The Ultimate Guide to Data Quality Tools: Top Solutions, Features, and Selection Criteria for 2025
As we approach 2025, data becomes of paramount importance, especially in making driven and accurate decisions.
Organizations are collecting large volumes of data, and without the correct tools in place, they risk the chance of not only losing the edge but also leaving multiple growth opportunities.
This article will equip you to:
- How to evaluate and select the right data quality tools for your organization
- Critical features that separate exceptional tools from mediocre ones
- Proven implementation strategies
- Pitfalls to avoid in your data quality journey
What Are Data Quality Tools?
Data quality tools are specialized software solutions that help organizations assess, improve, and maintain the integrity of their data assets.
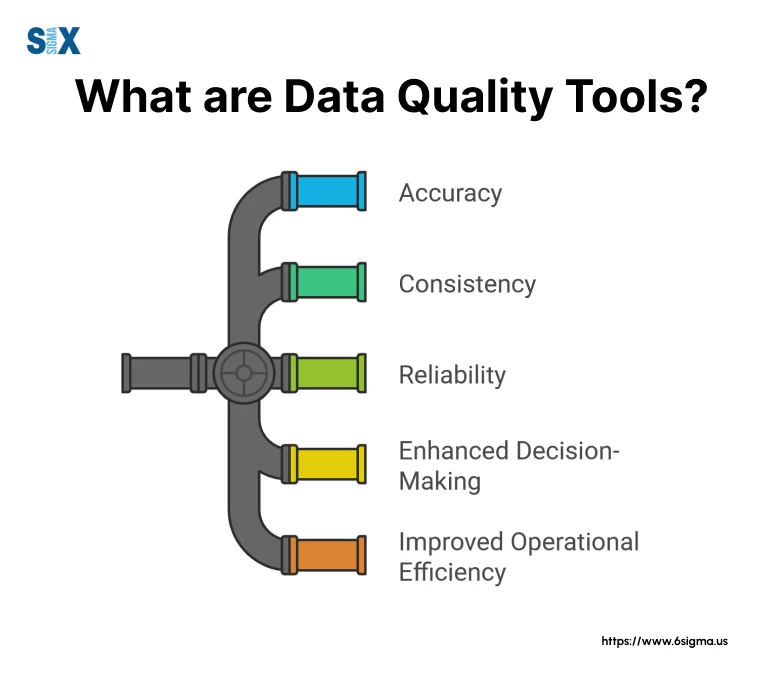
These tools typically perform several critical functions:
- Data Profiling: Analyzing data patterns and identifying anomalies
- Data Cleansing: Correcting errors and standardizing formats
- Data Validation: Ensuring data meets defined quality rules
- Data Enrichment: Enhancing existing data with additional information
- Data Monitoring: Continuously tracking data quality metrics
Types of Data Quality Tools
I categorize data quality tools into two main dimensions:
Open Source vs. Commercial Solutions
- Open Source Tools: I find these tools work well for organizations with strong technical teams and limited budgets. Examples include Apache Griffin and Great Expectations.
- Commercial Solutions: I find these more suitable for enterprises requiring comprehensive support and advanced features. Tools like Informatica and Talend fall into this category.
Cloud-based vs. On-premises Tools
- Cloud-based: There is a strong shift toward these owing to their scalability and ease of implementation.
- On-premises: These tools remain crucial, especially in highly regulated industries like healthcare and finance with strict data security requirements.
The 7 C’s of Data Quality
All effective data quality tools address what I call the 7 C’s of data quality:
- Completeness: Ensuring all required data is present
- Consistency: Maintaining uniform data across systems
- Correctness: Verifying the accuracy of data values
- Credibility: Establishing trust in data sources
- Conformity: Adhering to specified formats
- Clarity: Ensuring data is easily understood
- Currency: Maintaining up-to-date information
Problems These Data Quality Tools Solve
Data quality tools resolve numerous critical business challenges:
- Reducing manual data cleaning efforts by up to 80%
- Preventing costly decision-making errors
- Ensuring regulatory compliance
- Improving customer satisfaction through accurate reporting
- Enabling reliable analytics and AI initiatives
For example, during a recent project with a major healthcare provider, implementing proper data quality tools helped reduce reporting errors by 95% and saved over 200 hours of manual validation work monthly.
Transform Your Data Quality Management
Quality can’t be ‘inspected in’ ā it must be ‘built-in’ from the start. Join the Quality by Design course to learn how to build quality into processes!
Key Features to Look for in Data Quality Tools
Not all features carry equal weight. Letās look at which capabilities truly matter for successful data quality management.
Essential Features
I’ve identified these core features as essential:
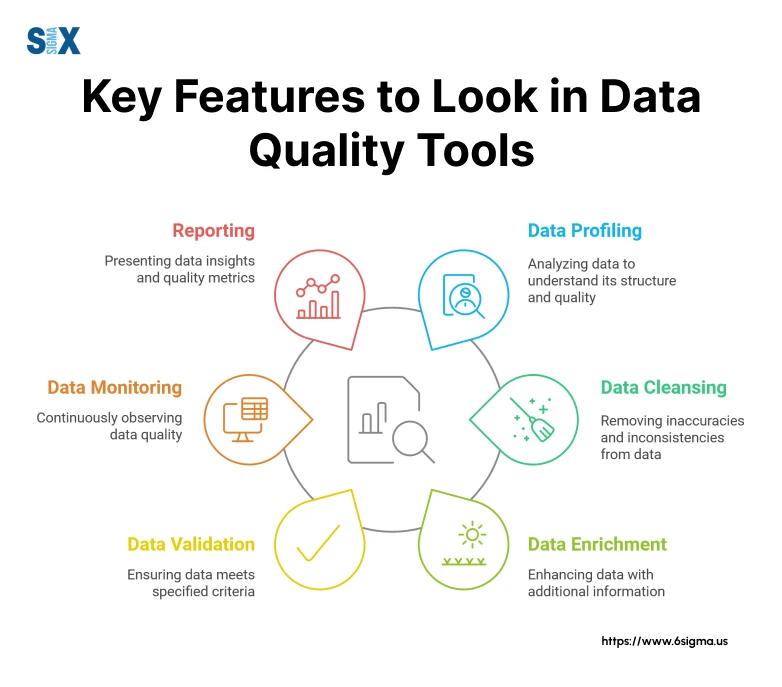
Data Profiling and Discovery
Robust profiling capabilities are non-negotiable. A quality data system must automatically analyze data patterns, identify anomalies, and provide statistical distributions.
Data Cleansing and Standardization
I can’t overemphasize the importance of automated cleansing capabilities. Your tool should handle:
- Format standardization
- Duplicate removal
- Missing value handling
- Error correction
- Pattern matching
Data Enrichment and Augmentation
I discovered that enrichment capabilities can transform good data into exceptional data by:
- Appending missing information
- Validating against external sources
- Enhancing existing records with additional context
Data Validation and Verification
Robust validation features should include:
- Business rule enforcement
- Cross-field validation
- Reference data verification
- Custom validation rules
Data Monitoring and Alerting
Real-time monitoring can prevent costly errors. Your tool should provide:
- Automated quality checks
- Customizable alerts
- Threshold monitoring
- Trend analysis
Reporting and Analytics with Data Quality Tools
Effective reporting features should deliver:
- Customizable dashboards
- Trend analysis
- Quality metrics
- ROI measurements
Integration Capabilities
Integration flexibility is crucial. Look for:
- API connectivity
- Native integrations
- Custom connector support
- Batch and real-time processing
Scalability and Performance with Data Quality Tools
Scalability features must include:
- Parallel processing capabilities
- Performance optimization
- Resource management
- Load balancing
User-Friendly Interface
Usability features should offer:
- Intuitive workflow design
- Visual data profiling
- Drag-and-drop functionality
- Role-based access control
Addressing Specific Challenges with Data Quality Tools
Here’s how these features address common challenges:
Data Accuracy Issues
- Profiling identifies potential problems
- Validation ensures data meets quality standards
- Monitoring prevents future issues
Efficiency Challenges
- Automation reduces manual effort
- Integration streamlines workflows
- User-friendly interfaces speed up adoption
Scalability Concerns
- Performance features handle growing data volumes
- Integration capabilities support expanding systems
- Monitoring scales with business growth
Master the Statistical Software That Powers Six Sigma Success
Don’t let statistics hold you back. Our Minitab Essentials training provides you with practical skills to analyze business processes effectively.
Top Data Quality Tools Comparison
Have a look into how different tools perform in real-world scenarios. Let’s examine the top data quality tools available today.
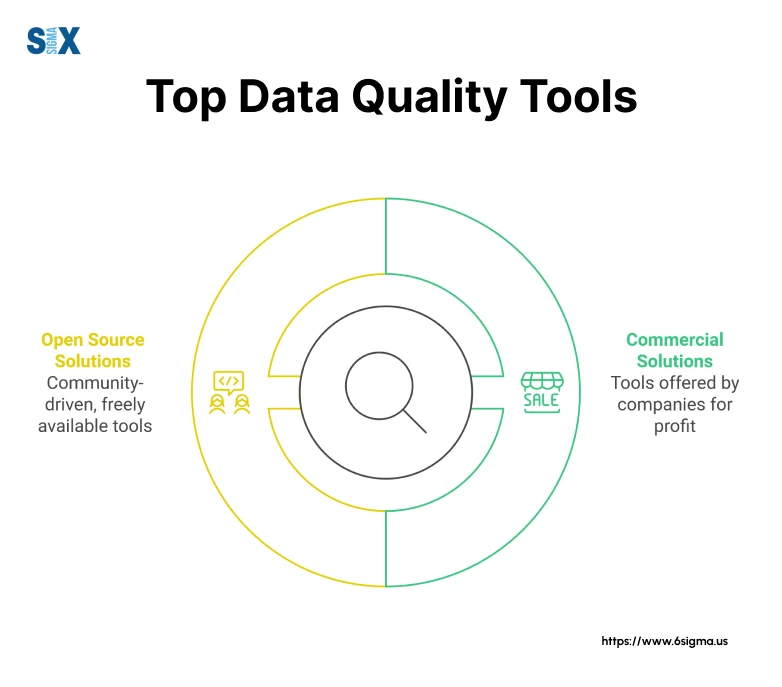
Commercial Solutions
Informatica Data Quality
Strengths:
- Enterprise-grade data profiling
- Advanced matching algorithms
- Robust cloud integration
Limitations:
- Complex setup process
- Higher price point
Best For: Large enterprises with complex data environments
Talend Data Quality
Strengths:
- Excellent visual interface
- Strong data integration capabilities
Limitations:
- Steep learning curve
- Resource-intensive
Best For: Mid-size to large organizations with technical teams
IBM InfoSphere
Strengths:
- Comprehensive governance features
- Advanced AI capabilities
- Excellent security controls
Limitations:
- Complex deployment
- Requires significant investment
Best For: Large enterprises with existing IBM infrastructure
SAS Data Quality
Strengths:
- Advanced analytics integration
- Strong statistical capabilities
- Excellent documentation
Limitations:
- Premium pricing
- Platform dependency
Best For: Organizations with heavy analytics requirements
Open Source Solutions
Great Expectations
Strengths:
- Python-native implementation
- Strong community support
- Excellent documentation
Limitations:
- Limited GUI
- Requires programming knowledge
Best For: Data teams with Python expertise
Apache Griffin
Strengths:
- Hadoop ecosystem integration
- Real-time validation
- Scalable architecture
Limitations:
- Complex setup
- Limited documentation
Best For: Organizations using Hadoop ecosystems
Additional Considerations of Data Quality Tools
Pricing Considerations:
- Enterprise solutions: $50,000-$250,000+ annually
- Mid-tier solutions: $25,000-$50,000 annually
- Open source: Free, but consider implementation costs
Implementation Complexity:
- Enterprise solutions: 3-6 months
- Mid-tier solutions: 1-3 months
- Open source solutions: 2-4 weeks with technical team
Key ROI Factors:
- Reduction in manual data cleaning (typically 60-80%)
- Improved decision accuracy (25-40%)
- Reduced data-related incidents (40-70%)
How to Choose the Right Data Quality Tool
Hereās a systematic approach to selecting the right data quality tools.
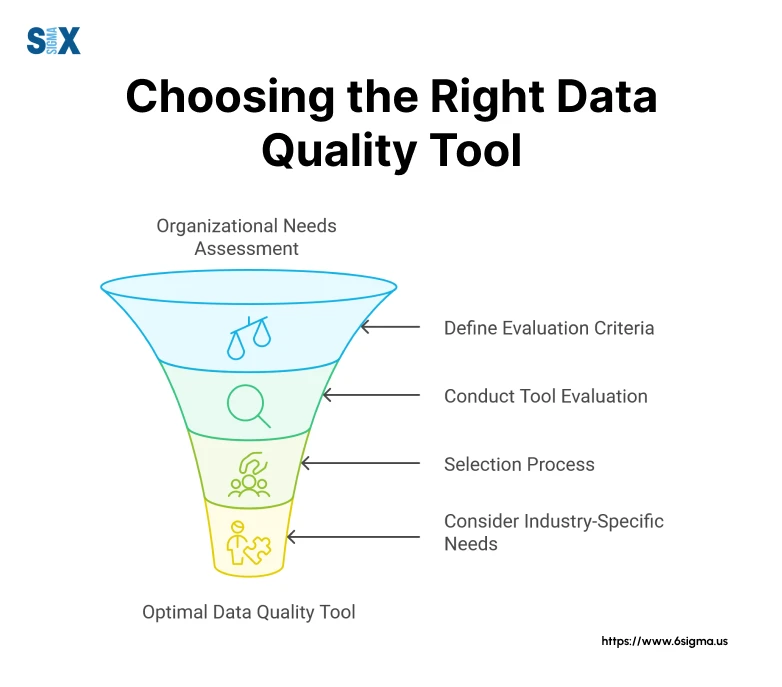
Assessing Organizational Needs
Before evaluating data quality tools in the market, I always guide organizations through these critical questions:
Current State Assessment
- What are your existing data quality pain points?
- How mature is your data management practice?
- What’s your current technical infrastructure?
Future State Vision
During my work with Intel, we identified these key considerations:
- Desired quality metrics and KPIs
- Growth projections
- Integration requirements
- Compliance needs
Evaluation Criteria Checklist for Data Quality Tools
You should prioritize these factors:
Essential Criteria:
- Technical compatibility
- Scalability requirements
- Security standards
- Integration capabilities
- Total cost of ownership
Supporting Criteria:
- Vendor stability
- Support quality
- Training resources
- Implementation timeline
- User interface
Step-by-Step Selection Process
Hereās the step-by-step selection process:
1. Initial Screening (2-3 weeks)
- Create vendor longlist
- Review technical documentation
- Assess pricing models
2. Detailed Evaluation (4-6 weeks)
- Request demos
- Check references
- Review security compliance
3. Proof of Concept (6-8 weeks)
- Define success criteria
- Test with real data
- Evaluate performance
- Assess user feedback
Industry-Specific Considerations for Data Quality Tools
I’ve identified these key industry factors:
Healthcare:
- HIPAA compliance
- Patient data security
- Integration with EMR systems
Financial Services:
- Real-time processing
- Regulatory compliance
- Audit trails
Manufacturing:
- Supply chain integration
- Quality control systems
- Production data handling
Pro Tips from My Experience:
1. Vendor Evaluation
- Don’t just evaluate the tool; assess the vendor’s stability and support
- Request customer references in your industry
- Review the product roadmap
2. POC Best Practices
- Use real data samples
- Test edge cases
- Involve end users
- Measure performance metrics
3. Common Pitfalls to Avoid
- Overlooking total cost of ownership
- Underestimating training needs
- Ignoring scalability requirements
- Neglecting change management
Turn Data into Reliable Insights with MSA
Accurate measurements are the foundation of quality decisions. MSA provides the tools and methodology to ensure your measurement systems are reliable and your data is trustworthy.
Measuring Data Quality: KPIs and Best Practices
I’ve learned that you can’t improve what you don’t measure. Letās look at a proven framework for measuring and monitoring data quality using effective data quality check tools.
Key Performance Indicators
I’ve identified these critical KPIs for measuring the quality of data:
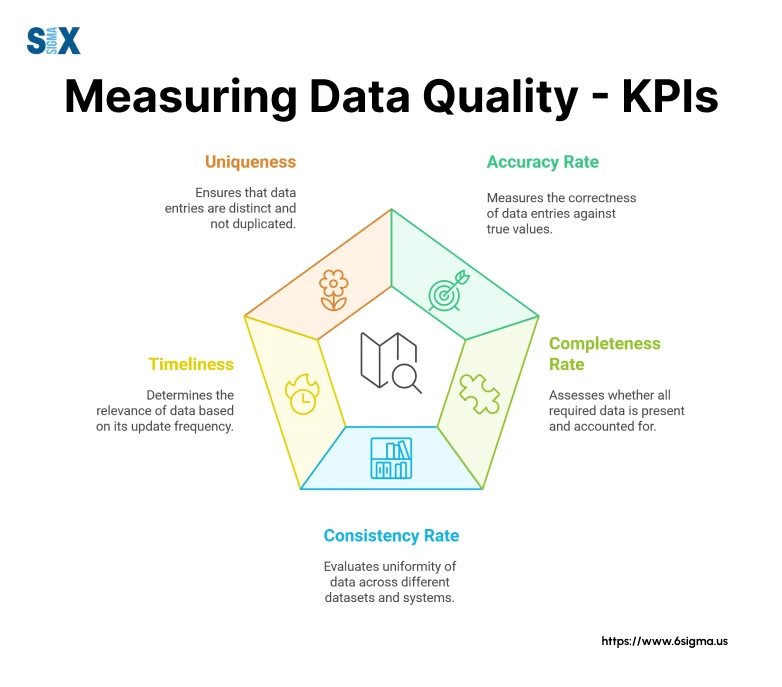
1. Accuracy Rate
- Error frequency
- Deviation from expected values
- Validation failure rates
Target: Aim for >98% accuracy
2. Completeness Rate
- Required field population
- Missing value frequency
- Data coverage metrics
Target: Minimum 95% completeness
3. Consistency Rate
- Cross-system data alignment
- Format standardization
- Value conformity
Target: >97% consistency across systems
4. Timeliness
- Data delivery speed
- Processing lag time
- Update frequency
Target: Based on business requirements
5. Uniqueness
- Duplicate record rates
- Matching accuracy
- Resolution success rates
Target: <1% duplicate rate
Assessment Methodologies
You should use these assessment approaches:
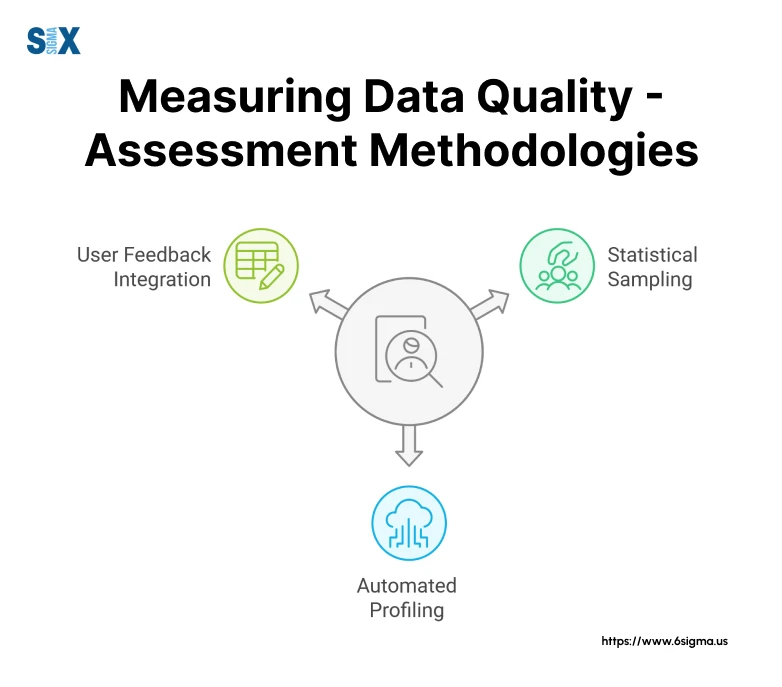
1. Statistical Sampling
- Random sampling for large datasets
- Stratified sampling for complex data
- Confidence level calculations
2. Automated Profiling
- Regular data scans
- Pattern analysis
- Anomaly detection
3. User Feedback Integration
- Structured feedback loops
- Issue tracking
- Impact assessment
Monitoring Strategies with Data Quality Tools
These strategies work best:
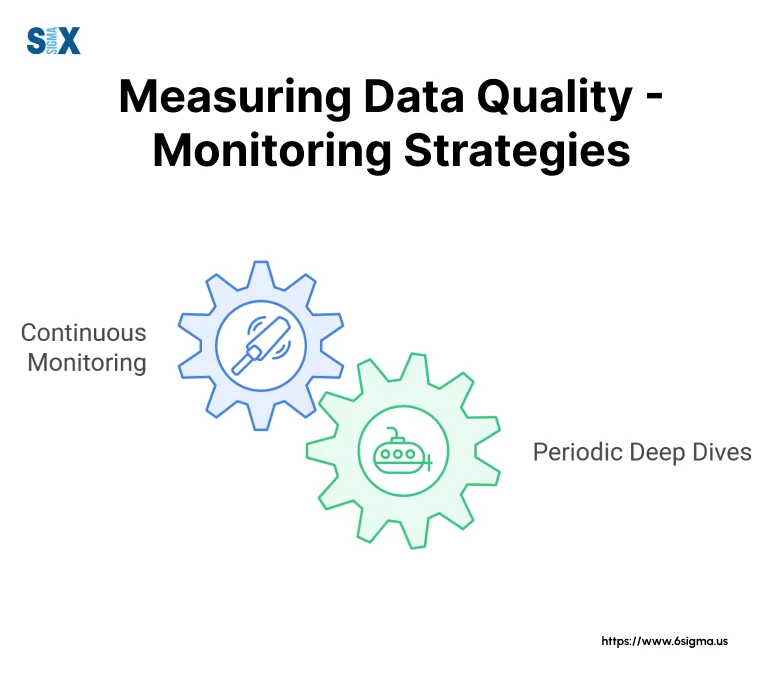
1. Continuous Monitoring
- Real-time quality checks
- Automated alerts
- Trend analysis
2. Periodic Deep Dives
- Monthly quality audits
- Quarterly trend reviews
- Annual assessments
Setting Realistic Targets
Based on my experience, I recommend:
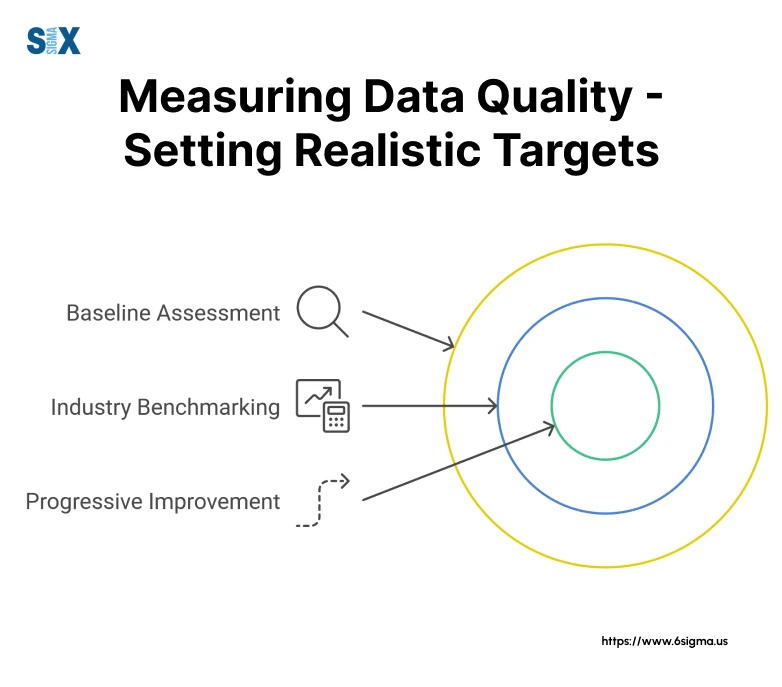
1. Baseline Assessment
- Document current performance
- Identify improvement opportunities
- Set incremental goals
2. Industry Benchmarking
- Compare with industry standards
- Adjust for organizational maturity
- Consider resource constraints
3. Progressive Improvement
- Set phased targets
- Monitor progress regularly
- Adjust based on results
Emerging Trends in Data Quality Tools
The trends today are revolutionizing how we approach data quality.
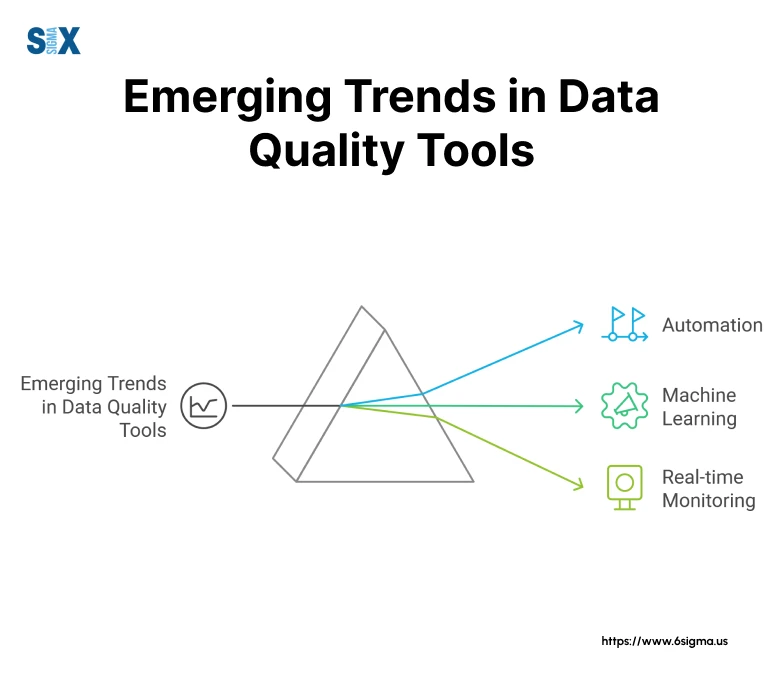
AI and Machine Learning Capabilities
I’ve observed AI transforming data quality tools in several ways:
- Automated anomaly detection
- Predictive quality scoring
- Self-learning validation rules
- Pattern recognition for data standardization
Real-time Management with Data Quality Tools
Real-time capabilities are becoming essential:
- Instant data validation
- Continuous quality monitoring
- Automated correction workflows
- Immediate alerting systems
Big Data Environments
I’ve seen data quality management tools evolve to handle:
- Massive data volumes
- Diverse data types
- Complex data relationships
- Distributed processing
Integration with Governance
Modern tools are increasingly combining quality and governance:
- Automated compliance checking
- Policy enforcement
- Data lineage tracking
- Privacy protection
Cloud/Hybrid Environments
I’m seeing a strong shift toward:
- Cloud-native solutions
- Hybrid deployment options
- Multi-cloud compatibility
- Edge computing support
Future Developments in Data Quality Tools
I predict these emerging trends:
1. Advanced Automation
- Self-healing data pipelines
- Automated root cause analysis
- Smart data remediation
2. Enhanced Collaboration
- Cross-functional workflows
- Integrated communication tools
- Collaborative problem-solving
3. Predictive Quality Management
- Early warning systems
- Risk prediction
- Proactive intervention
Case Studies and Expert Insights
Letās look at some impactful cases and insights using data quality tools.
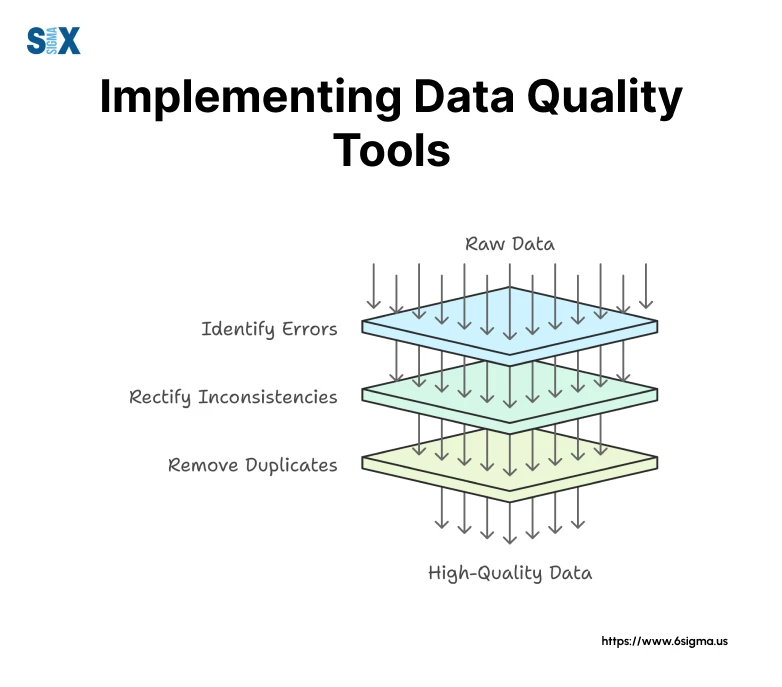
Manufacturing Sector
A manufacturing organization operating in the industry, worker safety, and consumer goods, implemented a comprehensive data quality tool that resulted in:
- 87% reduction in data errors
- $2.3M annual savings
- 65% faster reporting cycles
Key Success Factors:
- Phased implementation approach
- Strong executive sponsorship
- Comprehensive training program
Technology Industry
A leading semiconductor and circuit manufacturing company achieved:
- 92% automation of quality checks
- 4-hour reduction in daily data validation
- Zero critical data incidents in the first year
Implementation Strategy:
- Started with high-impact areas
- Built automated workflows
- Established clear ownership
Insights
Here are critical success factors:
1. Leadership Engagement
The most successful implementations I’ve seen had strong C-suite support from day one.
2. Change Management
Through my international consulting experience, I’ve found:
- Early stakeholder involvement is crucial
- Regular communication maintains momentum
- Training must be role-specific
3. Technical Considerations
You should emphasize on:
- Start with data profiling
- Build scalable architectures
- Plan for integration needs
Best Practices and Pitfalls
Best Practices:
- Begin with a pilot program
- Document baseline metrics
- Create clear success criteria
- Establish governance structure
- Plan for scalability
Common Pitfalls:
- Rushing implementation
- Neglecting user training
- Ignoring change management
- Underestimating resource needs
- Lacking clear ownership
Going Ahead
The success of your data quality initiative depends on choosing the right tools and implementing them strategically.
Key Takeaways:
- Data quality management tools are essential investments for any data-driven organization
- The best data quality tools combine automation, scalability, and user-friendly interfaces
- Success requires alignment between technical capabilities and business objectives
- Implementation should be phased and methodical
Final Recommendations:
- Start with a thorough assessment of your current data landscape
- Choose tools that match your organization’s maturity level
- Prioritize solutions offering scalability and integration capabilities
- Invest in proper training and change management
- Establish clear metrics for measuring success
Frequently Asked Questions
You should start with:
– Assessing current data quality levels
– Identifying critical data elements
– Setting clear quality objectives
– Selecting appropriate tools
– Building a phased implementation plan
From my experience:
– Small organizations: 2-3 months
– Mid-sized companies: 3-6 months
– Enterprise-level: 6-12 months
Success depends heavily on organizational readiness and commitment.
You can typically expect:
– 30-40% reduction in manual data cleaning
– 50-60% fewer data-related incidents
– 25-35% improvement in decision-making speed
ROI usually becomes evident within 6-12 months.
You should:
– Involve users early in tool selection
– Provide role-specific training
– Create clear documentation
– Establish support systems
– Demonstrate quick wins
The common implementation challenges are:
– Resistance to change
– Limited resources
– Technical integration issues
– Lack of executive support
– Unclear ownership
Successful organizations focus on:
– Regular monitoring and reporting
– Continuous training
– Process documentation
– Regular tool updates
– Feedback incorporation
SixSigma.us offers both Live Virtual classes as well as Online Self-Paced training. Most option includes access to the same great Master Black Belt instructors that teach our World Class in-person sessions. Sign-up today!
Virtual Classroom Training Programs Self-Paced Online Training Programs