Cause and Effect Matrix: Complete Guide with Examples and Tips
A cause and effect matrix, also known as an X-Y diagram, prioritization matrix, or correlation matrix, is a versatile Six Sigma tool used to analyze the relationship between inputs and outputs in a process.
By mapping and scoring the connections between causes (process inputs) and effects (process results), teams can identify the most critical variables to focus on for process improvements.
The key objective of developing a cause-and-effect matrix is to pinpoint the vital few input factors from a longer list that have an outsized influence on outputs.
This data-driven approach provides an objective, quantified view of the variables rather than relying just on gut feelings or opinions.
Constructing a detailed cause-and-effect matrix does require some upfront effort – gathering the voice of customer inputs, mapping the process flow, and carefully evaluating the input-output correlations.
However, the payoff is an actionable priority list of root causes to address that can lead to major leaps in quality, capability, yield, or other critical outcomes.
What is a Cause and Effect Matrix?
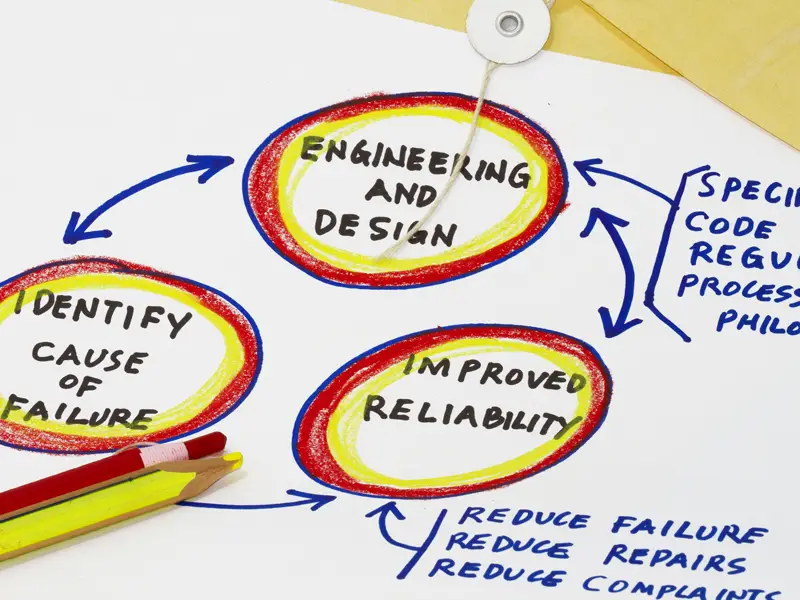
A cause and effect matrix, also known as an X-Y diagram or prioritization matrix, is a Lean Six Sigma tool used to prioritize key process input variables (KPIVs) based on the importance of customer outputs (KPOVs). It helps establish correlations between process inputs and customer outputs to guide root cause analysis and process improvement efforts.
The key inputs are plotted against process results to identify which variables have the greatest influence on performance or outcomes.
Specifically, a cause and effect matrix allows teams to quantify and rank correlations between many potential root causes, called input variables or process enablers, versus outcomes of a process, known as output variables or process results.
By cross-multiplying the subjective importance rating given to output metrics by the strength of impact relationships, an objective priority score helps zero in on the vital inputs.
More About Cause and Effect Matrix
Cause and effect matrices are also referred to by several other common names:
- X-Y Diagram
- Prioritization Matrix
- Correlation Matrix
The X-Y diagram name comes from plotting inputs (X’s) against outputs (Y’s) on a chart. It is also used for prioritization based on the ratings and serves as a visual representation of variable correlations.
Why Use the Cause and Effect Matrix?
The overarching purpose of developing a cause-and-effect matrix is to identify the key process input variables (KPIVs) based on the priorities of customer requirements or process outputs (KPOVs).
The key inputs filter down from a longer list of potential factors that may be influencing the performance metrics. This aligns improvement or optimization efforts on the elements that matter most.
Fundamentally, a cause-and-effect matrix brings together customer priorities, process knowledge, and team insights to determine critical inputs objectively.
The quantified relationships guide data-driven decision-making on where to focus.
When to Use a Cause and Effect Matrix
Cause and effect matrices have broad applicability for understanding the drivers behind process performance and key outcomes.
They bring an analytical rigor to decisions on prioritizing improvement efforts.
Teams can leverage cause and effect matrices during various phases of Lean Six Sigma projects when needing to identify vital inputs behind defects, delays, variations, bottlenecks, or shortfalls in goals.
Root Cause Analysis
A common usage is during root cause analysis in the Measure and Analyze stages. By revealing the strong versus weak relationships between inputs and outputs, the analysis points to potential core issues behind problems.
The tool integrates well with cause and effect diagrams and 5 Whys for drilling down further.
Prioritization in DMAIC Projects
The Measure phase more generally involves the discovery of critical inputs and variables that influence metrics the project aims to improve.
Teams can apply cause and effect matrices to cut through the complex web of possible factors to hone in on the vital few using the KPOV and KPIV approaches.
DOE Experiments
In the Design of Experiments during Improve stages, the cause and effect matrix is valuable for screening designs to find the process variables and interactions that may be most impactful to test.
This aligns experimentation around the factors likely to lead to breakthroughs.
Matrix Diagrams and FMEAs
The outputs of a cause and effect matrix also provide the basis for constructing prioritized matrix diagrams and failure modes effects analysis (FMEA) in the Analyze phases.
The vital few inputs can be further analyzed for ways they might fail and the effects.
How to Create a Cause and Effect Matrix
While cause and effect matrices may seem complicated at first glance, the step-by-step process boils down to three main stages:
- Understanding customer needs and process results
- Detailing process inputs
- Evaluating and quantifying the input-output relationships
By methodically working through each element, teams develop an insightful data-backed view into the vital few drivers tied to top priorities and outcomes.
Identify Customer Requirements
The first step involves connecting to the voice of the customer. What are the key quantifiable metrics that matter most to customer satisfaction and loyalty?
Common examples include on-time delivery, first-pass yield, accuracy, durability, mean time between failures, price, or cycle time.
Assign Priority Scores to Outputs
With the critical customer needs and process results defined, rank their importance on a 1-10 scale. 10 indicates extremely important factors, with priority descending toward less crucial metrics.
This quantifies the relative weight or value of the business.
List Input Variables
Transition to detailing the process inputs, materials, settings equipment, and other potential variables that influence the outputs.
Brainstorm an exhaustive list of Xs across the different stages of the process under review.
Assess Variable Relationships
Here teams review each input versus output combination and evaluate the degree of correlation based on process knowledge and capacity analysis.
Use a 0-1-3-9 scale to assign a rating, with 0 meaning no relationship and 9 indicating a very strong, direct relationship between the X and Y.
Calculate Correlation Score
Multiply the importance factor of each output by the relationship ratings identified, then sum the scores for each input variable.
This leads to composite scores that reveal the weighted impact of each X on overall performance.
Rank Inputs
Finally, order the list by the correlation scores, with the highest at the top. The vital few KPIVs emerge that drive KPOVs. Teams can proceed to optimize these factors.
Cause and Effect Matrix Example
To illustrate how a cause-and-effect matrix works in practice, let’s walk through an example applied to improving customer satisfaction ratings for the coffee served at a truck stop chain.
Business Case Background
The XYZ Truck Stop Chain has been struggling with declining customer satisfaction scores around the coffee offering over the past several quarters.
Complaints mention the coffee sometimes tastes “burnt” or “bitter.” The process owner assembled a project team to investigate the root causes and improve taste consistency.
Outputs and Priorities
The team first identified three key coffee-related metrics for customers using surveys and transaction data:
- Taste: Priority Score 10
- Temperature: Priority Score 8
- Time to Serve: Priority Score 5
Process Steps and Inputs
Next, they mapped out the high-level process steps involved in serving coffee to customers:
- Fill the Coffee Carafe with Water
- Insert Coffee Grounds Filter
- Set Brewing Temperature
- Dispense Coffee
- Add Cream/Sugar
Correlation Scoring
At each step, the team listed input variables that potentially influence the taste, temperature, and time outputs. They then scored the correlation strength for each input-output relationship from 0-9.
For example, the grind consistency, brewing time, and water quality were believed to have a very strong impact on taste (score of 9), while the location of the creamer had no influence (0).
Results and Analysis
Tabulating the scores revealed the top three vital inputs were grind consistency, brewing temperature, and water quality – which became the focus areas driving customer satisfaction.
Benefits of Using a Cause and Effect Matrix
There are several valuable reasons why teams invest the time to develop cause and effect matrices during continuous improvement initiatives:
Includes Customer Perspective
The very first step focuses attention on the vital few metrics that directly impact customer satisfaction and priorities as the lens for the rest of the analysis. This aligns improvements tightly with customer needs.
Visualizes Variable Relationships
The formatted grid structure provides a visual heat map of the higher versus lower correlation areas guiding discussion and shared understanding. Patterns emerge indicating relationships.
Provides Objective Prioritization
Rather than relying just on hunches or intuition, the numeric scoring introduces objectivity using the combined wisdom of the team and process insights. Vital few bubble up mathematically.
Focuses Data Collection
By revealing the critical Xs, the analysis prescribes where to concentrate measurement system analysis and data acquisition. This efficiently aligns with Six Sigma efforts.
Identifies Critical Inputs
The result answers the fundamental question – what are the key process input variables needing priority attention and control to substantially influence the outputs? Action-oriented.
Limitations of Cause and Effect Matrices
While using cause and effect matrices has many benefits, teams should also be aware of inherent limitations and misapplications of the tool:
Subject to Expert Bias
The relationship scoring relies heavily on subject matter expertise and process familiarity.
Unknown interactions or unintended consequences may lead to underestimating critical variables while over-weighting trivial ones.
Bias can skew outcomes.
Static View of Process
The analysis provides a snapshot reflecting current knowledge and assumptions. But processes continue to evolve dynamically.
Without updating, cause and effect matrices grow stale and findings show diminishing returns over time.
Other limitations include a tendency toward paralysis by analysis if the diagrams become extremely intricate mapping out all subtleties.
This can slow down improvements waiting for perfect information.
Additionally, while identifying vital inputs, the tool does not provide solutions or answers on how exactly to optimize them without additional analysis methods or experiments.
By being cognizant of these constraints, teams can maximize benefits while proactively addressing challenges that may emerge when overly relying on cause-and-effect matrices alone to guide decisions.
Revalidating with DOE tests and inspecting suspected interactions uncovers hidden drivers over time.
Alternatives and Variations
While cause and effect matrix serves as the most common name for this analytical methodology, there are a few other labels and closely related tools that accomplish similar prioritization objectives:
X-Y Diagram
The X-Y diagram variant emphasizes the visualization of plotting multiple X input variables against Y output variables for analysis. Same construction steps otherwise.
Prioritization Matrix
A prioritization matrix is primarily focused on risk assessments of various factors, scoring probability and severity or impact to rank scenarios. Can be tailored for process inputs as well but oriented differently.
Correlation Matrix
Correlation matrices indicate the linear statistical relationships bonding variables across large multivariate data sets.
These matrices contain correlation coefficients rather than the subjective ratings used in cause-and-effect approaches.
In most forms, the core framework surrounds connecting multiple influencing X factors to multiple Y performance metrics in a systematic way.
This provides an evidence-based rationale for driving process improvements.
Implementation Tips and Best Practices
A cause and effect matrix, also referred to as an X-Y diagram or prioritization matrix, is a Lean Six Sigma tool used to analyze the relationship between inputs and outputs of a process.
Like any analytical tool, perfecting the technique and application of cause and effect matrices takes some finesse. Following best practices will lead to optimal results:
Careful Scoring
Take the time to carefully consider each input-output relationship, leveraging benchmarks and scientific mechanisms where possible.
Avoid defaulting to moderate scores without backing. Distinguish strong vs weak ties.
Review with Multiple Experts
Prevent individual bias by reviewing the full matrix with several subject matter experts and reconciling outlying perspectives.
Surfacing healthy debate flushes out deeper insights.
Update with New Data
Run through the scoring process again after any major changes, new metrics, or process learnings. This keeps the matrix dynamic rather than stale by incorporating the latest findings.
Additionally, start manageable in scope when first getting familiar with constructing cause and effect matrices.
While they can get extremely complex mapping an entire end-to-end value chain, limiting to 3-5 key outputs and 10-15 inputs makes it practical. Build out from there once the methodology is clear.
SixSigma.us offers both Live Virtual classes as well as Online Self-Paced training. Most option includes access to the same great Master Black Belt instructors that teach our World Class in-person sessions. Sign-up today!
Virtual Classroom Training Programs Self-Paced Online Training Programs