Data Collection Plan: The Foundation of Six Sigma Success
A data collection plan serves as the roadmap for gathering, analyzing, and utilizing information within Six Sigma projects.
This structured approach ensures organizations capture relevant data that drives process improvements and quality enhancements across operations.
Key Highlight
- Systematic Data Collection Methods
- Strategic Sampling Techniques
- Quality Control Process Integration
- Digital Tools for Data Management
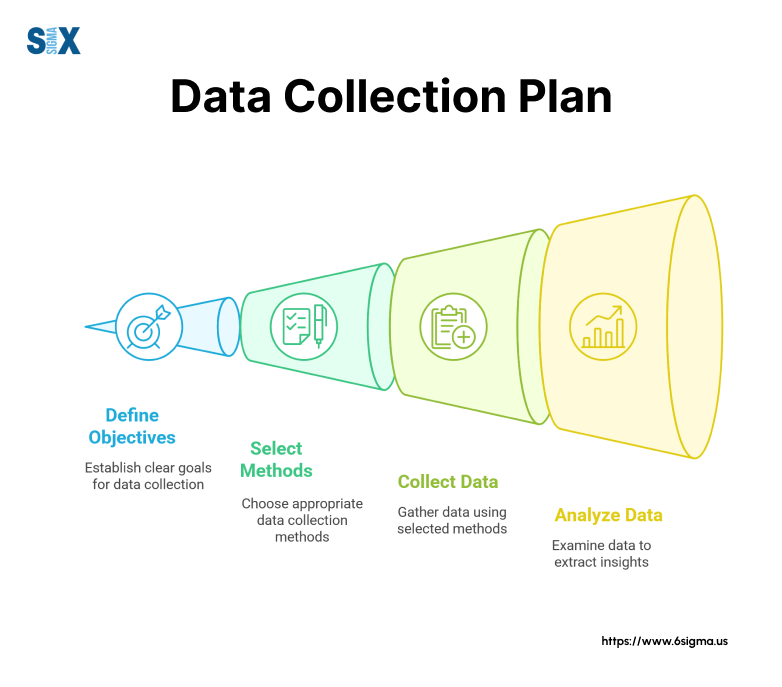
Understanding Data Collection Plans in Six Sigma
Six Sigma data collection plans establish structured methods for gathering and organizing information that drives process improvements.
These plans ensure teams collect relevant data that directly supports project objectives and organizational goals.
Purpose and Core Objectives of a Data Collection Plan
The primary purpose of a data collection plan centers on gathering accurate, relevant data that measures process performance.
Quality teams use this information to identify variations, track improvements, and validate changes within their Six Sigma projects.
The plan creates standardized protocols that eliminate guesswork and ensure consistency across different measurement phases.
Organizations implement data collection plans to achieve several key objectives. Teams need reliable data to establish baseline performance metrics.
Project managers require accurate measurements to track improvement initiatives. Quality control specialists depend on consistent data to monitor process variations and identify potential issues before they escalate.
Essential Components of Data Collection
A robust data collection plan contains several critical elements that work together to ensure successful implementation.
The measurement system defines specific metrics and collection methods. Sampling strategies determine how teams will select data points. Documentation protocols establish standard recording procedures and storage methods.
The plan also includes validation procedures to ensure data accuracy. Quality checks verify that collected information meets established standards.
Analysis methods outline how teams will process and interpret the gathered data. These components create a framework that supports reliable data collection throughout the project lifecycle.
Integration of Data Collection Plan with DMAIC Framework
Data collection plans play vital roles in each phase of the DMAIC methodology.
During the Define phase, teams use collected data to scope project parameters.
The Measure phase relies heavily on structured data collection to establish baseline performance. Analysis phases depend on accurate data to identify root causes and potential solutions.
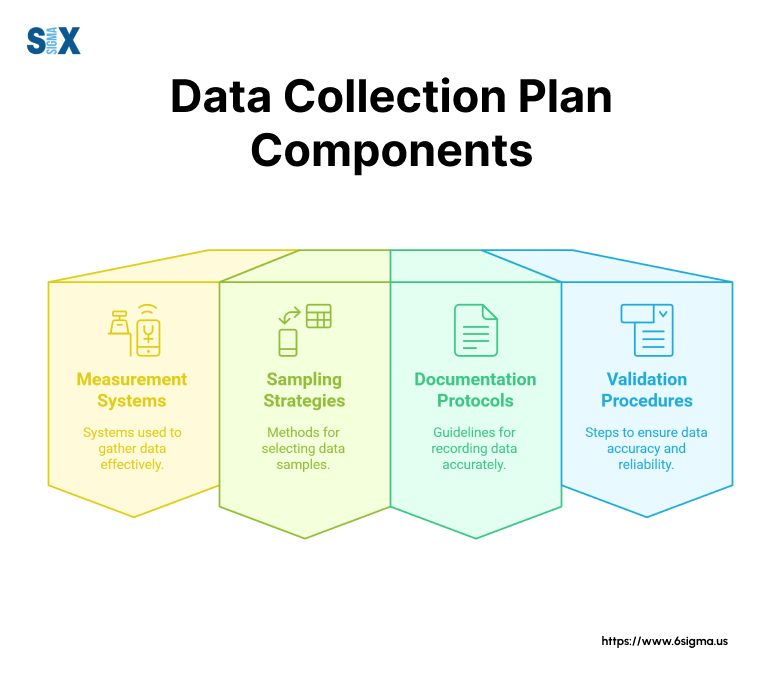
The Improve phase requires ongoing data collection to validate proposed solutions. Control phases use collected data to monitor long-term process stability.
This integration ensures that data collection supports continuous improvement efforts throughout the project lifecycle.
Measurement System Analysis
Quality teams must evaluate their measurement systems to ensure reliable data collection. Gauge R&R studies assess measurement precision and accuracy.
Calibration procedures maintain equipment reliability. Regular system audits verify that collection methods remain consistent and effective over time.
These systematic approaches to data collection help organizations maintain high standards of quality control while supporting continuous improvement initiatives.
By following structured plans, teams can gather the information needed to drive meaningful process improvements and achieve their Six Sigma objectives.
Join our Lean Six Sigma Overview course to learn proven methodologies that boost organizational productivity.
Steps to Create a Data Collection Plan
Creating an effective data collection plan requires careful planning and systematic execution.
Each step builds upon the previous one to ensure the gathered data supports project objectives and drives meaningful improvements.
Define Problems and Set Clear Objectives
The first step involves identifying specific problems that need solving and establishing measurable objectives. Quality teams must outline clear project goals that align with organizational targets.
This clarity helps determine what data needs collection and how it will support decision-making processes.
Teams should document current process performance metrics and desired improvement targets. These benchmarks guide the selection of appropriate measurement parameters and success criteria.
Setting specific, measurable objectives ensures the data collection effort remains focused and purposeful.
Identify Relevant Data Sources and Types
Once objectives are clear, teams must identify where to find relevant data. Process outputs, customer feedback, equipment readings, and quality inspection reports often serve as primary data sources.
Teams should evaluate both internal and external sources to ensure they capture all necessary information.
The type of data needed influences collection methods and analysis approaches. Quantitative data might include measurements, counts, or time studies.
Qualitative data could encompass customer complaints, operator observations, or process descriptions. Both types play crucial roles in understanding process performance.
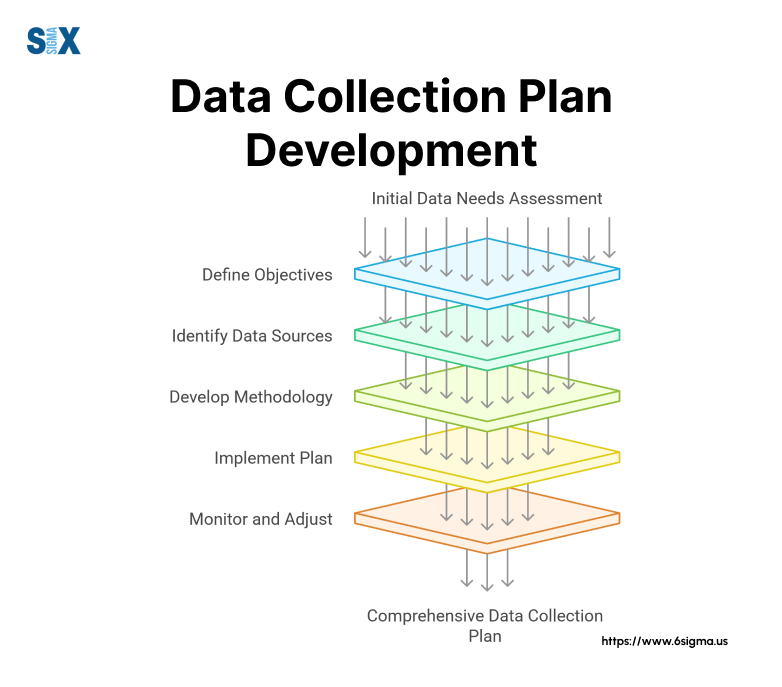
Select Appropriate Collection Methods
Different data types require different collection methods. Automated systems might gather equipment performance data. Manual observation might track operator behaviors.
Surveys could collect customer satisfaction information. Teams must choose methods that balance accuracy, efficiency, and resource availability.
Determine Sampling Parameters for the Data Collection Plan
Proper sampling ensures collected data represents the entire process accurately. Teams must determine appropriate sample sizes that provide statistical validity.
Sampling techniques should align with project objectives and practical constraints like time and resources.
Design Collection Tools and Forms
Standard forms and tools ensure consistent data collection across different operators and shifts. Digital templates streamline data entry and reduce errors.
Collection forms should include clear instructions, measurement units, and space for relevant observations.
Set Timeline and Assign Responsibilities for the Data Collection Plan
The plan must specify when data collection occurs and who handles specific tasks. Clear timelines help teams coordinate efforts and ensure timely data gathering.
Assigned responsibilities prevent confusion and ensure accountability throughout the collection process.
Establish Analysis and Reporting Protocols
Teams need predetermined methods for analyzing collected data and reporting findings. Analysis plans should outline statistical tools and techniques to use. Reporting formats must present findings clearly to support decision-making processes.
Regular review meetings help teams assess data quality and collection progress. These reviews identify potential issues early and allow for necessary adjustments to the collection process.
Continuous monitoring ensures the plan remains effective throughout the project lifecycle.
Data Collection Methods in Six Sigma
Six Sigma projects employ various data collection methods to gather accurate and relevant information. The choice of method depends on the type of data needed, project objectives, and available resources.
Surveys and Questionnaires
Structured surveys provide standardized ways to collect both quantitative and qualitative data. Digital survey platforms enable rapid data gathering from large groups.
Teams can design surveys to measure customer satisfaction, process effectiveness, or employee feedback.
Quality teams must craft clear, unbiased questions that yield actionable data. Survey design should consider response formats, question sequence, and completion time.
Regular survey updates ensure questions remain relevant to current project needs.
Interviews and Focus Groups
Direct interviews yield detailed insights into process operations and potential improvements. One-on-one sessions allow deeper exploration of specific issues.
Focus groups generate discussions that reveal unexpected problem areas or solution opportunities.
These methods work best when following structured protocols. Interview guides ensure consistent question coverage across sessions.
Recording mechanisms capture detailed responses for later analysis. Skilled facilitators keep discussions focused on relevant topics.
Observations and Direct Measurements
Physical observations capture actual process performance data. Time studies measure task durations and identify inefficiencies.
Quality checks record defect rates and product variations. Environmental monitoring tracks workplace conditions affecting process outcomes.
Teams must standardize observation methods to ensure consistent data collection. Measurement tools require proper calibration and maintenance.
Observer training reduces variation in recorded data. Documentation protocols preserve important contextual information.
Historical Data Analysis with a Data Collection Plan
Existing databases often contain valuable process information. Production records show output trends over time.
Maintenance logs reveal equipment reliability patterns. Quality reports document past improvement efforts and their results.
Teams should verify data accuracy before analysis begins. Data cleaning removes errors and inconsistencies. Format standardization enables efficient processing. Proper documentation tracks data sources and collection dates.
Automated Collection Systems
Modern technology enables automated data gathering from various sources. Sensors monitor equipment performance continuously. Software systems track process metrics automatically.
Digital platforms integrate data from multiple collection points.
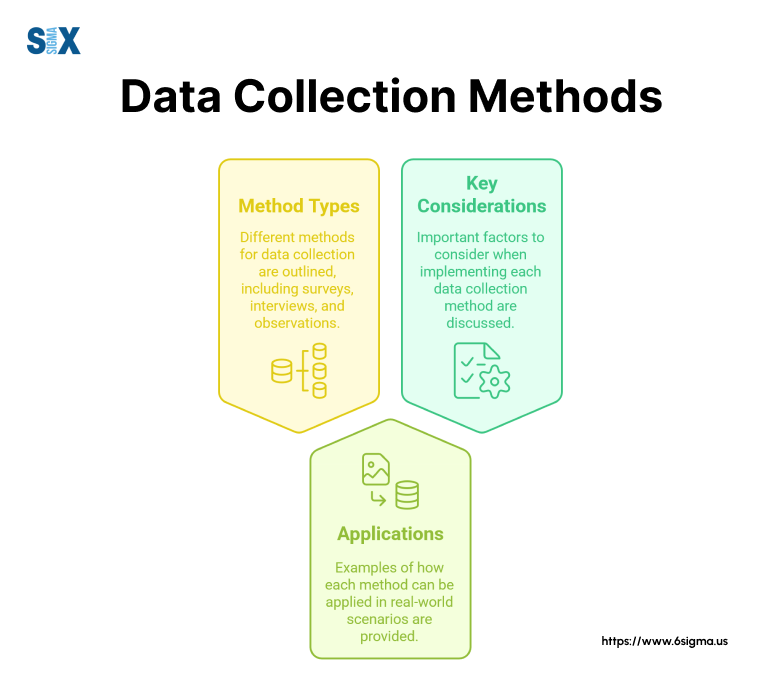
Automated systems require regular maintenance and validation. Data verification protocols ensure accuracy.
Backup systems prevent information loss. Security measures protect sensitive data.
Integration of Multiple Methods
Most Six Sigma projects benefit from combining several collection methods. Survey data might validate observation findings.
Automated systems complement manual measurements. Historical analysis guides new data collection efforts.
Method selection should align with project goals and resources. Teams must balance data quality against collection costs. Regular evaluation ensures chosen methods continue meeting project needs.
Quality professionals recognize that successful data collection requires careful method selection and implementation.
By choosing appropriate methods and following established protocols, teams can gather the information needed to drive process improvements and achieve project objectives.
Sampling Techniques in Six Sigma
Sampling techniques form a crucial part of any data collection plan, enabling teams to gather representative data efficiently.
The right sampling method ensures accurate conclusions while optimizing resource usage in Six Sigma projects.
Random Sampling Methods
Simple random sampling provides every element in a population an equal chance of selection. This method eliminates bias and produces statistically valid results.
Manufacturing teams might use random sampling to inspect product quality from production lines. Quality control specialists often employ random sampling for process audits.
The success of random sampling depends on proper selection procedures. Teams must use validated random number generators or selection tools.
Sample sizes need careful calculation to ensure statistical significance. Documentation should track selection methods and sampling parameters.
Stratified Sampling Approaches for the Data Collection Plan
Stratified sampling divides populations into distinct groups before selection begins. This method ensures representation from all important subgroups within a process.
Production teams might stratify samples by shift, machine, or operator to identify variation sources.
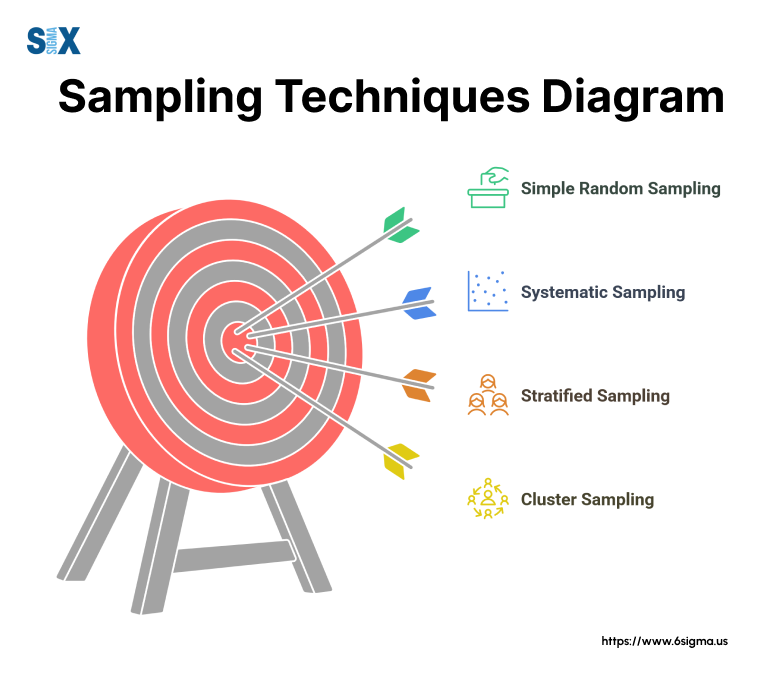
The effectiveness of stratified sampling relies on proper group definition. Teams must identify meaningful stratification factors.
Group sizes should reflect population proportions. Selection within strata follows random sampling principles.
Systematic Sampling Implementation
Systematic sampling selects items at fixed intervals throughout a population. Quality teams might inspect every nth item from a production line.
This method works well for continuous processes where timing or sequence matters.
Teams must choose appropriate sampling intervals. The interval should avoid alignment with process cycles.
Regular validation ensures sampling patterns remain representative. Adjustments might be needed if process conditions change.
Cluster Sampling Applications with a Data Collection Plan
Cluster sampling groups population elements into clusters for selection. This technique helps when populations spread across different locations or time periods.
Organizations might use cluster sampling for multi-site quality audits.
Successful cluster sampling requires careful cluster definition. Clusters should represent the whole population.
Selection methods must ensure unbiased cluster choice. Sample sizes need adjustment for cluster effects.
Selection Criteria For Sampling Methods
Teams should consider several factors when choosing sampling techniques. Process characteristics influence method selection.
Resource constraints affect sample sizes. Quality requirements determine acceptable precision levels.
Method selection impacts data collection procedures. Each technique requires specific implementation protocols. Training ensures consistent application across teams.
Documentation maintains sampling integrity over time.
Validation and Monitoring
Regular validation ensures sampling methods remain effective. Teams should monitor sample representativeness. Statistical tests verify sampling accuracy.
Adjustments address any identified issues.
Quality professionals understand that proper sampling forms the foundation of reliable data collection.
By selecting and implementing appropriate sampling techniques, teams can gather meaningful data while managing resource constraints effectively.
The Key Role of Data Collection in Six Sigma
Successful Six Sigma projects rely on robust data collection strategies that ensure accuracy, reliability, and ethical compliance.
Following established best practices helps teams gather high-quality data that drives meaningful process improvements.
Ensuring Data Accuracy and Reliability
Data accuracy begins with proper measurement system design. Calibrated instruments provide reliable readings.
Standardized procedures reduce variation between operators. Regular equipment maintenance prevents measurement drift.
Teams must implement quality checks throughout the collection process. Cross-verification identifies potential errors early.
Documentation trails track data sources and collection methods. Regular audits verify adherence to established procedures.
Minimizing Collection Bias
Bias prevention requires careful attention to collection procedures. Standardized protocols ensure consistent data gathering across shifts and locations.
Proper training reduces operator influence on measurements. Random sampling helps eliminate selection bias.
Collection timing should avoid known process variations. Multiple data collectors reduce individual bias. Blind studies prevent expectation bias. Regular rotation of collection responsibilities maintains objectivity.
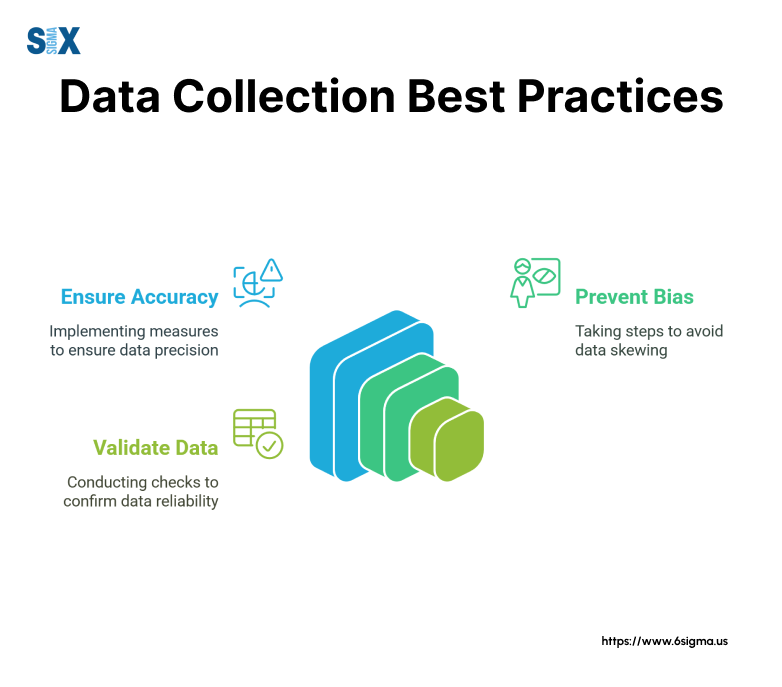
Managing Incomplete Data Sets with a Data Collection Plan
Missing data requires systematic handling approaches. Teams should document reasons for missing values.
Statistical methods can address data gaps appropriately. Collection procedures might need adjustment to prevent future missing data.
Incomplete data sets need careful evaluation before analysis. Valid statistical techniques can compensate for missing values.
Documentation should track all data handling decisions. Teams must consider the impact of missing data on conclusions.
Data Validation Methods
Validation techniques verify data quality throughout collection. Range checks identify outliers and impossible values. Consistency checks reveal logical errors. Cross-referencing confirms data alignment across sources.
Statistical process control charts monitor data patterns. Trend analysis identifies unusual shifts. Control limits help detect special cause variation.
Regular validation maintains data integrity over time.
Ethical Data Collection Practices
Ethical considerations guide all data collection activities. Privacy protection safeguards sensitive information. Consent procedures ensure proper data usage. Security measures protect collected data.
Teams must balance data needs against privacy concerns. Clear policies guide data handling and storage. Regular training reinforces ethical practices. Documentation demonstrates compliance with regulations.
Quality Control Measures with a Data Collection Plan
Quality control procedures ensure reliable data collection. Check sheets verify complete data capture. Visual controls guide proper measurement techniques. Error-proofing prevents common mistakes.
Regular reviews assess collection effectiveness. Teams should monitor error rates and patterns. Corrective actions address identified issues. Continuous improvement enhances collection procedures.
Quality professionals recognize that strong data collection practices form the foundation of successful Six Sigma projects.
By following these best practices, teams can ensure their data supports valid analysis and drives meaningful process improvements.
Learn how to analyze data effectively and identify root causes in our focused training program
Common Challenges and Solutions in Data Collection
Organizations implementing lean Six Sigma data collection plans often encounter several obstacles. Understanding these challenges and their solutions helps teams maintain effective data gathering processes while ensuring project success.
Addressing Resistance to Data Collection
Employee resistance often stems from concerns about increased workload or performance monitoring. Clear communication about project objectives helps alleviate these concerns.
Training programs demonstrate the value of data collection to process improvement. Regular feedback sessions show how collected data drives positive changes.
Teams can reduce resistance through streamlined collection methods. Automated tools minimize manual effort. Simple documentation forms speed up recording processes.
Visual management systems make data collection more intuitive.
Managing Resource Limitations with a Data Collection Plan
Resource constraints frequently impact data collection efforts. Smart allocation of available resources maximizes collection efficiency.
Prioritization frameworks help teams focus on critical data points. Scheduling optimization ensures efficient use of personnel and equipment.
Cost-effective solutions help overcome budget limitations. Digital tools replace expensive manual processes. Cross-training staff increases collection flexibility. Shared resources across departments reduce equipment needs.
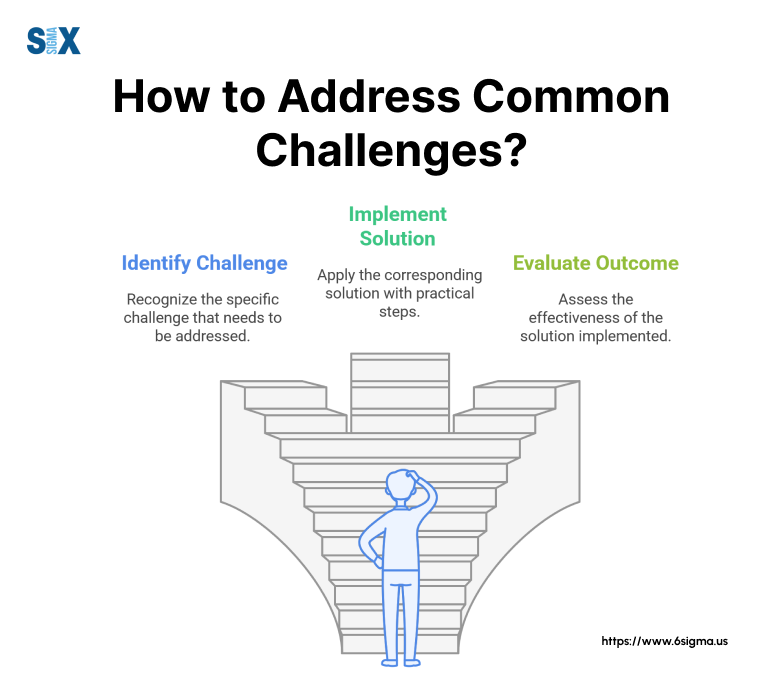
Improving Data Quality Issues
Poor data quality undermines analysis efforts and project outcomes. Standard operating procedures ensure consistent collection methods. Quality checks catch errors before they impact analysis. Regular calibration maintains measurement accuracy.
Teams should implement error prevention strategies. Automated validation rules flag incorrect entries. Real-time monitoring identifies quality issues quickly. Correction protocols address problems systematically.
Overcoming Technology Barriers
Technical challenges often hinder effective data collection. User-friendly interfaces reduce technical barriers. Mobile solutions enable field data collection. Cloud storage provides accessible data storage options.
Integration issues require systematic solutions. Compatible systems ensure smooth data transfer. Backup procedures prevent data loss. Security measures protect sensitive information.
Building Sustainable Solutions with a Data Collection Plan
Long-term success requires sustainable collection methods. Regular system updates maintain technological relevance. Continuous training develops team capabilities. Performance monitoring identifies improvement opportunities.
Documentation helps preserve institutional knowledge. Process maps guide consistent implementation. Training materials support new team members. Regular reviews ensure methods remain effective.
Measuring Implementation Success
Teams must track solution effectiveness over time. Performance metrics monitor collection efficiency. Quality indicators assess data reliability. Cost analysis validates resource utilization.
Regular assessments guide continuous improvement. Teams should evaluate solution impacts periodically. Adjustment strategies address emerging challenges. Success stories motivate continued engagement.
Quality professionals understand that overcoming data collection challenges requires systematic approaches and sustained effort.
By implementing targeted solutions and monitoring their effectiveness, teams can maintain reliable data collection processes that support successful Six Sigma projects.
Implementing Effective Data Collection Plans: Moving Forward
A well-designed data collection plan serves as the foundation for successful Six Sigma initiatives.
The systematic approach to gathering, analyzing, and utilizing data drives measurable improvements across organizational processes.
Key Elements for Success
Successful data collection plans incorporate several critical components. Structured methodologies ensure consistent data gathering across teams.
Proper sampling techniques provide representative data sets. Quality control measures maintain data integrity throughout the collection process.
Teams must focus on selecting appropriate collection methods for their specific needs. Digital tools streamline data gathering operations.
Automated systems reduce manual errors. Validation procedures verify data accuracy and reliability.
Impact on Process Improvement
Effective data collection directly influences project outcomes. Quality teams rely on accurate data to identify process variations.
Analysis results guide improvement decisions. Performance metrics track progress toward project goals.
Organizations see tangible benefits from robust data collection practices. Process efficiency increases through targeted improvements.
Quality metrics show measurable gains. Customer satisfaction rises with enhanced product consistency.
Steps for Implementation
Organizations ready to enhance their data collection practices should begin with clear objectives. Team training ensures proper methodology application.
Regular monitoring validates collection effectiveness. Continuous improvement refines processes over time.
Success requires commitment from all organizational levels. Leadership support provides necessary resources. Team engagement ensures consistent execution. Regular reviews maintain focus on collection goals.
Quality professionals recognize that strong data collection forms the backbone of process improvement initiatives.
By implementing structured collection plans, organizations position themselves for sustained success in their Six Sigma journey.
The path forward requires dedication to maintaining high standards in data collection practices. Teams should regularly evaluate their methods, embrace new technologies, and adjust strategies as needed.
Through consistent application of proven techniques, organizations can achieve their quality improvement objectives and drive meaningful process enhancements.
SixSigma.us offers both Live Virtual classes as well as Online Self-Paced training. Most option includes access to the same great Master Black Belt instructors that teach our World Class in-person sessions. Sign-up today!
Virtual Classroom Training Programs Self-Paced Online Training Programs