Data-Driven Process Improvement: Boosting Business Performance
Data-driven process improvement strategies help companies to stay competitive and on the edge.
Organization optimize their operations and reach to measurable results with this methodological approach which transforms raw data into actionable insights.
What This Guide Covers
- Understanding Data Analysis Methods
- Implementing Process Changes
- Measuring Performance Metrics
- Future Technology Integration
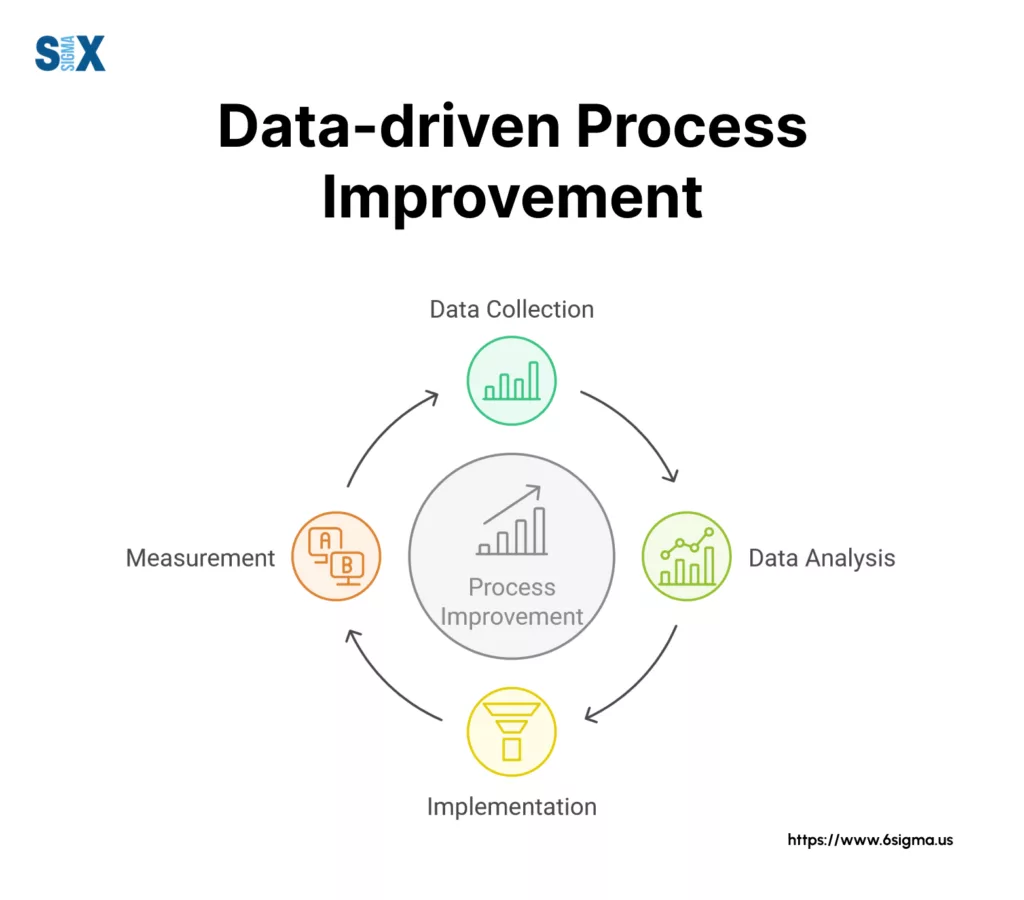
What is Data-Driven Process Improvement?
When business changes their operations through systematic analysis of operational data, that process is called as Data-driven process improvement.
Business grow and change with this data-driven process improvement by systematic analysis of operational data.
To identify inefficiencies, implemented solutions, and measure results it needs relevant metrics.
Data’s Critical Role in Process Enhancement
From the daily operations of these modern businesses, vast amount of data is been generated.
The generated data helps identifying bottlenecks,inefficiencies, and opportunities for improvement.
By analyzing historical and real-time data, organizations can:
- Track performance metrics against established benchmarks
- Identify root causes of recurring problems
- Predict potential issues before they impact operations
- Validate the effectiveness of implemented solutions
Integration of Data-Driven Process Improvement with Six Sigma Methodology
Six Sigma methodology enhances data-driven process improvement through its structured DMAIC framework.
This systematic approach aligns perfectly with data-driven decision making, providing a proven roadmap for achieving measurable results.
The DMAIC process consists of five key phases:
- Define: Identify specific process problems using data
- Measure: Collect baseline data on current performance
- Analyze: Use statistical tools to identify root causes
- Improve: Implement and test solutions based on data
- Control: Monitor results and maintain improvements
Organizations that combine Six Sigma principles with data-driven approaches often achieve superior results compared to those using either method alone.
Transform your organization’s process improvement initiatives with proven Six Sigma leadership techniques.
Tools and Technologies of Data-driven Process Improvement
Modern data-driven process improvement relies on various analytical tools and technologies.
Statistical software packages help analyze complex data sets, while process mapping tools visualize workflows and identify improvement opportunities.
Advanced analytics platforms incorporate machine learning algorithms to detect patterns and predict outcomes with increasing accuracy.
These tools support the systematic analysis of process data, enabling organizations to:
- Generate detailed performance reports
- Create statistical models for process optimization
- Visualize data through charts and graphs
- Monitor real-time process metrics
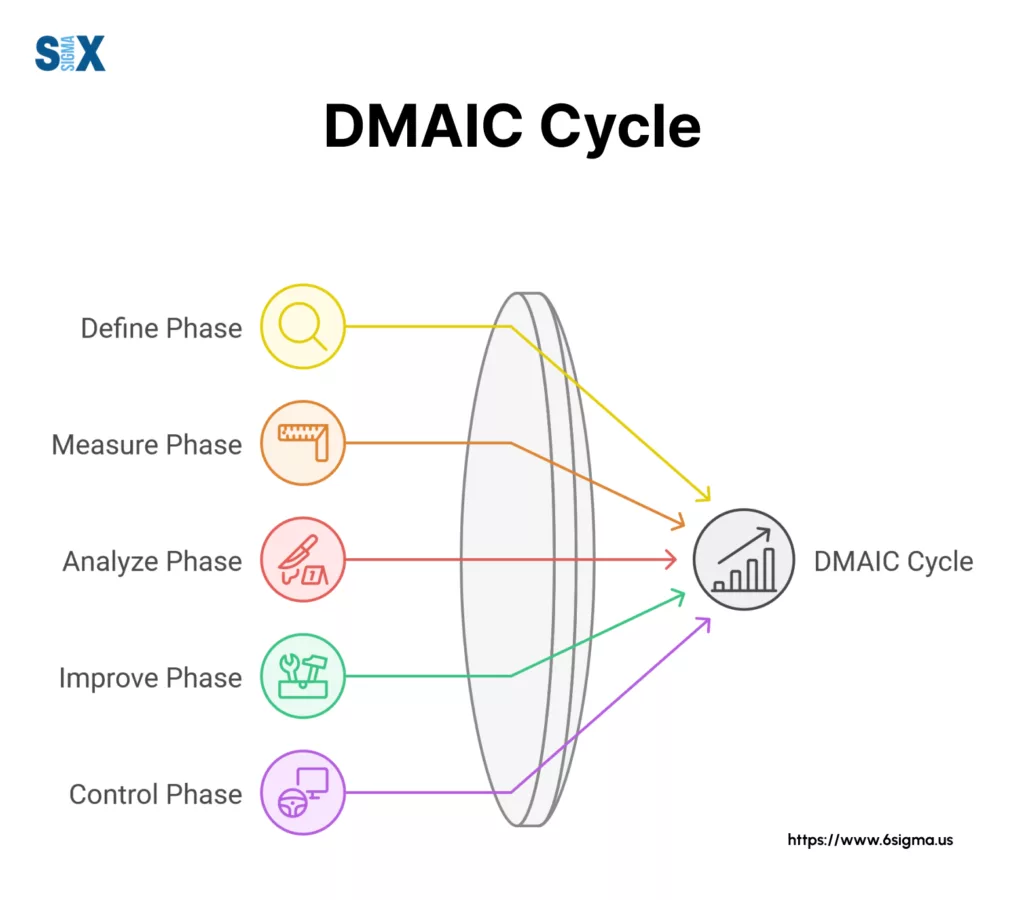
Stop struggling with statistical analysis. Master Minitab’s powerful tools for data-driven decision making.
Benefits of Data-Driven Decision Making
Data-driven decision making transforms operational efficiency by identifying and eliminating process bottlenecks.
Organizations that implement this approach typically report 20-30% improvement in productivity across their operations.
The systematic analysis of workflow data reveals hidden inefficiencies and opportunities for streamlining processes.
Teams can then focus their efforts on high-impact improvements that deliver measurable results.
Key performance indicators track these improvements, providing real-time feedback on process changes.
These gains stem from the ability to identify and address issues before they impact production schedules.
Quality Improvements and Customer Satisfaction
Data-driven process improvement directly influences product quality and customer satisfaction levels.
Organizations monitoring quality metrics through statistical process control have reported defect reductions of up to 40%.
This improvement translates into higher customer satisfaction scores and increased loyalty.
The connection between quality improvements and customer satisfaction becomes evident through:
- Reduced product defect rates
- Faster response times to quality issues
- More consistent product performance
- Higher customer retention rates
Regular analysis of customer feedback data enables organizations to identify and address satisfaction drivers proactively.
Optimizing Costs and Resources with Data-Driven Process Improvement
Resource optimization through data-driven decision making delivers significant cost savings. Organizations implementing these methods typically achieve:
- 15-25% reduction in operational costs
- 30-40% improvement in resource utilization
- 20-30% decrease in waste reduction
- Substantial returns on improvement investments
The ability to analyze resource usage patterns enables precise allocation of materials, labor, and equipment.
Manufacturing companies have reported saving millions annually through data-driven optimization of their production processes.
Enhanced Risk Management Capabilities
Data-driven approaches strengthen risk management by enabling predictive analysis of potential issues.
Organizations can identify risk patterns and implement preventive measures before problems occur. This proactive stance has resulted in:
- Reduced downtime frequency
- Lower maintenance costs
- Fewer safety incidents
- Improved regulatory compliance
Financial institutions using data-driven risk assessment models have reduced their exposure to losses by up to 35% while maintaining growth targets.
The ability to quantify and predict risks enables more effective mitigation strategies and resource allocation.
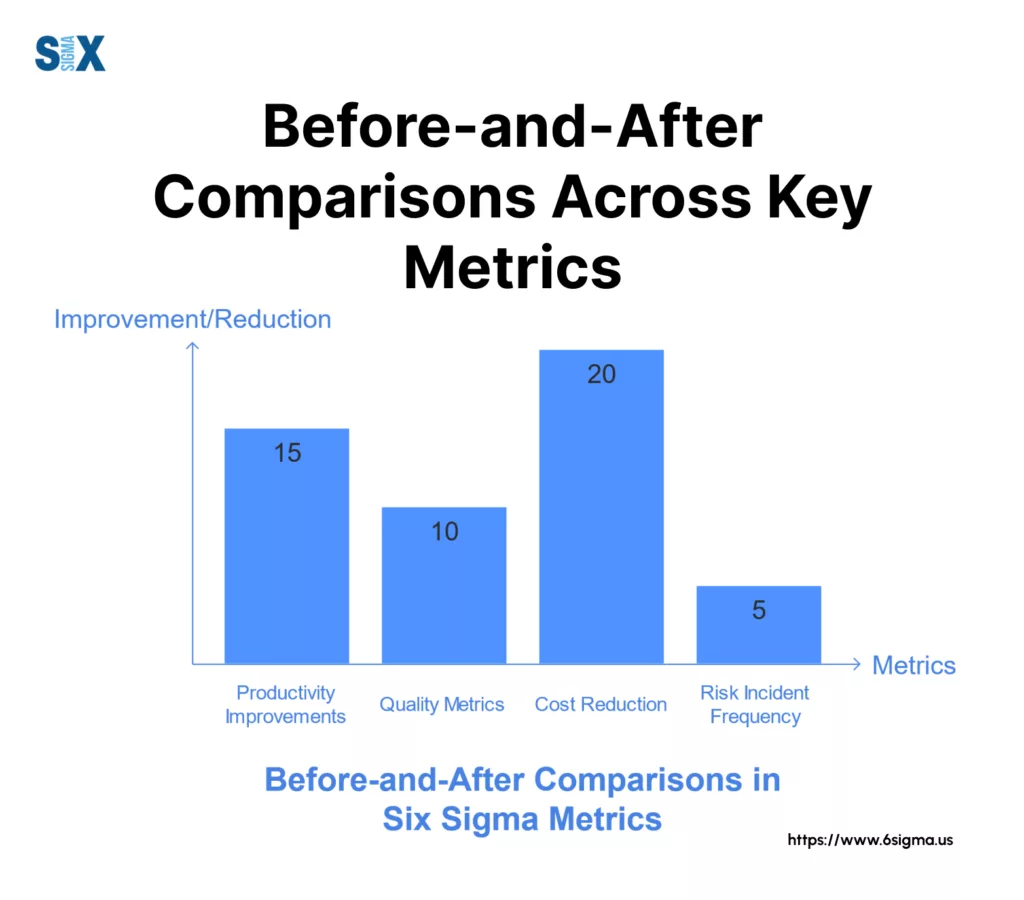
The benefits of data-driven decision making extend beyond immediate operational improvements.
Organizations that embrace this approach build stronger foundations for sustainable growth and competitive advantage.
A Steps to Implement Data-Driven Processes
The foundation of successful data-driven process improvement begins with selecting appropriate key performance indicators (KPIs).
These metrics must align with organizational goals and provide meaningful insights into process performance.
Data Collection and Preparation Methods
Implementing data-driven processes requires systematic data collection methods. Organizations must establish reliable data sources and standardized collection procedures.
This phase involves:
- Setting up automated data collection systems
- Validating data accuracy and consistency
- Creating data storage and management protocols
- Establishing data cleaning procedures
The quality of collected data directly impacts the effectiveness of process improvement initiatives.
Organizations should implement validation checks and regular audits to maintain data integrity throughout the collection process.
Analysis and Visualization Techniques
Statistical tools and techniques transform raw data into actionable insights. Modern analysis methods include regression analysis, statistical process control, and predictive modeling.
These tools help identify patterns, correlations, and potential areas for improvement within processes.
Data visualization plays a crucial role in communicating findings to stakeholders.
Charts, graphs, and dashboards make complex data patterns more accessible and facilitate better decision-making.
Developing Strategic Improvements with Data-Driven Process Improvement
The analysis phase reveals opportunities for process enhancement. Organizations must prioritize these opportunities based on:
- Potential impact on key metrics
- Resource requirements
- Implementation complexity
- Expected return on investment
Successful improvement strategies often start with pilot programs to validate proposed changes before full-scale implementation.
This approach minimizes risks and allows for refinements based on initial results.
Implementation and Performance Monitoring
Rolling out process improvements requires careful planning and coordination. Organizations should:
- Create detailed implementation schedules
- Assign clear responsibilities
- Establish communication channels
- Set up monitoring systems
Regular monitoring helps track the effectiveness of implemented changes and identifies any necessary adjustments. Organizations should maintain detailed records of changes and their impacts on key metrics.
Continuous Evaluation and Refinement with Data-Driven Process Improvement
Data-driven process improvement demands ongoing evaluation and refinement. Organizations must regularly review performance data and adjust their approaches based on results.
This cycle ensures sustained improvement and helps identify new opportunities for enhancement.
Performance reviews should occur at scheduled intervals, with formal assessments of:
- Progress against target metrics
- Effectiveness of implemented changes
- Resource utilization
- New improvement opportunities
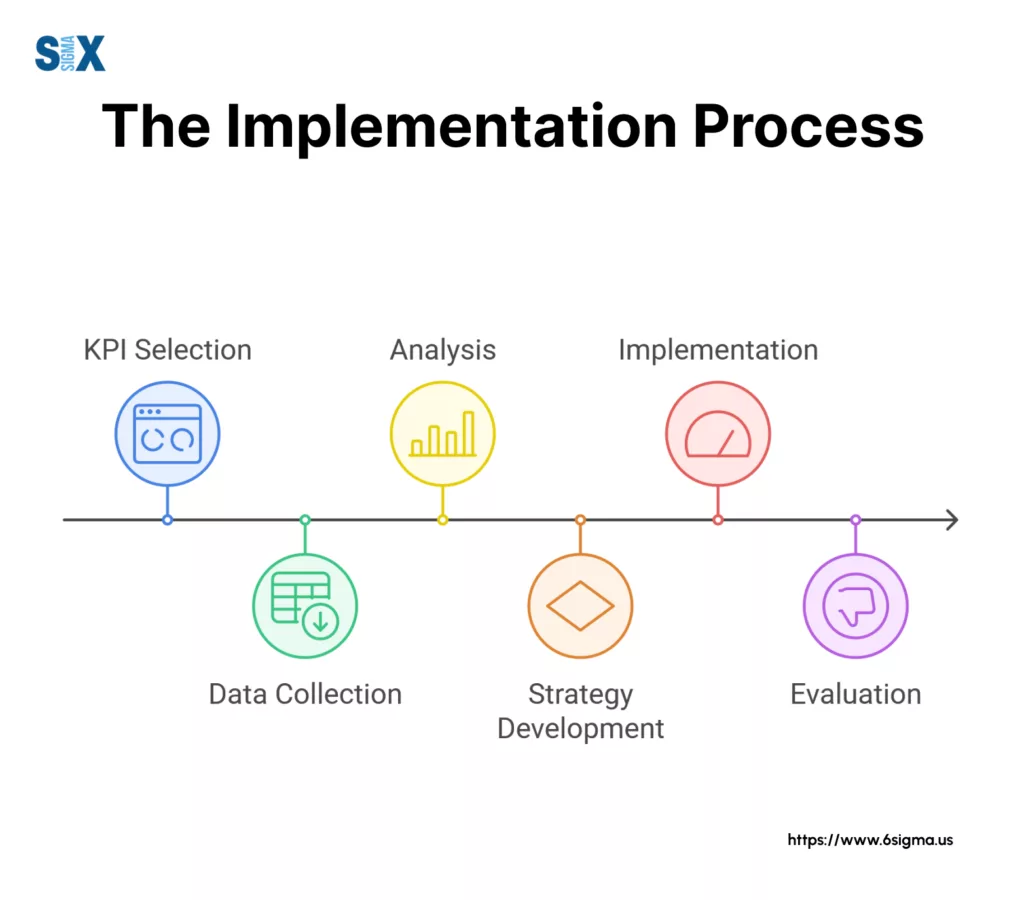
The success of data-driven process improvement relies heavily on maintaining momentum throughout the implementation cycle.
Organizations must remain committed to the process and be prepared to make adjustments as needed.
This structured approach to implementing data-driven processes provides organizations with a clear roadmap for success.
Advanced Data Analysis Techniques and Tools
Statistical analysis forms the backbone of data-driven process improvement projects.
Modern organizations employ various statistical tools and techniques to extract meaningful insights from process data.
Six Sigma projects frequently utilize statistical process control (SPC) charts to monitor and control process variations.
Machine Learning and AI Applications within Data-Driven Process Improvement
Machine learning algorithms revolutionize process improvement by identifying complex patterns in large datasets.
Predictive maintenance models analyze equipment sensor data to forecast potential failures before they occur.
Natural language processing techniques extract valuable insights from customer feedback and service records, enabling proactive process adjustments.
AI-powered process optimization tools can:
- Automatically detect anomalies in real-time data streams
- Generate optimal process parameters based on historical performance
- Predict quality issues before they impact production
- Recommend specific improvement actions based on analyzed patterns
These advanced capabilities significantly reduce the time required to identify and implement process improvements, while increasing the accuracy of predictions and recommendations.
Data Visualization Tools and Techniques
Modern data visualization tools transform complex data sets into clear, actionable insights.
Tools like Tableau, Power BI, and QlikView enable organizations to create interactive dashboards that display real-time process metrics.
These platforms support various visualization types, from basic bar charts to sophisticated heat maps and network diagrams.
- Effective visualization techniques include:
- Process control charts for monitoring stability
- Pareto charts for identifying critical factors
- Box plots for analyzing data distributions
- Scatter plots for correlation analysis
Organizations implementing Six Sigma projects particularly benefit from these visualization tools, as they help communicate complex statistical concepts to diverse stakeholders.
Process Mining and Analysis
Process mining techniques extract insights directly from event logs and system data.
This approach reveals actual process flows, bottlenecks, and deviations from intended procedures. Advanced process mining tools can:
- Map actual process flows automatically
- Identify process variations and their frequencies
- Calculate process performance metrics
- Monitor compliance with standard procedures
Process mining provides objective evidence of how processes actually operate, often revealing unexpected patterns and improvement opportunities that traditional analysis methods might miss.
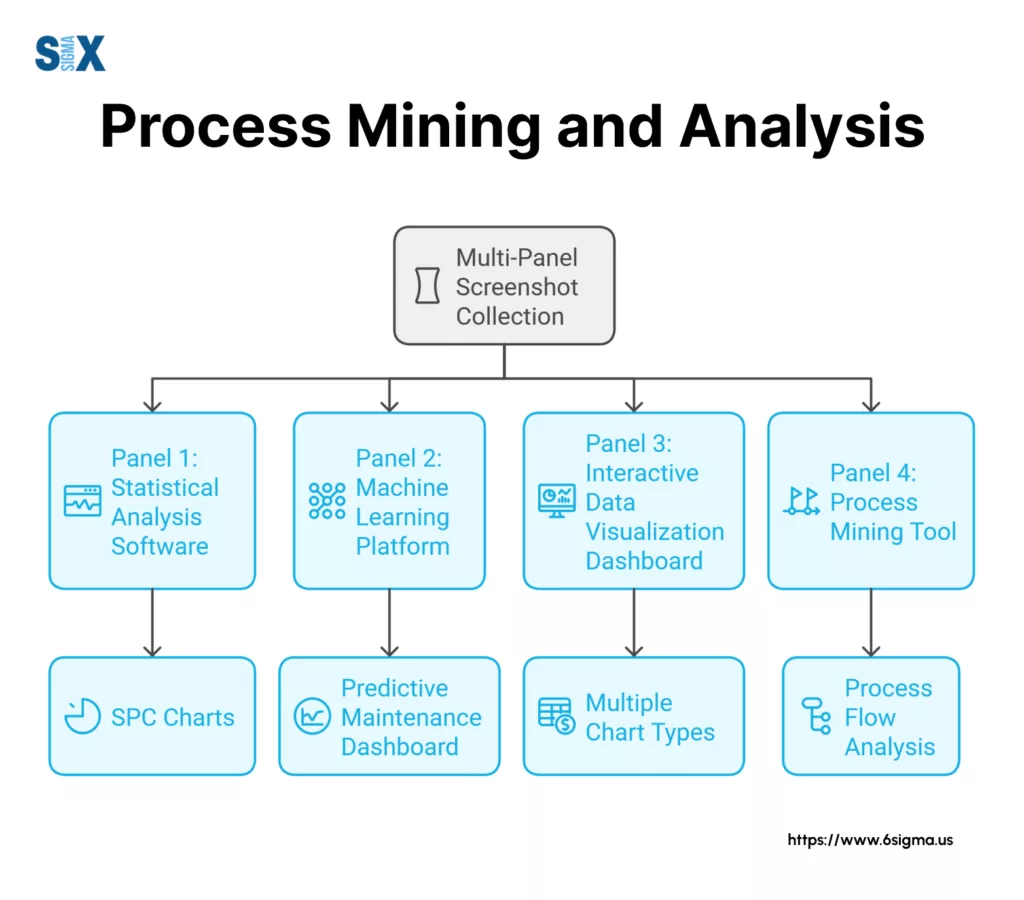
The integration of these advanced analytical tools creates a powerful ecosystem for data-driven process improvement.
Organizations must select appropriate tools based on their specific needs and ensure proper training for team members who will use these technologies.
Success in implementing these advanced techniques requires a balanced approach.
While sophisticated tools offer powerful capabilities, organizations should focus on selecting methods that align with their process improvement goals and team capabilities.
Industry-Specific Applications and Case Studies of Data-Driven Process Improvement
Manufacturing organizations lead the way in implementing data-driven processes in their operations.
Toyota’s production system demonstrates the power of data-driven process improvement through their implementation of Six Sigma methodologies.
Their approach reduced production defects by 45% and increased throughput by 30% across multiple plants.
Another notable example comes from General Electric’s turbine manufacturing division.
By implementing data-driven processes in their quality control systems, they achieved a 75% reduction in inspection time while improving accuracy rates by 25%.
Healthcare Transformation and Patient Care
Healthcare organizations utilize data-driven process improvement to enhance patient care and operational efficiency.
Mayo Clinic revolutionized their patient scheduling system through detailed analysis of appointment data and patient flow patterns.
This initiative reduced wait times by 35% and increased patient satisfaction scores by 28%.
Financial Services Innovation
To enhance risk management and customer service various banks leverage data-driven processes.
JPMorgan Chase implemented advanced analytics in their fraud detection systems, resulting in a 35% improvement in fraud identification accuracy while reducing false positives by 45%.
Implementing advance analytics
Goldman Sachs transformed their trading operations through Six Sigma processes and data analytics.
Their implementation of automated trading algorithms, based on historical pattern analysis, improved trading efficiency by 50% and reduced operational errors by 65%.
These improvements translated into significant cost savings and enhanced customer satisfaction.
Retail and E-commerce Evolution
In inventory management and customer experience the retail sector demonstrates powerful applications of data-driven process improvement
Walmart’s supply chain transformation improved inventory turnover by 25% reduced out-of-stock incidents by 40% through data analytics.
Fulfillment centers showcases the potential for operational excellence from Amazon’s implementation of data-driven processes.
Their automated picking and packing systems, optimized through continuous data analysis, reduced order processing time by 50% while maintaining 99.9% accuracy rates.
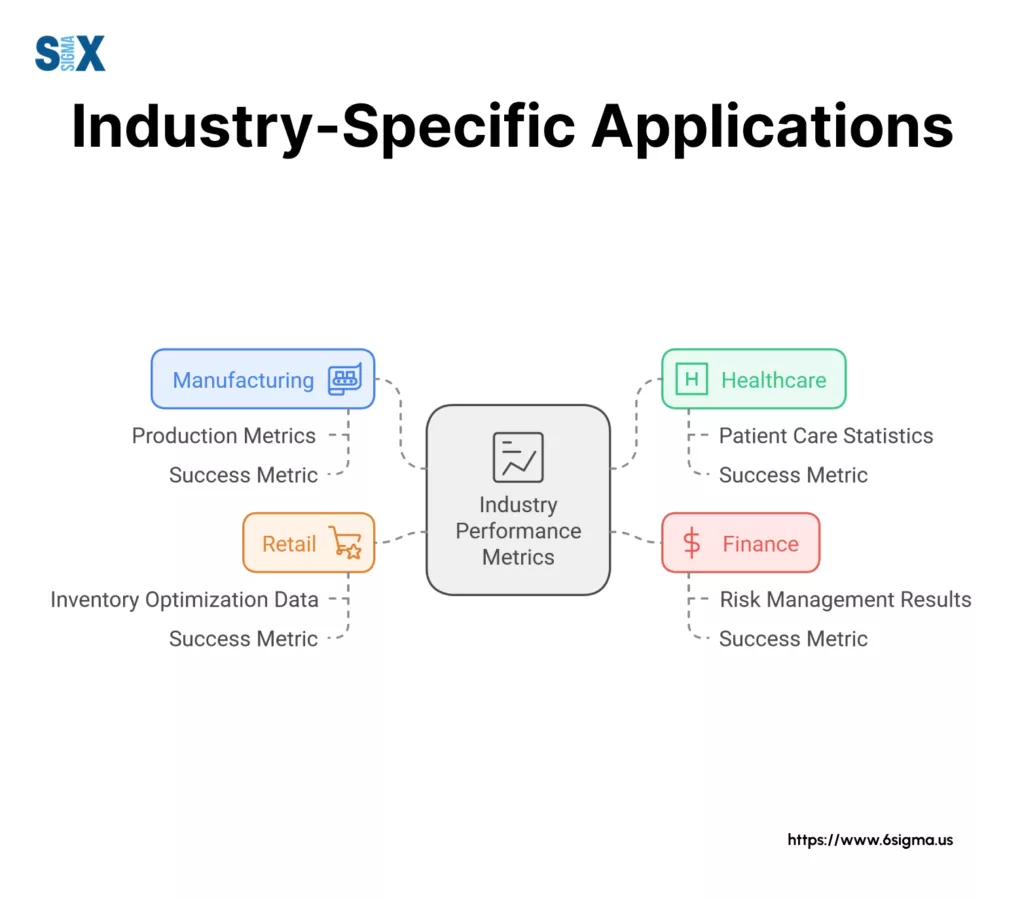
The success stories shows how these industries adapt data-driven process improvement.
Manufacturing focuses on production efficiency and quality control, while healthcare prioritizes patient outcomes and resource utilization.
Financial services emphasize risk management and transaction accuracy, and retail concentrates on inventory optimization and customer satisfaction.
The implementation of Six Sigma methodologies across these industries reveals common success factors:
- Systematic data collection and analysis
- Clear definition of success metrics
- Regular monitoring and adjustment of processes
- Strong leadership commitment to data-driven decision making
Frequently Asked Questions
Data-driven process improvement combines statistical analysis with systematic methodologies to enhance business operations. This approach relies on measurable data to identify areas for enhancement, implement changes, and verify results.
Data processing forms the foundation of process improvement by transforming raw data into actionable insights. This involves collecting, cleaning, and analyzing operational data through statistical methods.
The seven fundamental steps of data-driven process improvement include:
– Define measurement objectives
– Collect relevant data
– Analyze current performance
– Identify improvement opportunities
– Implement changes
– Monitor results
– Standardize successful changes
Organizations can strengthen their data-driven decision making by establishing clear key performance indicators, implementing robust data collection systems, and utilizing appropriate statistical tools and techniques.
Popular data-driven techniques include statistical process control, regression analysis, and hypothesis testing. Organizations also employ advanced methods such as machine learning algorithms and predictive analytics.
Essential KPIs vary by industry but typically include:
– Process efficiency metrics
– Quality measurements
– Customer satisfaction scores
– Cost reduction indicators
– Productivity ratios
– Error rates and defect counts
Data processing types include:
– Descriptive analytics examining historical performance
– Diagnostic analytics identifying problem root causes
– Predictive analytics forecasting future trends
– Prescriptive analytics recommending specific actions
Six Sigma methodology provides a structured framework for data-driven process improvement.
This approach emphasizes statistical analysis and measurement-based goals.
Modern technology enables more sophisticated data collection, analysis, and visualization capabilities. Advanced analytics platforms, artificial intelligence, and machine learning tools enhance the ability to identify patterns and predict outcomes.
SixSigma.us offers both Live Virtual classes as well as Online Self-Paced training. Most option includes access to the same great Master Black Belt instructors that teach our World Class in-person sessions. Sign-up today!
Virtual Classroom Training Programs Self-Paced Online Training Programs