Attribute Sampling: Guide for Modern Auditors and Quality Control Professionals
The foundation of attribute sampling rests on binary outcomes – an item either possesses or lacks a specific characteristic.
For instance, when auditors examine purchase orders, they check if each document contains an authorized signature. The result is straightforward: the signature exists or it doesn’t.
This statistical approach differs from other sampling methods because it focuses on countable traits rather than measurements.
What This Guide Covers
- Statistical principles and methodologies
- Step-by-step process of implementing attribute sampling
- Practical applications across different industries
- Tools and techniques for analyzing and interpreting sampling results.
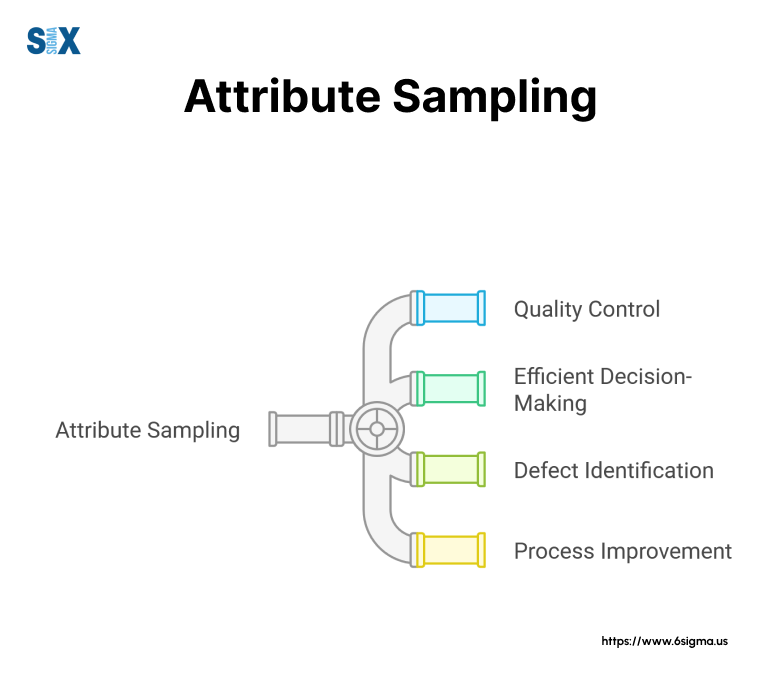
What is Attribute Sampling?
Attribute sampling represents a statistical method where inspectors examine specific characteristics in a sample to determine whether items meet predefined criteria.
This technique proves valuable when organizations need to evaluate yes/no or pass/fail scenarios.
For example, when auditors review purchase orders, they check if each document contains proper authorization signatures – either the signature exists, or it doesn’t.
Quality control teams often implement attribute sampling to monitor manufacturing processes.
They might examine a batch of products to verify if items conform to safety standards or contain specific defects.
The binary nature of these assessments – conforming versus non-conforming – makes attribute sampling particularly effective for quality assurance programs.
Essential Principles of Attribute Sampling That Drive Results
Several key principles govern effective attribute sampling processes.
The sample size must be statistically significant to represent the entire population accurately. Random selection ensures unbiased results.
The acceptance criteria need clear definition before sampling begins. These elements work together to produce reliable data for decision-making.
Statistical validity remains crucial in attribute sampling. Organizations must determine acceptable quality levels (AQL) and tolerable error rates before implementing sampling plans.
These parameters help establish the minimum sample size needed for meaningful results.
Attribute Vs. Variable Sampling: Key Differences
While attribute sampling focuses on presence/absence characteristics, variable sampling measures specific values on a continuous scale.
Consider a manufacturing example: attribute sampling would check if products meet size specifications (pass/fail), while variable sampling would measure the exact dimensions of each item.
The choice between these methods depends on several factors:
- Data type requirements
- Cost considerations
- Time constraints
- Required precision levels
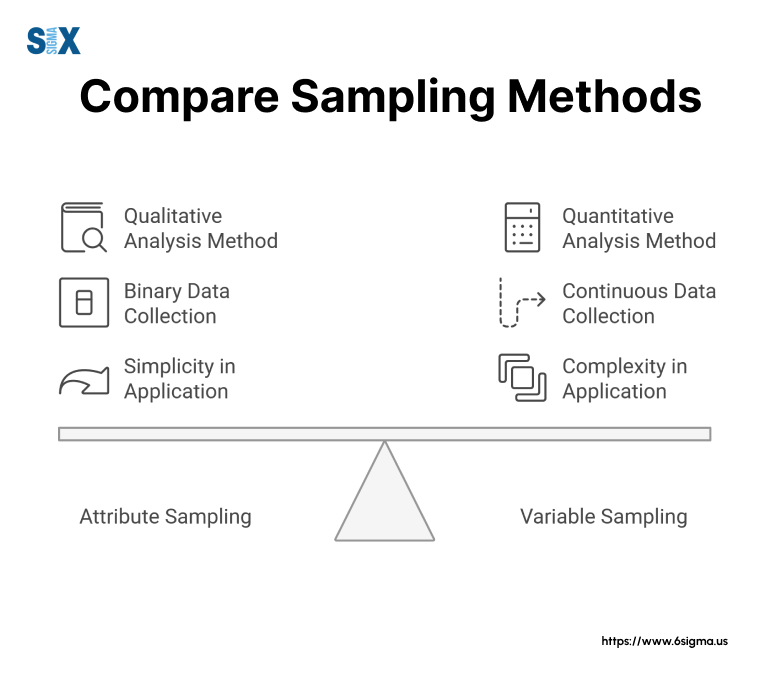
Types Of Attribute Data In Practice
Organizations encounter various forms of attribute data in their operations.
Defective/non-defective products represent the most common type in manufacturing.
In service industries, compliance/non-compliance with procedures forms another crucial category. Financial audits often examine correct/incorrect transaction processing.
Quality control managers might implement attribute sampling to check:
- Proper documentation in files
- Correct assembly of components
- Presence of required labels
- Adherence to safety protocols
Each type of attribute data requires specific sampling plans and acceptance criteria.
The sampling method must align with the organization’s quality objectives and risk tolerance levels.
Regular evaluation and adjustment of sampling plans ensure continued effectiveness in meeting quality control goals.
Through proper implementation of attribute sampling, organizations can maintain quality standards while optimizing resource allocation.
The next sections will explore practical applications and implementation strategies for various business contexts.
Master the statistical foundations of quality control through our Six Sigma Champion program
The Attribute Sampling Process: From Planning To Results
The attribute sampling process follows a structured approach that ensures statistical validity and reliable results.
Organizations must first define their objectives clearly, identifying specific characteristics they need to evaluate.
For example, a manufacturing facility might focus on product defects, while an accounting department examines invoice accuracy.
Quality managers then establish acceptance criteria and risk levels.
These parameters determine what constitutes acceptable quality and the confidence level required for decision-making. Setting these boundaries early prevents confusion during later evaluation stages.
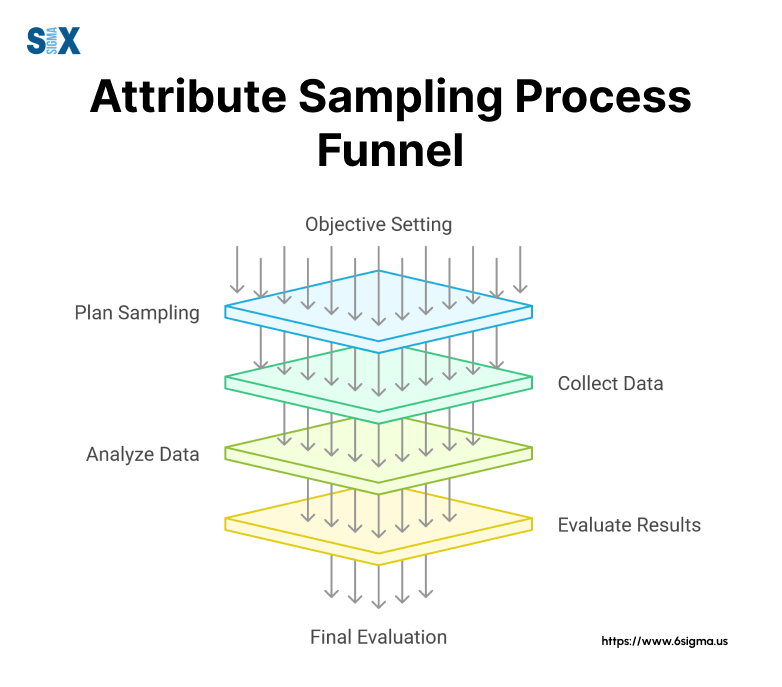
Calculating The Right Sample Size with Attribute Sampling
Determining the appropriate sample size requires careful consideration of several factors. The population size, desired confidence level, and acceptable error rate influence this calculation.
Statistical tables and specialized software help quality professionals select optimal sample sizes that balance accuracy with resource constraints.
For instance, when examining a batch of 10,000 products, quality engineers might determine that a sample size of 315 items provides 95% confidence in their findings.
This calculation takes into account the acceptable quality level (AQL) and the organization’s risk tolerance.
Sample Selection Methods That Work
Random selection forms the foundation of reliable attribute sampling. Quality teams employ various techniques to ensure unbiased sample selection:
Systematic sampling selects items at fixed intervals throughout the population. Stratified sampling divides the population into subgroups before selection.
Random number generators provide computerized selection methods.
Each method serves specific purposes, but random selection remains crucial for statistical validity. Modern software solutions often incorporate these selection methods, streamlining the process while maintaining accuracy.
Result Evaluation And Decision Making with Attribute Sampling
The evaluation phase transforms raw data into actionable insights. Quality professionals compare the number of defective items against predetermined acceptance criteria.
This comparison leads to clear accept/reject decisions about the entire population.
Statistical analysis tools help interpret results accurately. These tools calculate confidence intervals and provide statistical validation of findings.
Organizations use these results to make informed decisions about process improvements or corrective actions.
The Sequence That Drives Success
The correct order of attribute sampling follows this sequence:
- Define objectives and quality characteristics
- Determine population parameters
- Calculate required sample size
- Select appropriate sampling method
- Draw random samples
- Inspect selected items
- Record and analyze results
- Make accept/reject decisions
- Document findings
- Implement necessary actions
Following this sequence ensures methodical execution and reliable results. Each step builds upon previous ones, creating a logical progression from planning through implementation to final decision-making.
Quality managers must maintain detailed documentation throughout this process. Clear records support future audits and help identify trends over time.
This documentation also proves valuable when explaining decisions to stakeholders or regulatory bodies.
The attribute sampling process requires careful attention to detail and strict adherence to statistical principles.
When executed properly, it provides organizations with reliable data for quality control decisions while optimizing resource utilization.
Applications Of Attribute Sampling
Auditors rely heavily on attribute sampling to evaluate internal controls and financial statements.
During audit engagements, teams examine samples of transactions to verify compliance with established procedures.
For instance, auditors might review purchase orders to confirm proper authorization signatures or inspect payment records to verify appropriate documentation.
The audit attribute sampling process helps identify control weaknesses and potential risks.
When examining accounts payable, auditors might sample invoices to check for proper approval, matching purchase orders, and accurate payment processing.
This systematic approach allows audit teams to form conclusions about the entire population based on statistically valid samples.
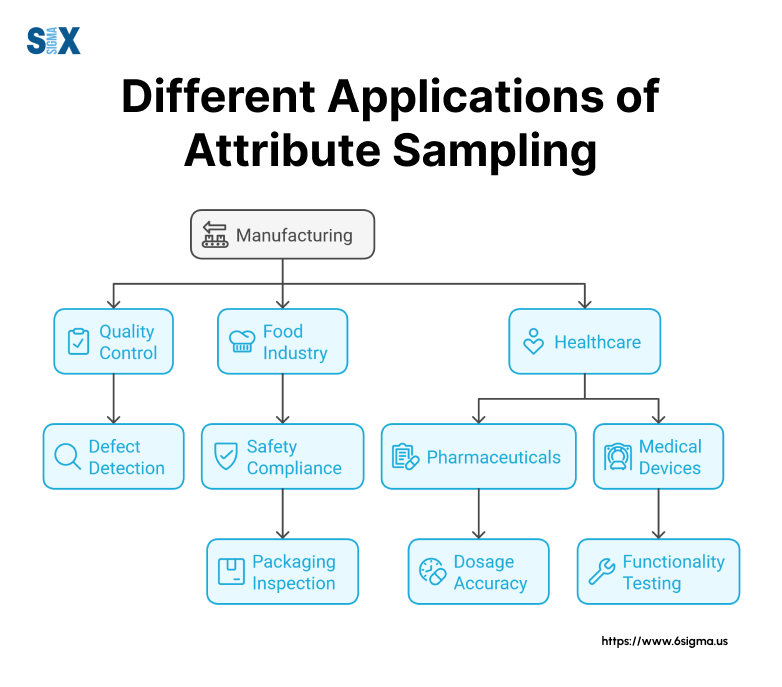
Quality Control Applications
Manufacturing facilities implement attribute sampling to maintain product quality standards.
Quality control teams inspect samples from production runs to identify defects or non-conforming items.
This approach proves particularly valuable in high-volume production environments where 100% inspection proves impractical.
Production managers use sampling results to make decisions about entire batches.
For example, in electronics manufacturing, quality teams might sample circuit boards to check for proper component placement. The results determine whether to acceptplans
or reject entire production lots.
Project Management Integration with Attribute Sampling
Project managers incorporate attribute sampling PMP principles into their quality management plans.
This integration helps teams monitor deliverables and ensure compliance with project requirements.
The Project Management Institute recognizes attribute sampling as a valuable tool for quality control in project environments.
Teams apply these techniques to various project aspects:
- Document review processes
- Deliverable inspections
- Milestone verifications
- Performance evaluations
Compliance Testing Framework
Attribute sampling for compliance serves as a crucial tool in regulatory environments. Organizations use this method to verify adherence to standards, regulations, and internal policies.
The process helps identify non-compliance issues while optimizing resource allocation.
Financial institutions employ attribute sampling to test compliance with banking regulations.
Healthcare organizations use it to verify medical record documentation. Manufacturing companies apply it to ensure adherence to safety standards.
The compliance testing framework typically involves:
- Identifying regulatory requirements
- Establishing acceptance criteria
- Selecting appropriate sample sizes
- Conducting systematic inspections
- Documenting findings
- Implementing corrective actions
Industry-Specific Applications of Attribute Sampling
Different sectors adapt attribute sampling to meet their unique needs. Healthcare facilities use it to monitor patient care documentation.
Retail operations apply it to inventory management. Technology companies implement it in software testing protocols.
Manufacturing organizations might examine:
- Product specifications
- Assembly procedures
- Packaging requirements
- Safety compliance
Service industries focus on:
- Customer interaction records
- Service delivery standards
- Documentation completeness
- Process adherence
Through these varied applications, attribute sampling provides organizations with reliable methods to maintain quality, ensure compliance, and make informed decisions.
The technique’s versatility makes it valuable across diverse business environments and operational contexts.
The success of attribute sampling applications depends on proper implementation and consistent execution.
Organizations must regularly evaluate and adjust their sampling procedures to maintain effectiveness and adapt to changing business requirements.
Statistical Foundations That Power Attribute Sampling
Probability theory provides the mathematical foundation for attribute sampling decisions. The basic principles establish how sample results reflect entire populations.
Quality managers use these principles to determine the likelihood of detecting defects or non-conforming items within their processes.
Statistical validity relies on random selection and independence of observations.
When examining a production batch, each item must have an equal chance of selection.
This randomness ensures the sample accurately represents the whole population, leading to reliable conclusions about product quality.
Working With Confidence Levels And Intervals
Confidence levels express the reliability of sampling results.
A 95% confidence level, commonly used in attribute sampling, indicates that similar samples would yield comparable results 95% of the time.
Quality professionals select appropriate confidence levels based on risk tolerance and business requirements.
Confidence intervals provide ranges where true population values likely exist.
For instance, if a sample shows a 3% defect rate with a ±1% confidence interval, the actual population defect rate probably falls between 2% and 4%.
These intervals help managers make informed decisions about process acceptance or rejection.
The Attribute Sampling Formula Explained
The attribute sampling formula determines required sample sizes and evaluates results. The basic formula incorporates several key variables:
n = (Z²pq)/E²
Where:
Z = Standard normal deviation for confidence level
p = Expected proportion
q = 1-p
E = Maximum allowable error
Quality engineers apply this formula to calculate minimum sample sizes needed for statistically valid results.
The formula balances precision requirements against resource constraints, enabling efficient sampling plans.
Understanding And Managing Sampling Error
Sampling error represents the difference between sample statistics and true population parameters.
This error occurs naturally in all sampling processes, but proper statistical methods help control its impact. Two main types affect attribute sampling results:
Random sampling error stems from natural variation in the selection process. This error decreases with larger sample sizes but never completely disappears.
Systematic error results from flawed sampling procedures or bias in selection methods. Unlike random error, systematic error requires procedural corrections to address.
Practical Error Management Strategies of Attribute Sampling
Quality managers implement several strategies to minimize sampling error:
- Increase sample sizes for greater precision
- Use stratified sampling when appropriate
- Maintain strict random selection procedures
- Document all sampling parameters
- Regular calibration of measurement systems
These strategies help ensure reliable results while maintaining practical resource constraints. Organizations must balance statistical precision against operational efficiency when designing sampling plans.
Statistical Tools For Decision Making
Modern statistical software packages assist in complex calculations and analysis. These tools help quality professionals:
- Calculate optimal sample sizes
- Generate random selection sequences
- Analyze sampling results
- Create statistical control charts
- Track sampling performance over time
The combination of sound statistical principles and proper tools enables organizations to implement effective attribute sampling programs.
Regular evaluation and adjustment of statistical parameters ensure continued reliability of sampling results.
Transform your statistical knowledge into leadership action with our Six Sigma Champion certification
Designing An Effective Attribute Sampling Plan
The foundation of any attribute sampling plan begins with well-defined objectives.
Quality managers must specify exactly what characteristics they need to evaluate and why these measurements matter to organizational goals.
These objectives guide all subsequent decisions in the sampling process.
Organizations typically focus their sampling objectives on specific quality characteristics.
For example, a pharmaceutical company might examine batch uniformity, while a financial institution checks transaction accuracy.
Each objective requires precise definition to ensure consistent evaluation across all samples.
Establishing Quality Levels That Matter
Acceptable quality levels (AQL) define the maximum percentage of nonconforming items a business considers satisfactory.
Setting these levels requires careful balance between quality standards and operational realities.
Quality engineers must consider industry standards, customer requirements, and cost implications when determining appropriate AQLs.
The process of setting quality levels involves:
- Reviewing industry standards
- Analyzing historical performance data
- Evaluating customer expectations
- Considering regulatory requirements
- Assessing cost implications
Building Your Attribute Sampling Plan
Creating an effective sampling plan requires systematic approach and attention to detail. The plan must specify sample sizes, selection methods, and acceptance criteria.
Quality professionals develop these plans to ensure consistent application across different inspection scenarios.
Key elements of a robust sampling plan include:
- Selection criteria for items to be sampled
- Detailed inspection procedures
- Clear acceptance and rejection guidelines
- Documentation requirements
- Response protocols for identified issues
Implementation Strategies That Drive Results
Successful implementation of attribute sampling plans depends on proper training and consistent execution.
Quality teams must ensure all personnel understand sampling procedures and evaluation criteria. Regular monitoring helps maintain consistency and identifies potential improvements.
Organizations should establish:
- Training programs for inspection personnel
- Standard operating procedures
- Quality control checkpoints
- Documentation systems
- Review and feedback mechanisms
Monitoring And Adjustment Protocols of Attribute Sampling
Regular evaluation of sampling plan effectiveness ensures continued relevance and reliability.
Quality managers should review results periodically to identify trends and potential areas for improvement.
This ongoing assessment helps organizations maintain optimal sampling procedures while adapting to changing conditions.
Performance indicators to monitor include:
- Sampling accuracy rates
- Inspector consistency
- Resource utilization
- Detection rates
- Process efficiency metrics
Documentation And Control Systems
Proper documentation forms the backbone of successful attribute sampling plans.
Organizations must maintain detailed records of sampling procedures, results, and any corrective actions taken. These records provide valuable data for future analysis and demonstrate regulatory compliance.
Essential documentation includes:
- Sampling plan specifications
- Inspection results
- Nonconformance reports
- Corrective action records
- Training documentation
Through careful design and implementation of attribute sampling plans, organizations can maintain quality standards while optimizing resource allocation.
Regular review and adjustment ensure these plans continue to meet evolving business needs and quality requirements.
Best Practices And Common Pitfalls In Attribute Sampling
Sample representativeness stands as the cornerstone of reliable attribute sampling results. Quality managers must ensure selected samples accurately reflect the entire population under study.
This requirement demands careful attention to selection methods and timing of sample collection.
Several factors influence sample representativeness. Production variations throughout shifts, material changes, and environmental conditions can affect product characteristics.
Quality teams should account for these variables when designing sampling schedules and selection criteria.
Eliminating Selection Bias
Selection bias poses a significant threat to sampling validity.
Quality professionals must implement strict random selection procedures to prevent conscious or unconscious bias from affecting results.
Automated selection tools and standardized procedures help maintain objectivity throughout the sampling process.
Methods for reducing selection bias include:
- Implementing automated selection systems
- Rotating inspection personnel
- Standardizing selection procedures
- Documenting selection criteria
- Regular procedure audits
Result Interpretation Guidelines of Attribute Sampling
Accurate interpretation of sampling results requires both statistical knowledge and practical understanding.
Quality engineers must consider confidence intervals, margin of error, and historical trends when analyzing data. Misinterpretation can lead to incorrect conclusions and inappropriate corrective actions.
Key considerations for result interpretation:
- Statistical significance levels
- Historical performance patterns
- Process capability factors
- Environmental influences
- Operational constraints
Process Improvement Strategies
Continuous improvement in attribute sampling requires systematic evaluation and adjustment.
Organizations should regularly review their sampling procedures, update methods based on new technologies, and incorporate lessons learned from previous experiences.
Improvement areas typically include:
- Sampling efficiency
- Data collection methods
- Analysis techniques
- Documentation systems
- Training programs
Technology Integration For Better Results
Modern technology offers numerous tools to enhance attribute sampling processes.
Quality teams should evaluate and implement appropriate software solutions for sample selection, data collection, and analysis. These tools can improve accuracy while reducing resource requirements.
Beneficial technological applications include:
- Statistical analysis software
- Automated data collection systems
- Digital documentation platforms
- Real-time monitoring tools
- Reporting dashboards
Training And Competency Development with Attribute Sampling
Effective attribute sampling depends heavily on well-trained personnel.
Organizations must provide comprehensive training programs covering sampling procedures, statistical concepts, and result interpretation. Regular refresher training helps maintain consistency and accuracy.
Essential training components include:
- Statistical principles
- Sampling procedures
- Documentation requirements
- Analysis techniques
- Quality standards
Documentation Best Practices
Proper documentation supports both current operations and future improvements.
Quality managers should establish clear guidelines for recording sampling activities, results, and corrective actions. These records provide valuable data for trend analysis and process optimization.
Required documentation typically covers:
- Sampling plans
- Selection procedures
- Inspection results
- Corrective actions
- Training records
Through careful attention to these best practices and active management of common pitfalls, organizations can maintain effective attribute sampling programs.
Regular review and updates ensure continued relevance and reliability of sampling processes.
Moving Forward With Attribute Sampling
Attribute sampling serves as a fundamental tool in quality control and process improvement.
The method provides organizations with reliable data for decision-making while optimizing resource allocation.
From manufacturing floors to financial audits, attribute sampling delivers consistent results when properly implemented.
Statistical validity remains crucial for effective sampling programs. Organizations must maintain proper sample sizes, selection methods, and evaluation criteria.
These elements work together to ensure reliable conclusions about population characteristics based on sample results.
The Business Impact Of Effective Sampling
Mastering attribute sampling brings significant benefits to organizations across industries.
Quality improvements lead to reduced costs, enhanced customer satisfaction, and stronger competitive positions. The systematic approach to quality control enables data-driven decisions that support business objectives.
Quality managers who implement robust sampling programs often see improvements in:
- Process efficiency
- Product quality
- Customer satisfaction
- Regulatory compliance
- Resource utilization
Building On Current Knowledge
The field of attribute sampling continues to evolve with new technologies and methodologies. Quality professionals should stay informed about emerging trends and best practices.
Regular training and professional development ensure sampling programs remain effective and efficient.
Organizations benefit from:
- Updated statistical methods
- Advanced analysis tools
- Improved documentation systems
- Enhanced training programs
- Refined implementation strategies
The Path Forward
Success in attribute sampling requires commitment to continuous improvement. Quality teams should regularly evaluate their programs, incorporate new technologies, and refine their processes.
This ongoing development ensures sampling methods remain aligned with business needs and industry standards.
Future developments in attribute sampling will likely include:
- Advanced statistical software
- Automated sampling systems
- Real-time analysis capabilities
- Integrated quality management
- Enhanced reporting tools
Quality professionals who master attribute sampling position themselves and their organizations for continued success.
The systematic approach to quality control through sampling provides a foundation for sustainable improvement and competitive advantage in today’s business environment.
Through dedicated application of attribute sampling principles, organizations can maintain high quality standards while optimizing operational efficiency.
The investment in proper sampling methods pays dividends through improved quality, reduced costs, and enhanced customer satisfaction.
SixSigma.us offers both Live Virtual classes as well as Online Self-Paced training. Most option includes access to the same great Master Black Belt instructors that teach our World Class in-person sessions. Sign-up today!
Virtual Classroom Training Programs Self-Paced Online Training Programs