Manufacturing Process Simulation: Transforming the Lean Six Sigma Industry
The digital transformation of manufacturing has made process simulation a crucial tool for modern industries.
Manufacturing process simulation helps organizations test, validate, and optimize their production systems before implementing changes in the real world.
This technology enables manufacturers to reduce risks, cut costs, and improve efficiency while maintaining quality standards.
From small-scale operations to large manufacturing plants, simulation technology offers a practical approach to testing production scenarios without disrupting ongoing operations.
Key Highlights
- Understanding Simulation Basics and Benefits
- Integrating with Lean Six Sigma
- Modern Simulation Tools and Methods
- Implementation Strategies and Best Practices
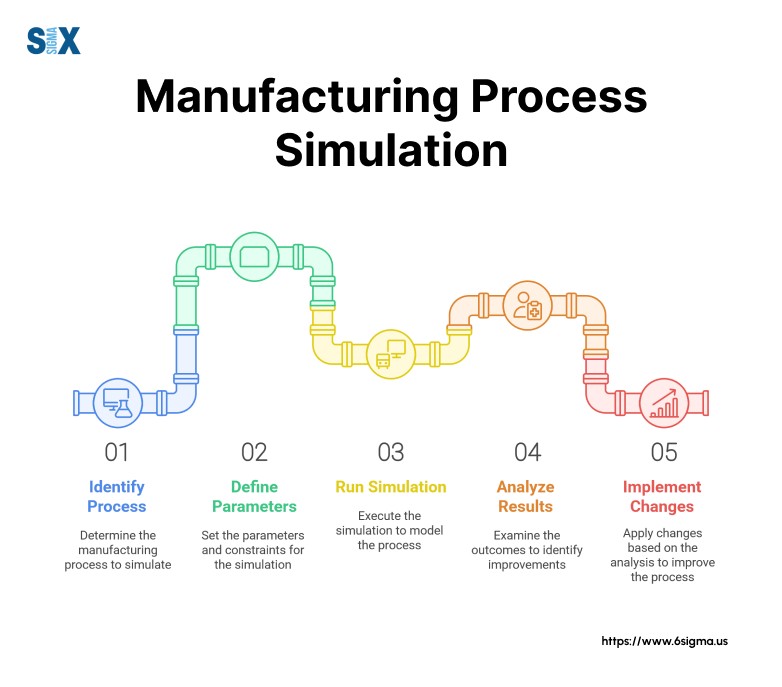
What Is Manufacturing Process Simulation?
Manufacturing process simulation creates virtual models of production systems to test and optimize operations before real-world implementation.
This digital approach allows manufacturers to analyze workflows, identify bottlenecks, and validate changes without disrupting active production lines.
Definition And Core Elements
The technology combines mathematical modeling with computer-aided design to replicate manufacturing environments.
These digital replicas factor in equipment specifications, material flow, worker interactions, and production schedules to create accurate representations of factory operations.
Modern simulation tools integrate real-time data and machine learning algorithms to enhance prediction accuracy and decision-making capabilities.
Types Of Manufacturing Process Simulation
Different simulation methods serve various manufacturing needs. Discrete event simulation tracks individual events through production systems, making it ideal for assembly lines and batch processing.
Continuous simulation models handle ongoing processes like chemical manufacturing or fluid dynamics.
Agent-based simulations focus on interactions between system components, particularly useful for complex automation scenarios.
Phases Of The Simulation Process
The simulation journey starts with data collection and system analysis. Engineers then build digital models, validate their accuracy, and run multiple scenarios.
The testing phase examines various production conditions and potential improvements. Finally, results analysis leads to implementation plans for validated changes.
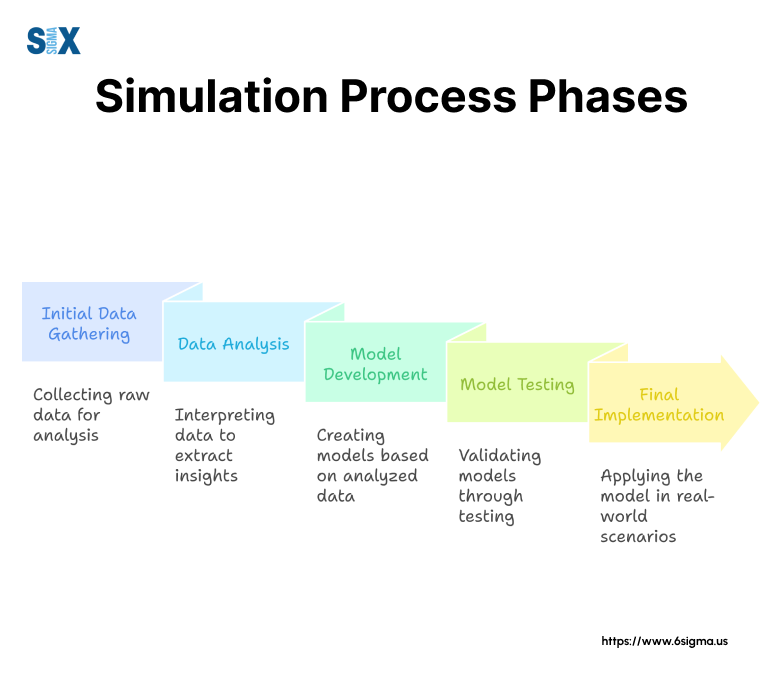
Purpose And Benefits In Manufacturing
Manufacturing simulation drives operational excellence through risk-free experimentation. It reduces equipment downtime by identifying maintenance needs before failures occur.
Production managers use simulation data to optimize resource allocation and minimize waste. The technology also supports training programs by providing safe environments for operator practice.
Key benefits include:
- 30-40% reduction in project planning time
- Significant decrease in implementation risks
- Enhanced quality control through virtual testing
- Improved resource utilization across production lines
Modern simulation platforms now incorporate digital twin capabilities, enabling real-time monitoring and predictive analytics.
This evolution supports the growing need for agile manufacturing and sustainable production practices.
Lean Six Sigma And Manufacturing Process Simulation
The integration of manufacturing process simulation with Lean Six Sigma methodologies creates powerful opportunities for process improvement.
Modern manufacturers use this combination to eliminate waste, reduce variations, and optimize production flows with greater precision than ever before.
Overview Of Lean Six Sigma Principles
Lean Six Sigma focuses on eliminating waste and reducing process variations. The methodology combines Lean’s efficiency principles with Six Sigma’s statistical quality control.
Manufacturing teams use these tools to streamline operations, minimize defects, and boost productivity while maintaining high-quality standards.
Role Of Manufacturing Process Simulation In Lean Six Sigma
Process simulation enhances Lean Six Sigma initiatives by providing data-driven insights before implementation.
Virtual testing environments allow teams to validate improvement ideas without disrupting current operations.
Manufacturing simulation tools help identify hidden inefficiencies and predict the impact of proposed changes.
Teams can test multiple scenarios simultaneously, measuring their effects on key performance indicators such as cycle time, throughput, and quality metrics.
Applications of Manufacturing Process Simulation In DMAIC Methodology
- Define: Simulation models map current processes and highlight problem areas
- Measure: Virtual environments capture performance data and process variations
- Analyze: Statistical analysis of simulation results reveals root causes
- Improve: Teams test and validate solutions in risk-free digital environments
- Control: Ongoing simulation monitoring ensures sustained improvements
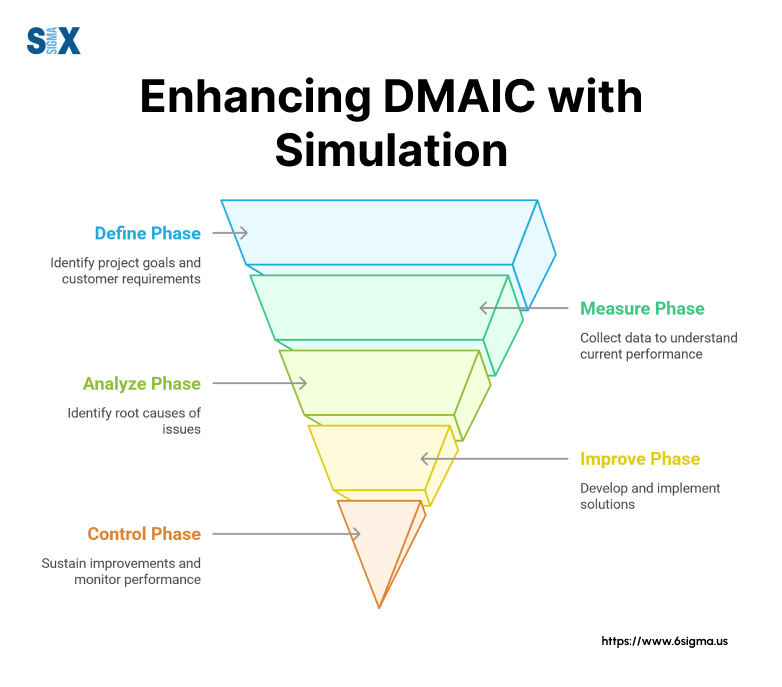
Case Study: Automotive Assembly Line Optimization
A leading automotive manufacturer used manufacturing process simulation to optimize their assembly line operations.
The project focused on reducing bottlenecks and improving throughput.
Through simulation testing, the team:
- Identified three major bottlenecks in the production line
- Tested five different line configurations virtually
- Implemented the optimal solution with 22% throughput improvement
- Achieved ROI within six months of implementation
The simulation-driven approach saved an estimated 40% in project time compared to traditional improvement methods.
It also prevented potential disruptions that could have occurred during physical testing of different configurations.
Achieve breakthrough improvements in your manufacturing processes with
DFSS Green Belt course to master simulation-driven design optimization.
Methodologies For Manufacturing Process Simulation
Modern manufacturing environments require specific simulation approaches based on their unique production needs.
Each methodology offers distinct advantages for different types of manufacturing processes, from discrete assembly operations to continuous flow production.
Discrete Event Simulation (DES)
DES excels in modeling sequential manufacturing operations where events occur at specific points in time.
This methodology tracks individual parts through production systems, making it ideal for assembly lines and batch processing operations.
DES helps manufacturers optimize queue management, resource allocation, and throughput rates by analyzing event-triggered changes in the system.
Agent-Based Modeling (ABM)
ABM focuses on the interactions between autonomous entities within manufacturing systems.
This approach models individual components, workers, and machines as agents with defined behaviors and decision-making capabilities.
The methodology proves particularly effective for complex automation scenarios and flexible manufacturing systems where multiple entities interact simultaneously.
System Dynamics (SD)
System Dynamics addresses the continuous aspects of manufacturing processes, emphasizing feedback loops and time-delayed relationships.
This methodology helps manufacturers understand long-term behavior patterns and strategic implications of process changes.
SD proves valuable for capacity planning, supply chain optimization, and resource management across extended time horizons.
Comparison Of Methodologies
Each simulation methodology serves specific manufacturing needs:
DES suits discrete manufacturing with clear event sequences and queuing systems. It provides detailed operational insights and helps optimize short-term process flows.
ABM excels in flexible manufacturing environments where individual component decisions impact overall system performance. It offers superior insights into emergent behaviors and complex interactions.
SD benefits strategic planning and policy analysis in manufacturing operations. It helps decision-makers understand long-term implications of process changes and system-wide impacts.
Manufacturers often combine these methodologies to create hybrid solutions that address multiple aspects of their operations.
The simulation of manufacturing processes continues to evolve with new technologies, incorporating AI and machine learning capabilities for enhanced accuracy and predictive power.
Enroll in our Green Belt course to master data-driven decision making.
Software Tools For Manufacturing Process Simulation
The right simulation software can dramatically impact manufacturing efficiency and decision-making quality.
Modern tools offer sophisticated capabilities while maintaining user-friendly interfaces, making advanced simulation accessible to manufacturing teams of all sizes.
Overview Of Popular Simulation Software
Leading manufacturing process simulation software includes Arena, FlexSim, and Simio, each offering distinct advantages.
Arena excels in discrete event simulation with robust statistical analysis capabilities. FlexSim provides powerful 3D visualization features for realistic process modeling.
Simio combines object-oriented modeling with advanced analytics for complex manufacturing environments.
Comparison Of Features And Capabilities
Modern simulation software varies significantly in their core strengths.
Arena offers extensive statistical libraries and detailed reporting functions, making it ideal for data-driven decision-making.
FlexSim’s 3D capabilities enable realistic virtual factory layouts and animated process flows. Simio’s object-oriented approach simplifies model building while maintaining flexibility for complex systems.
Key differentiating features include:
- Arena: Statistical analysis, optimization modules, industry-specific templates
- FlexSim: Real-time 3D visualization, drag-and-drop modeling, VR support
- Simio: Object-oriented architecture, risk analysis tools, cloud integration
Integration of Manufacturing Process Simulation With Lean Six Sigma Tools
Manufacturing process simulation software now seamlessly integrates with popular Lean Six Sigma tools.
These integrations enable:
- Value Stream Mapping: Digital mapping capabilities for current and future states
- Statistical Process Control: Real-time monitoring and analysis of process variations
- Root Cause Analysis: Data collection and visualization for problem-solving
- Continuous Improvement: Scenario testing for proposed process changes
The latest software releases emphasize cloud connectivity and digital twin capabilities.
These features allow teams to access simulation models remotely and integrate real-time production data.
Mobile applications extend accessibility, enabling managers to monitor simulations and make decisions from anywhere.
When selecting simulation software, manufacturers should consider:
- Specific process requirements
- Team technical expertise
- Integration needs with existing systems
- Budget constraints and ROI expectations
Implementing Manufacturing Process Simulation In Lean Six Sigma Projects
Successful implementation of manufacturing process simulation requires careful planning and systematic execution.
When integrated with Lean Six Sigma initiatives, simulation tools can significantly accelerate process improvements and validate changes before deployment.
Step-By-Step Implementation Guide
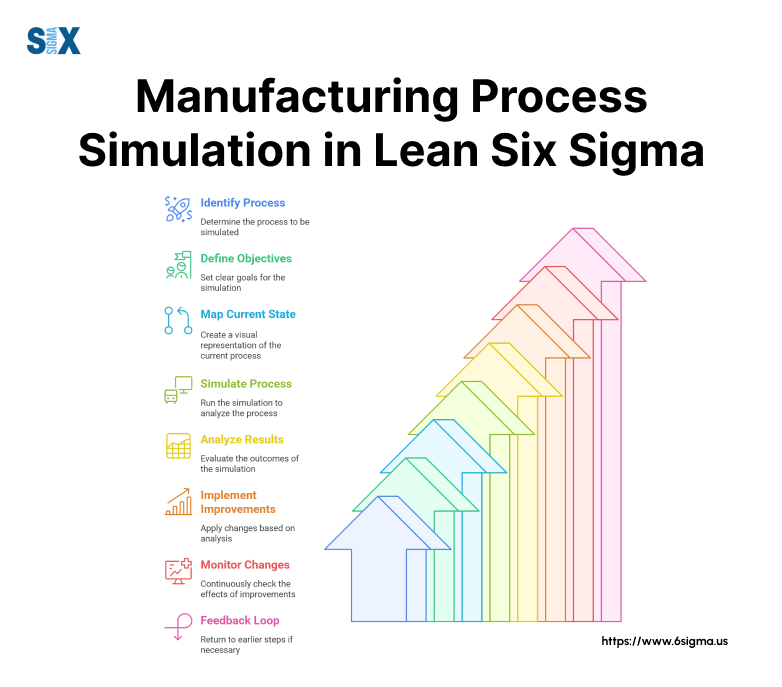
The implementation process starts with defining clear project objectives and scope. Teams must gather accurate data about current processes, including cycle times, resource requirements, and quality metrics.
Next, create baseline models that reflect existing operations. After validation, teams can begin testing improvement scenarios and measuring potential impacts.
Model validation requires comparing simulation results with actual process data. Once validated, teams can experiment with different scenarios to identify optimal solutions.
The final step involves documenting findings and creating implementation plans for approved changes.
Best Practices And Common Pitfalls of Manufacturing Process Simulation
Successful implementations rely on accurate data collection and stakeholder engagement. Teams should start with smaller projects to build confidence and expertise.
Regular validation ensures model accuracy throughout the project lifecycle.
Common pitfalls include insufficient data quality, oversimplified models, and lack of stakeholder buy-in.
Teams often struggle with scope creep or try to model too many variables simultaneously. Setting realistic expectations and maintaining clear communication helps avoid these issues.
ROI Calculation Framework
ROI calculations should consider both direct and indirect benefits. Direct savings include reduced cycle times, decreased waste, and improved resource utilization.
Indirect benefits encompass reduced risk, faster implementation, and improved decision-making capability.
The framework should track:
- Initial software and training costs
- Implementation time and resources
- Projected operational improvements
- Risk reduction values
- Long-term maintenance requirements
Industry-Specific Applications And Examples
Automotive manufacturers use simulation software to optimize assembly line configurations, reducing setup times by 30%.
Electronics manufacturers employ simulation tools to balance production lines and reduce WIP inventory. Food processing plants utilize simulation models to improve throughput while maintaining quality standards.
Recent implementations show:
- Aerospace: 25% reduction in production bottlenecks
- Pharmaceuticals: 40% improvement in batch scheduling
- Consumer goods: 20% decrease in changeover times
Modern simulation software manufacturing platforms now incorporate AI capabilities for enhanced prediction accuracy.
These tools integrate with existing manufacturing execution systems, providing real-time data for continuous model updates and refinement.
Conclusion
Manufacturing process simulation has evolved from a specialized tool into an essential component of modern manufacturing operations.
The integration with Lean Six Sigma methodologies creates powerful opportunities for process optimization, risk reduction, and sustainable growth.
The future of manufacturing simulation points toward increased AI integration and cloud-based solutions.
Digital twin technology continues to bridge the gap between virtual models and physical operations, while advanced analytics provide deeper insights into process improvements.
These developments make simulation more accessible and valuable for manufacturers of all sizes.
Organizations looking to stay competitive must embrace these technological advances. Starting with small-scale projects and gradually expanding simulation capabilities allows teams to build expertise while delivering measurable results.
The time to implement manufacturing process simulation is now, as early adopters gain significant advantages in efficiency, quality, and market responsiveness.
SixSigma.us offers both Live Virtual classes as well as Online Self-Paced training. Most option includes access to the same great Master Black Belt instructors that teach our World Class in-person sessions. Sign-up today!
Virtual Classroom Training Programs Self-Paced Online Training Programs