designed EWMA charts have superior ARL performance for detecting small shifts of 0.5 to 1.5 sigma away from the mean. For larger shifts beyond 2 sigma, Shewhart charts tend to perform better.
The geometric moving average (GMA) and other weighted moving average schemes are alternatives that can provide similar performance to EWMA charts. However, the EWMA has the advantage of being based on recursive calculations that are easier to implement.
Control chart selection often involves evaluating which chart type works best for the expected process behavior and shift magnitudes. EWMA chart excel for its ability to quickly detect small, persistent shifts in manufacturing and other processes.
EWMA Chart Applications
The EWMA chart has several advanced applications and topics that are worth exploring in more depth. One key area is the selection of the lambda smoothing parameter.
While values like Ī»=0.2 are commonly used, the optimal lambda can vary based on the process being monitored. Some guidelines have been proposed for choosing lambda, such as using higher values like 0.4 when better change detection is needed at the expense of more false alarms. Simulation studies can help identify ideal lambda values for a given situation.
Another advanced topic is the combined use of EWMA chart with other control charts like Shewhart or CUSUM charts. This can capitalize on the respective strengths of the charts – for example, using an EWMA chart to detect small persistent shifts and a Shewhart chart to detect larger shifts. Various combinations and strategies have been researched.
The EWMA chart has also been extended to monitor other statistics beyond just the mean. For example, EWMA charts can be used to monitor process variability using statistics like the sample standard deviation. Multivariate applications monitoring multiple correlated variables simultaneously using a multivariate EWMA have also been studied.
Specialized applications of EWMA charts include short production run scenarios where there are frequent process setups. EWMA schemes have been developed to handle this by resetting parameters and warm-up periods.
Other areas include monitoring autocorrelated data, monitoring various types of processes like chemical processes, and integrating EWMA chart with engineering process control methodologies.
Overall, while the basic EWMA chart is relatively straightforward, advanced research continues to optimize its performance, extending it to new areas, and combining it with other monitoring techniques. As processes grow more complex, control charting methods like EWMA continue evolving to match modern monitoring needs.
Best Practices and Case Studies of EWMA Chart
When implementing EWMA charts, there are some important best practices to keep in mind to get the most value out of this monitoring technique. First, the choice of the weighted parameter Ī» is critical.
Generally, a Ī» between 0.2 and 0.3 is recommended as a starting point, but it should be adjusted based on the process characteristics. A smaller Ī» gives more weight to historical data, while a larger Ī» responds more quickly to process shifts.
It’s also important to establish rational control limits, typically at 3 sigma limits. However, tighter or wider limits may be used depending on the desired sensitivity. Control limit “zoning” with different levels of limits can provide enhanced change detection as well.
Proper control chart interpretation is vital. While a single point outside the control limits indicates an out-of-control process, runs or other non-random patterns can signal issues before points go beyond the limits when using the EWMA.
Applications
EWMA chart have proven valuable across many industries for monitoring processes over time. In semiconductor manufacturing, they are used to monitor critical dimensions and detect small process drifts before they lead to defective chips.
For call centers, EWMA chart can track call times, service levels, abandonment rates, and other KPIs to identify process shifts and underlying root causes. EWMA monitoring has also seen extensive use in healthcare for measures like patient wait times, lab turnaround times, infection rates, and more.
In the food industry, EWMA applications include monitoring oven temperatures, product weights, line speeds, and other parameters to maintain quality and consistency. Chemical companies utilize EWMAs to detect subtle changes in reactor conditions, raw material properties, and other leading indicators.
Case Study
A medical device manufacturer implemented EWMA charts to monitor a critical laser welding process. The process was fairly well controlled, so traditional Shewhart charts lacked the sensitivity to detect the small, persistent drifts that were leading to an unacceptable number of failures downstream.
By implementing an EWMA chart with a Ī» of 0.2, the engineers were able to identify when the process mean started to drift away from the target, even before individual points exceeded the control limits. This early detection allowed for root cause analysis and corrective actions before many more defects were produced, avoiding costly rework and scrap.
Conclusion
The EWMA chart is a powerful statistical process control tool that can be extremely useful for monitoring processes, especially those where small shifts are critical to detect. By applying exponentially weighted moving averages, the EWMA chart provides an effective way to track the process mean over time and quickly identify assignable causes of variation.
While the EWMA chart does require careful design through the selection of weighted values and control limits, it offers advantages over other control charts like enhanced sensitivity to small process shifts. When implemented correctly according to the guidelines outlined in this article, the EWMA chart can lead to improved process capability, reduced variation, and better overall quality.
For processes with sustained periods of stability punctuated by short runs of elevated variation, the EWMA chart may be preferred over a Shewhart X-bar chart.
For monitoring processes where small sustained shifts are more likely than large isolated events, the EWMA chart can outperform a CUSUM chart. Evaluating the relative costs and consequences of different shift detection scenarios can help determine if an EWMA is the optimal charting method.
As with any SPC technique, ensuring representative data, investigating special causes promptly, and developing an organizational commitment to continuous improvement are essential for maximizing the value of EWMA charts.
Applied judiciously alongside other process monitoring strategies, the EWMA chart can be a powerful tool for maintaining processes within specified limits and enhancing product/service quality over time.
SixSigma.us offers both Live Virtual classes as well as Online Self-Paced training. Most option includes access to the same great Master Black Belt instructors that teach our World Class in-person sessions. Sign-up today!
Virtual Classroom Training Programs Self-Paced Online Training Programs