Predictive Quality Analytics in Lean Six Sigma Manufacturing
Manufacturing organizations worldwide are shifting from reactive quality control to data-driven predictive approaches.
Predictive quality analytics transforms traditional quality management by combining advanced algorithms, real-time data analysis, and machine learning capabilities to detect and prevent quality issues before they occur.
In the Lean Six Sigma framework, predictive quality analytics serves as a powerful tool for reducing defects, minimizing waste, and optimizing production processes.
This technology enables quality engineers and production managers to make informed decisions based on data patterns rather than gut feelings or historical trends alone.
Key Highlights
- Predictive Analytics in Quality Management
- Implementation Steps for Manufacturing Success
- Data-Driven Quality Control Methods
- Applications and Results
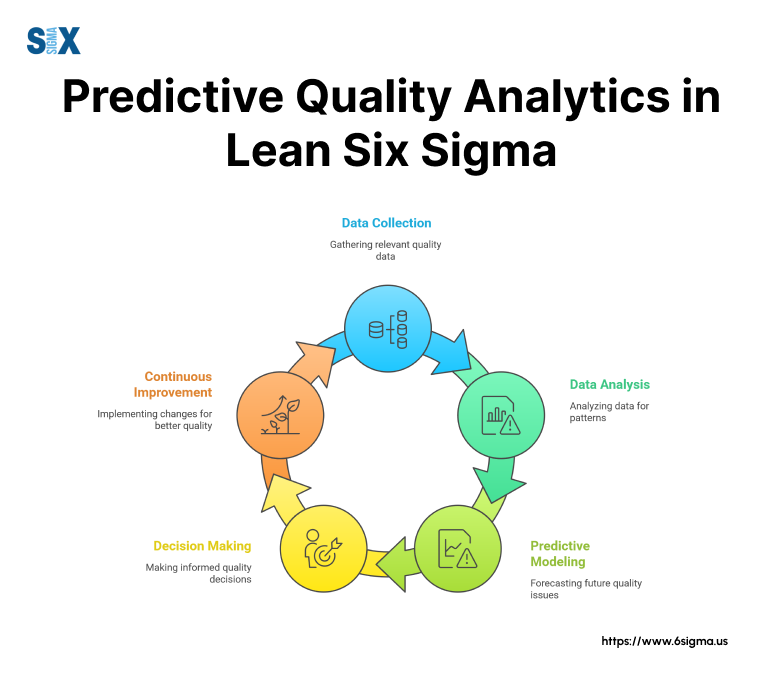
What Is Predictive Analytics In Quality Management?
Predictive quality analytics transforms manufacturing data into actionable insights that prevent quality issues before they occur.
This technology uses statistical analysis, machine learning algorithms, and real-time data monitoring to identify patterns that signal potential defects or process deviations.
The Foundation of Predictive Analytics in Manufacturing
Manufacturing facilities generate massive amounts of data through sensors, quality checks, and production metrics.
Predictive analytics tools process this information to forecast quality outcomes and identify risk factors that might affect product quality.
These forecasts enable quality teams to move from reactive problem-solving to proactive quality management.
Quality Management Through Data-Driven Insights
In the quality management context, predictive analytics combines historical data with real-time monitoring to:
- Detect anomalies in production processes
- Forecast maintenance needs before equipment failure
- Identify optimal process parameters
- Reduce quality-related costs through early intervention
Essential Components of Predictive Quality Systems
Modern predictive quality analytics systems rely on several key elements:
Data Collection Infrastructure: Sensors and IoT devices that gather real-time production data
Analysis Engines: Advanced algorithms that process and analyze collected data
Visualization Tools: Dashboards and reports that present insights to decision-makers
Integration Capabilities: Systems that connect with existing quality management tools
Alignment With Lean Six Sigma Principles
Predictive quality analytics enhances Lean Six Sigma methodologies by providing data-driven support for:
- Define: Identifying critical quality parameters through data analysis
- Measure: Capturing accurate, real-time process measurements
- Analyze: Uncovering hidden patterns and relationships in quality data
- Improve: Implementing targeted improvements based on predictive insights
- Control: Maintaining process stability through continuous monitoring
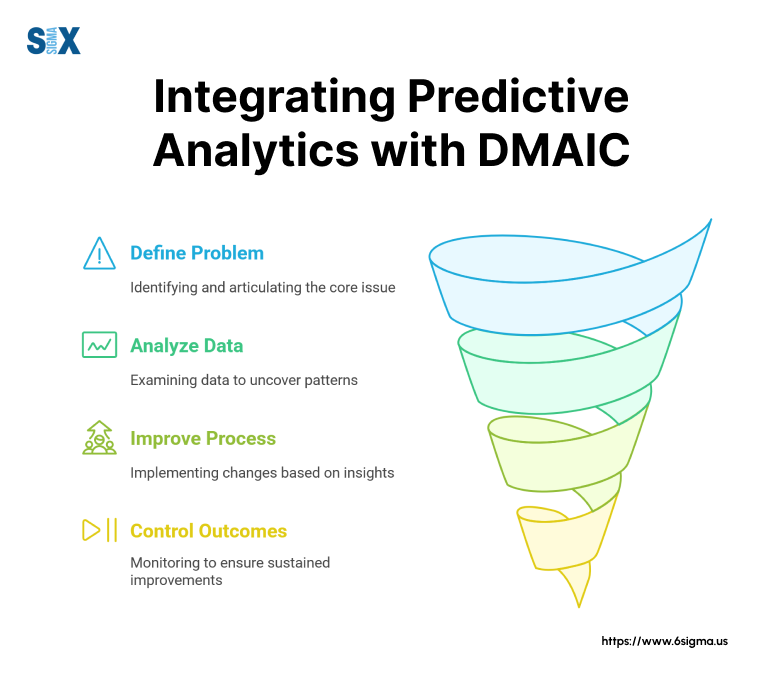
Quality engineers and production managers use these predictive insights to optimize processes, reduce waste, and maintain consistent product quality.
The technology supports both strategic planning and daily operational decisions, making it valuable across all levels of manufacturing organizations.
The Role of Machine Learning in Quality Prediction
Machine learning algorithms analyze historical quality data to create models that predict future outcomes.
These models become more accurate over time as they process more data, enabling increasingly precise quality predictions.
This self-improving capability makes predictive analytics particularly valuable for complex manufacturing processes where traditional quality control methods might fall short.
Master the integration of predictive analytics with Six Sigma methodologies. Join our Champion Leadership Program to lead data-driven quality improvements.
The Role of Predictive Quality Analytics in Lean Six Sigma
Predictive quality analytics revolutionizes Lean Six Sigma implementations by adding powerful data analysis capabilities to traditional quality improvement methods.
This technology strengthens each phase of the DMAIC methodology while providing real-time insights for faster, more accurate decision-making.
Enhancing The DMAIC Methodology
The integration of predictive analytics transforms each DMAIC phase:
Define: Statistical models identify critical quality characteristics and process variables that impact product quality. This data-driven approach helps teams focus on the most significant improvement opportunities.
Measure: Automated data collection and analysis tools provide accurate, real-time measurements of process performance. These tools eliminate manual data entry errors and enable continuous monitoring of key metrics.
Analyze: Advanced algorithms uncover hidden patterns and relationships between process variables. Quality teams can quickly identify root causes and predict potential quality issues before they occur.
Improve: Simulation models test proposed solutions virtually before implementation, reducing risk and resource waste. Teams can optimize process parameters based on predictive models rather than trial and error.
Control: Real-time monitoring systems maintain process stability through early detection of deviations. Automated alerts notify teams when processes drift toward unacceptable limits.
Process Control Through Predictive Insights
Modern manufacturing facilities use predictive quality analytics to maintain tighter process control.
The technology monitors multiple process parameters simultaneously, detecting subtle changes that might escape traditional control methods. This enhanced monitoring capability enables:
- Earlier detection of process drift
- More precise control limits
- Reduced variation in product quality
- Lower inspection costs
Data-Driven Decision Making In Manufacturing
Quality engineers and production managers leverage predictive analytics software to make informed decisions about:
Process Adjustments: Real-time data analysis guides parameter adjustments to maintain optimal quality levels.
Resource Allocation: Predictive models help teams focus resources on areas with the highest risk of quality issues.
Maintenance Scheduling: Analytics tools forecast equipment maintenance needs to prevent quality problems caused by machinery wear.
Supporting Continuous Improvement Initiatives
Predictive quality analytics drives continuous improvement by providing:
- Trend Analysis: Long-term data patterns reveal opportunities for process optimization.
- Performance Metrics: Real-time quality indicators track improvement progress.
- Risk Assessment: Predictive models identify potential quality risks before they impact production.
- ROI Tracking: Analytics tools measure the financial impact of quality improvements.
- The integration of predictive analytics with Lean Six Sigma methodologies creates a more robust quality management system.
This combination enables manufacturing organizations to achieve higher quality levels while reducing costs and improving efficiency.
Future-Proofing Quality Management
As manufacturing technologies advance, predictive quality analytics will play an increasingly crucial role in Lean Six Sigma implementations.
The technology continues to evolve, offering new capabilities for:
- Advanced pattern recognition
- Automated root cause analysis
- Real-time process optimization
- Predictive maintenance scheduling
Elevate your DMAIC expertise with advanced predictive analytics capabilities. Enroll in our Champion Program to master quality-driven leadership
Implementing Predictive Quality Analytics in Manufacturing
Successful implementation of predictive quality analytics requires careful planning, proper infrastructure, and strategic execution.
Manufacturing organizations must follow a structured approach to ensure maximum return on their analytics investment.
Implementation Roadmap For Manufacturing Success
Phase 1: Assessment and Planning
Manufacturing facilities must evaluate their current quality management systems and identify specific areas where predictive analytics will deliver the most value.
This phase includes defining clear objectives, setting measurable goals, and securing stakeholder buy-in.
Phase 2: Infrastructure Development
Organizations need to establish the necessary technical foundation, including:
- Data collection systems
- Network infrastructure
- Storage solutions
- Processing capabilities
- Security protocols
Phase 3: Team Development
Quality teams require training on new tools and methodologies. This includes both technical training for data analysis and practical application in daily operations.
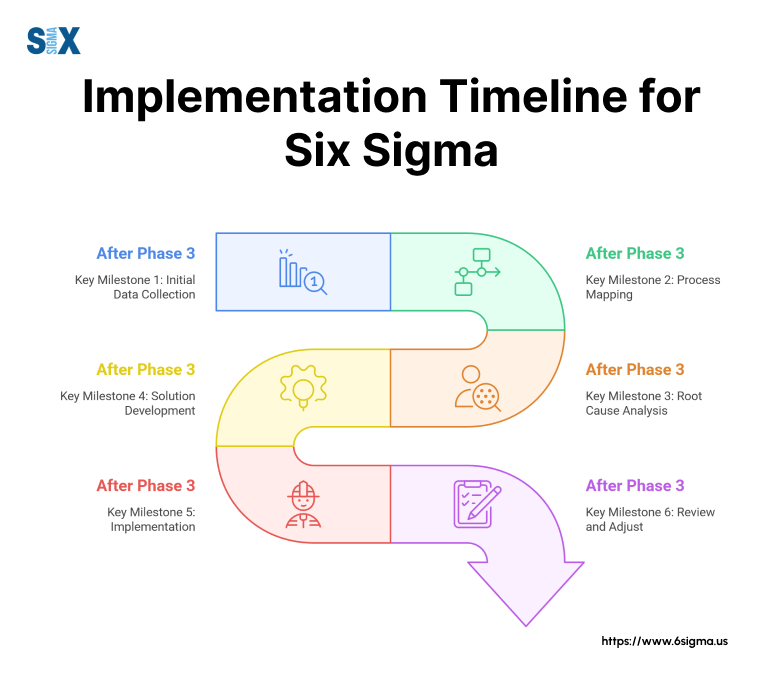
Data Requirements And Collection Methods
Quality data forms the foundation of predictive analytics success.
Manufacturing facilities must collect:
Production Data:
- Machine parameters
- Process variables
- Environmental conditions
- Production rates
Quality Data:
- Inspection results
- Test measurements
- Defect records
- Customer feedback
Operational Data:
- Maintenance records
- Operator inputs
- Equipment status
- Material tracking
Selecting The Right Predictive Analytics Tools
Manufacturing organizations should evaluate predictive analytics software based on:
Functionality Requirements:
The selected tool must align with specific quality management needs and support required analysis types.
Integration Capabilities:
Software should seamlessly connect with existing systems, including MES, ERP, and quality management platforms.
Scalability:
Tools must handle increasing data volumes and adapt to changing business needs.
User Interface:
The system should provide intuitive dashboards and reports accessible to various user levels.
Integration With Quality Management Systems
Predictive analytics tools must work alongside existing quality management systems.
Key integration considerations include:
Data Flow Management:
Establish automated data transfer between systems to ensure real-time analysis capabilities.
Process Alignment:
Align predictive analytics workflows with existing quality control procedures.
System Architecture:
Design an integrated system architecture that supports both current and future needs.
Security Protocols:
Implement robust security measures to protect sensitive quality and production data.
Measuring Implementation Success
Organizations should track specific metrics to evaluate their predictive analytics implementation:
Quality Metrics:
- Defect reduction rates
- First-pass yield improvements
- Scrap reduction
- Customer complaint decreases
Operational Metrics:
- Analysis speed improvements
- Prediction accuracy rates
- System utilization levels
- User adoption rates
Financial Metrics:
- Cost savings from prevented quality issues
- ROI on analytics implementation
- Reduced quality control costs
- Improved resource allocation
The successful implementation of predictive quality analytics requires ongoing monitoring and adjustment.
Organizations should regularly review their implementation progress and make necessary adjustments to maximize the value of their analytics investment.
Technical Components of Predictive Quality Analytics
The technical foundation of predictive quality analytics combines multiple advanced technologies to deliver accurate quality predictions and insights.
Understanding these components helps manufacturing teams select and implement the right solutions for their specific needs.
AI and Machine Learning in Quality Prediction
Modern predictive quality analytics systems employ various AI and ML algorithms to analyze manufacturing data.
These technologies enable:
- Pattern Recognition
- Anomaly Detection
- Predictive Modeling
[Suggested Image: Insert a flowchart showing how AI/ML processes manufacturing data to generate quality predictions, placed after this section]
Statistical Models and Analysis Techniques
Statistical analysis forms the backbone of predictive quality analytics.
Key statistical methods include:
- Regression Analysis: Models relationships between process variables and quality outcomes.
- Time Series Analysis: Tracks quality metrics over time to identify trends and seasonal patterns.
- Multivariate Analysis: Examines relationships between multiple variables simultaneously, enabling more accurate quality predictions.
Data Preprocessing Methods
Raw manufacturing data requires careful preparation before analysis. Essential preprocessing steps include:
- Data Cleaning: Removing errors, outliers, and inconsistencies from raw data to ensure accurate analysis.
- Data Normalization: Standardizing data formats and scales to enable meaningful comparisons across different variables.
- Feature Engineering: Creating new variables that better represent the underlying quality relationships in the data.
Analysis and Processing Tools
Manufacturing organizations use various tools to process and analyze quality data:
- ETL Tools: Extract, transform, and load data from multiple sources into analytics systems.
- Statistical Software: Process data using advanced statistical methods and algorithms.
- Machine Learning Platforms: Train and deploy predictive models for quality analysis.
Visualization and Reporting Systems
Effective data visualization helps teams understand and act on predictive insights:
- Real-Time Dashboards: Display current quality metrics and predictions in easy-to-understand formats.
- Interactive Reports: Allow users to explore data and generate custom analyses.
- Alert Systems: Notify relevant personnel when quality metrics approach critical thresholds.
Integration Architecture
The technical components must work together seamlessly through:
- Data Pipelines: Automated systems that collect and process data from multiple sources.
- APIs and Connectors: Enable communication between different systems and components.
- Storage Solutions: Handle large volumes of historical and real-time quality data.
- Security Systems: Protect sensitive quality data and ensure regulatory compliance.
Performance Monitoring and Optimization
Technical systems require continuous monitoring and adjustment:
- Model Performance: Track prediction accuracy and model drift over time.
- System Performance: Monitor processing speeds and resource utilization.
- Data Quality: Ensure ongoing data accuracy and completeness.
These technical components work together to create a robust predictive quality analytics system. Regular evaluation and updates ensure the system continues to deliver valuable insights for quality improvement.
Turn theory into practice with hands-on predictive analytics training. Join our Champion Program to master statistical design and process control
Applications and Examples of Predictive Quality Analytics in Manufacturing
Manufacturing organizations across various industries have successfully implemented predictive quality analytics to improve their operations.
These applications demonstrate the practical value and return on investment that organizations can achieve through proper implementation.
Industry-Specific Applications
Automotive Manufacturing:
Major automotive manufacturers use predictive quality analytics to monitor assembly line processes and prevent defects.
These systems analyze thousands of data points per vehicle, identifying potential quality issues before they impact final assembly.
Pharmaceutical Production:
FDA-regulated facilities employ predictive analytics to maintain strict quality standards.
These tools monitor critical process parameters and environmental conditions to ensure product safety and compliance.
Electronics Manufacturing:
Circuit board manufacturers utilize predictive quality systems to detect microscopic defects and maintain precise production parameters.
This application has reduced defect rates by up to 45% in several facilities.
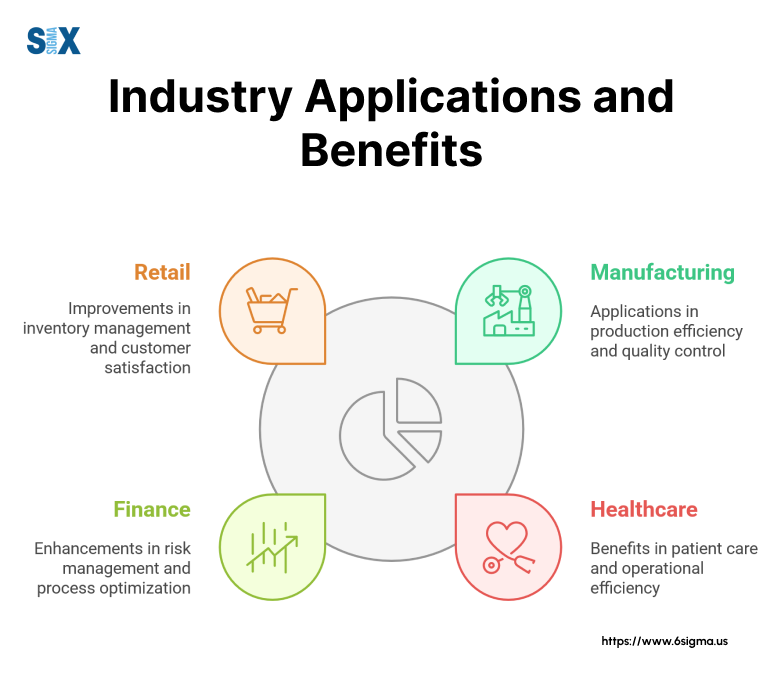
Solution Comparison and Selection
Enterprise-Level Solutions:
Designed for large manufacturing operations, these systems offer:
- Full integration capabilities
- Advanced analytics features
- Multi-site deployment options
- Customizable dashboards
Mid-Market Solutions:
Suited for medium-sized manufacturers, providing:
- Essential predictive capabilities
- Standard integration options
- User-friendly interfaces
- Reasonable cost structure
Industry-Specific Platforms:
Tailored for specific manufacturing sectors with:
- Pre-configured industry templates
- Relevant regulatory compliance features
- Sector-specific analytics models
Implementation Considerations
Resource Requirements:
Different solutions demand varying levels of:
- Technical expertise
- Infrastructure investment
- Training requirements
- Ongoing support needs
Integration Capabilities:
Organizations must evaluate:
- Compatibility with existing systems
- Data transfer capabilities
- API availability
- Scalability options
Cost Structures:
Solutions vary in:
- Initial investment requirements
- Ongoing maintenance costs
- Training expenses
- ROI timeframes
Future Trends and Developments
The predictive quality analytics field continues to evolve with:
- Enhanced AI capabilities
- Improved real-time processing
- Advanced visualization tools
- Greater automation features
Manufacturing organizations should consider these emerging capabilities when selecting and implementing predictive quality analytics solutions.
The Road Ahead
Predictive quality analytics transforms manufacturing quality management through data-driven insights and proactive problem prevention.
The integration of these tools with Lean Six Sigma methodologies creates powerful systems for quality improvement and cost reduction.
Manufacturing organizations that implement these solutions gain significant advantages in product quality, operational efficiency, and customer satisfaction.
Critical Success Factors
The successful implementation of predictive quality analytics depends on several key elements.
Organizations must ensure proper data collection and management systems, select appropriate analytics tools, and develop team capabilities.
The integration with existing quality management systems requires careful planning and execution to maximize value.
Quality teams that leverage predictive analytics tools see substantial improvements in their ability to prevent defects, optimize processes, and maintain consistent product quality.
These improvements translate directly to bottom-line benefits through reduced waste, lower quality costs, and improved customer satisfaction.
The Future of Manufacturing Quality
Manufacturing quality management continues to evolve with advancing technology. Future developments in predictive quality analytics will likely include:
Enhanced AI Capabilities:
More sophisticated algorithms will enable more accurate predictions and deeper insights into quality relationships.
Improved Integration:
Seamless connectivity between systems will create more efficient quality management workflows.
Advanced Automation:
Automated response systems will take immediate action based on predictive insights.
Real-Time Optimization:
Continuous process adjustment will maintain optimal quality levels automatically.
Taking Action for Quality Excellence
Manufacturing organizations should begin their predictive quality analytics journey by:
- Evaluating current quality management systems and identifying improvement opportunities
- Assessing data collection capabilities and infrastructure requirements
- Developing implementation plans that align with organizational goals
- Building team capabilities through training and development
- Selecting appropriate tools and technologies for their specific needs
The transition to predictive quality analytics requires commitment and resources, but the potential returns justify the investment.
Organizations that delay implementation risk falling behind competitors who leverage these powerful tools for quality improvement.
Manufacturing leaders should act now to explore predictive quality analytics solutions that fit their operations.
The technology continues to mature, offering increasingly sophisticated capabilities at more accessible price points. Early adopters gain competitive advantages while building the expertise needed for long-term success.
SixSigma.us offers both Live Virtual classes as well as Online Self-Paced training. Most option includes access to the same great Master Black Belt instructors that teach our World Class in-person sessions. Sign-up today!
Virtual Classroom Training Programs Self-Paced Online Training Programs