Analytical Modeling: A Guide to Data-Driven Decision Making
Analytical modeling is a comprehensive approach that employs mathematical models, statistical algorithms, and data analysis methods to understand, interpret, and predict outcomes based on historical data and known variables.
At its core, it represents a quantitative and computational framework for dissecting intricate systems, identifying patterns, and informing strategic decision-making processes.
Key Highlights
- Understanding Analytical modeling
- Learn key concepts and techniques
- Benefits of Analytical modeling
- Case studies span various domains
- Best practices
- Emerging trends for further revolutionize the field of analytical modeling.
Introduction to Analytical Modeling
Analytical modeling, or analytics modeling, is a comprehensive approach that employs mathematical models, statistical algorithms, and data analysis techniques to gain insights, make predictions, and inform business strategies.
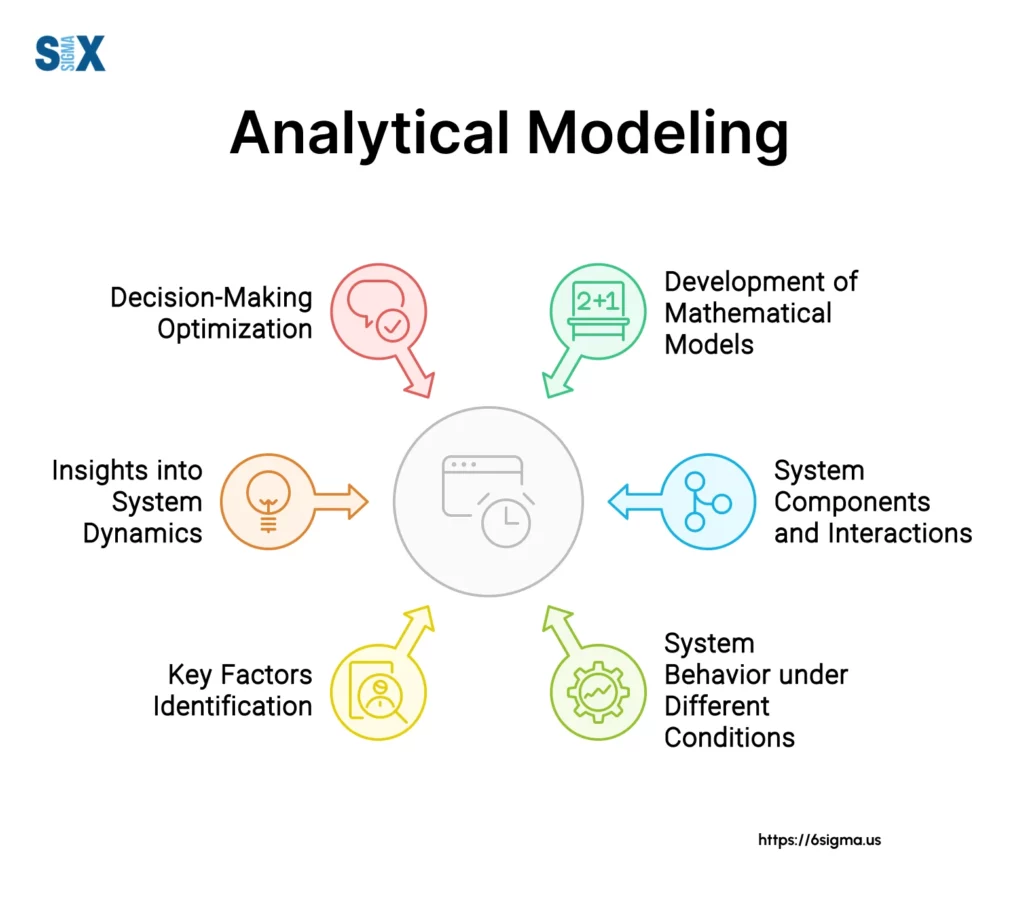
It is a quantitative and computational framework that allows organizations to dissect intricate systems, identify patterns, and understand the relationships between various variables and factors influencing specific outcomes.
Importance in data-driven decision making
Analytical modeling has emerged as an indispensable tool for organizations seeking to gain a competitive edge.
By leveraging advanced analytical techniques, businesses can unlock insights hidden within complex data sets, accurately define problems, formulate solutions, and anticipate outcomes based on empirical evidence rather than intuition or guesswork.
It promotes data-driven decision-making, enabling leaders to base their strategies and actions on quantitative analysis rather than relying solely on subjective theories or personal experiences.
This data-driven approach has proven invaluable in driving continuous improvement, optimizing processes, and achieving better outcomes across various industries.
Applications of Analytical Modeling across industries
The applications of analytical modeling span a wide array of industries and domains, including but not limited to:
- Business intelligence: Analytical modeling plays a crucial role in business intelligence, enabling organizations to extract valuable insights from data and make informed decisions related to operations, marketing, finance, and strategic planning.
- Operations research: This field heavily relies on analytical modeling techniques to optimize processes, allocate resources efficiently, and streamline supply chain operations.
- Finance: Financial institutions leverage it for risk assessment, portfolio management, credit scoring, and fraud detection.
- Marketing: Marketing professionals use it for customer segmentation, targeted advertising, and predicting consumer behavior.
- Healthcare: In the healthcare industry, it is employed for disease prediction, patient risk stratification, and optimizing resource allocation in healthcare facilities.
- Manufacturing: Analytical modeling techniques are instrumental in manufacturing settings’ quality control, process optimization, and predictive maintenance.
These are just a few examples of the vast applications of analytical modeling, which continue to expand as organizations increasingly recognize the value of data-driven decision-making.
Key Concepts and Techniques of Analytical Modeling
Take a look at the key concepts and techniques in analytical modeling.
Mathematical Models
Regression analysis
Regression analysis is a fundamental technique that aims to establish relationships between dependent and independent variables.
By analyzing historical data, regression models can identify patterns and quantify the impact of various factors on a particular outcome.
Common applications of regression analysis include demand forecasting, pricing optimization, and credit risk assessment.
Optimization techniques
Optimization techniques are employed in analytical modeling to find the best possible solution or combination of variables that maximize or minimize a specific objective function, subject to certain constraints.
These techniques are widely used in resource allocation, scheduling, portfolio optimization, and supply chain management.
Linear programming, integer programming, and nonlinear programming are examples of optimization techniques that can be applied to different problem domains.
Simulation modeling
Simulation modeling involves creating a virtual representation of a system or process to study its behavior under various conditions.
By incorporating relevant variables and constraints, organizations can experiment with different scenarios and assess the potential impact of decisions before implementing them in the real world.
This approach is particularly valuable in complex environments where analytical solutions are challenging to derive.
Monte Carlo simulation and discrete-event simulation are two commonly used simulation modeling techniques in fields such as risk analysis, process improvement, and logistics management.
Statistical Algorithms
Machine learning
Machine learning algorithms are a powerful component, enabling systems to learn from data and make predictions or decisions without being explicitly programmed.
These algorithms can identify patterns, classify data, and adapt to changing conditions, making them invaluable in areas such as predictive maintenance, fraud detection, and recommendation systems.
Supervised learning, unsupervised learning, and reinforcement learning are the three main types of machine learning algorithms used in analytical modeling.
Predictive analytics
Predictive analytics is a branch of analytical modeling that focuses on forecasting future outcomes or behaviors based on historical data and known variables.
Forecasting models
Forecasting models are a crucial component of analytical modeling, aimed at predicting future trends or events based on past data and identified patterns.
Time series analysis, exponential smoothing, and autoregressive integrated moving average (ARIMA) models are commonly used for forecasting applications in areas such as sales planning, inventory management, and resource allocation.
Data Analysis with Analytical Modeling
Descriptive analytics
Descriptive analytics is the foundation of analytical modeling, involving the summarization and visualization of historical data.
This type of analysis answers the question “What happened?” by presenting key performance indicators (KPIs), dashboards, and reports that provide insights into past events and current states.
Diagnostic analytics
Building upon descriptive analytics, diagnostic analytics aims to uncover the underlying causes or drivers behind observed outcomes.
Prescriptive analytics
Prescriptive analytics represents the most advanced form of analytical modeling, combining insights from descriptive, diagnostic, and predictive analyses to recommend the best course of action for a given scenario.
Benefits of Analytical Modeling
Informed decision making
One of the primary benefits of analytical modeling is its ability to support informed decision-making.
By leveraging data-driven insights and quantitative analysis, organizations can accurately define problems, formulate solutions, and anticipate outcomes rather than relying solely on intuition or subjective theories.
Logical information structuring
Analytical modeling facilitates the logical structuring and organization of information.
By establishing mathematical relationships between variables and factors, analytical models enable businesses to trace general outcomes back to specific sources or root causes.
The collaborative and iterative approach
The objective nature of analytical modeling makes it an ideal tool for establishing a common foundation for discussion and collaboration within organizations.
Rather than attempting to reconcile subjective theories, analytical data provides a universal reference framework that can be iteratively refined and improved as new information becomes available.
Process optimization
Analytical modeling techniques, such as optimization modeling and simulation, can be leveraged to identify opportunities for process optimization.
By analyzing various scenarios and constraints, organizations can determine the most efficient allocation of resources, streamline workflows, and minimize waste or inefficiencies, ultimately leading to improved productivity and cost savings.
Risk assessment
Risk assessment is a critical application of analytical modeling, particularly in industries such as finance, healthcare, and manufacturing.
By analyzing historical data and incorporating relevant variables, analytical models can quantify and predict potential risks, enabling organizations to develop mitigation strategies and make informed decisions regarding risk management.
Customer segmentation
In marketing and customer relationship management, analytical modeling plays a vital role in customer segmentation.
By analyzing customer data, behavioral patterns, and demographic information, organizations can identify distinct customer segments and tailor their products, services, and marketing strategies to better meet the needs and preferences of each segment, leading to increased customer satisfaction and loyalty.
Case Studies
Business Analytics
Marketing Analytics
Marketing analytics leverages these techniques to gain insights into customer behavior, optimize marketing campaigns, and drive revenue growth.
By analyzing customer data, market trends, and campaign performance, organizations can develop targeted marketing strategies, predict customer churn, and optimize resource allocation for maximum impact.
Financial modeling
Financial institutions heavily rely on analytical modeling for various applications, including risk management, portfolio optimization, credit scoring, and fraud detection.
Quantitative models are employed to assess credit risk, optimize investment portfolios, detect fraudulent activities, and ensure compliance with regulatory requirements.
Supply chain analytics
In the realm of supply chain management, it plays a crucial role in optimizing operations, reducing costs, and improving efficiency.
By analyzing data related to inventory levels, demand patterns, transportation networks, and supplier performance, organizations can optimize inventory management, route planning, and supplier selection processes.
Industry-specific Examples
Healthcare analytics
The healthcare industry is leveraging analytical modeling to improve patient outcomes, optimize resource allocation, and enhance operational efficiency.
Predictive models are used for disease prediction, risk stratification, and identifying high-risk patients, enabling proactive interventions and personalized care plans.
Retail analytics
In the retail sector, analytical modeling techniques are employed for demand forecasting, inventory management, pricing optimization, and location analysis.
By analyzing sales data, customer behavior, and market trends, retailers can make informed decisions regarding product assortments, pricing strategies, and store locations, ultimately enhancing customer satisfaction and profitability.
IoT analytics
The Internet of Things (IoT) has generated vast amounts of data from connected devices and sensors.
Analytical modeling plays a crucial role in extracting insights from this data, enabling predictive maintenance, asset optimization, and real-time monitoring in various industries, such as manufacturing, energy, and transportation.
Best Practices and Challenges of Analytical Modeling
The accuracy and reliability of analytical models are highly dependent on the quality of the input data.
Garbage in, garbage out – poor data input will inevitably lead to subpar outputs and erroneous insights.
Therefore, ensuring the quality, completeness, and relevance of data is a critical prerequisite for successful analytical modeling.
Data cleaning and preparation
Data cleaning and preparation are essential steps in the analytical modeling process.
This involves identifying and addressing issues such as missing values, inconsistencies, outliers, and formatting errors.
Techniques like data imputation, normalization, and feature engineering may be employed to transform raw data into a suitable format for modeling.
Model Selection and Validation
Choosing appropriate models
Selecting the most appropriate analytical model is crucial for obtaining accurate and actionable insights.
Different models are designed for specific types of problems or data structures, and using an inappropriate model can lead to inaccurate results or suboptimal solutions.
Careful consideration of the problem domain, data characteristics, and modeling assumptions is necessary when choosing the most suitable analytical technique.
Cross-validation techniques
Cross-validation techniques, such as k-fold cross-validation and holdout validation, are essential for evaluating the performance and generalization capability of analytical models.
These techniques involve partitioning the available data into training and testing sets, enabling the assessment of model accuracy and preventing overfitting or underfitting issues.
Interpretation and Communication with Analytical Modeling
Data visualization
Effective data visualization is a critical component of analytical modeling, as it facilitates the interpretation and communication of complex insights.
By presenting data and model outputs in a clear and visually appealing manner, stakeholders can more easily understand patterns, trends, and relationships, enabling informed decision-making.
Communicating insights effectively
While analytical models can generate valuable insights, it is equally important to communicate these insights effectively to various stakeholders, including executives, managers, and domain experts.
Clear and concise communication, tailored to the audience’s level of technical expertise, is essential for ensuring that the insights derived from analytical modeling are understood and can be translated into actionable strategies.
Ethical Considerations
Bias and fairness in modeling
Analytical models can be influenced by biases present in the underlying data or introduced during the modeling process itself.
It is crucial to address potential sources of bias, such as sample selection bias, algorithmic bias, or human bias, to ensure fair and equitable outcomes.
Techniques like bias testing, model auditing, and ethical AI frameworks can help mitigate these risks.
Privacy and data security
As analytical modeling often involves sensitive and personal data, maintaining privacy and data security is of utmost importance.
Organizations must comply with relevant data protection regulations and implement robust security measures to safeguard sensitive information from unauthorized access, breaches, or misuse.
Techniques such as data anonymization, encryption, and access controls can help mitigate privacy and security risks.
Future Trends and Emerging Technologies
With the exponential growth of data generated from various sources, such as social media, sensors, and online platforms, big data modeling has emerged as a critical area of analytical modeling.
This field focuses on developing techniques and technologies to handle and extract insights from massive, complex, and diverse data sets.
Distributed computing frameworks, NoSQL databases, and advanced machine learning algorithms are enabling organizations to leverage big data for competitive advantage.
Conclusion
Analytical modeling has emerged as an indispensable tool for organizations seeking to gain a competitive edge.
By using advanced mathematical models, statistical algorithms, and data analysis techniques, businesses can accurately define problems, formulate solutions, and anticipate outcomes based on empirical evidence.
While the potential benefits are substantial, ensuring data quality, selecting appropriate models, validating results, communicating insights effectively, and addressing ethical considerations like bias and data privacy are crucial best practices.
By using analytical modeling as an integral part of their decision-making processes and staying up to date on the latest developments, organizations can unlock a competitive advantage and drive sustainable growth.
SixSigma.us offers both Live Virtual classes as well as Online Self-Paced training. Most option includes access to the same great Master Black Belt instructors that teach our World Class in-person sessions. Sign-up today!
Virtual Classroom Training Programs Self-Paced Online Training Programs