The Importance of Qualitative Data Analysis in Research: A Comprehensive Guide
Qualitative data analysis, in essence, is the systematic examination of non-numerical information to uncover patterns, themes, and insights.
This process is crucial in various fields, from product development to business process improvement.
Key Highlights
- Defining qualitative data analysis and its importance
- Comparing qualitative and quantitative research methods
- Exploring key approaches: thematic, grounded theory, content analysis
- Understanding the qualitative data analysis process
- Reviewing CAQDAS tools for efficient analysis
- Ensuring rigor through triangulation and member checking
- Addressing challenges and ethical considerations
- Examining future trends in qualitative research
Introduction to Qualitative Data Analysis
Qualitative data analysis is a sophisticated process of examining non-numerical information to extract meaningful insights.
It’s not just about reading through text; it’s about diving deep into the nuances of human experiences, opinions, and behaviors.
This analytical approach is crucial in various fields, from product development to process improvement, and even in understanding complex social phenomena.
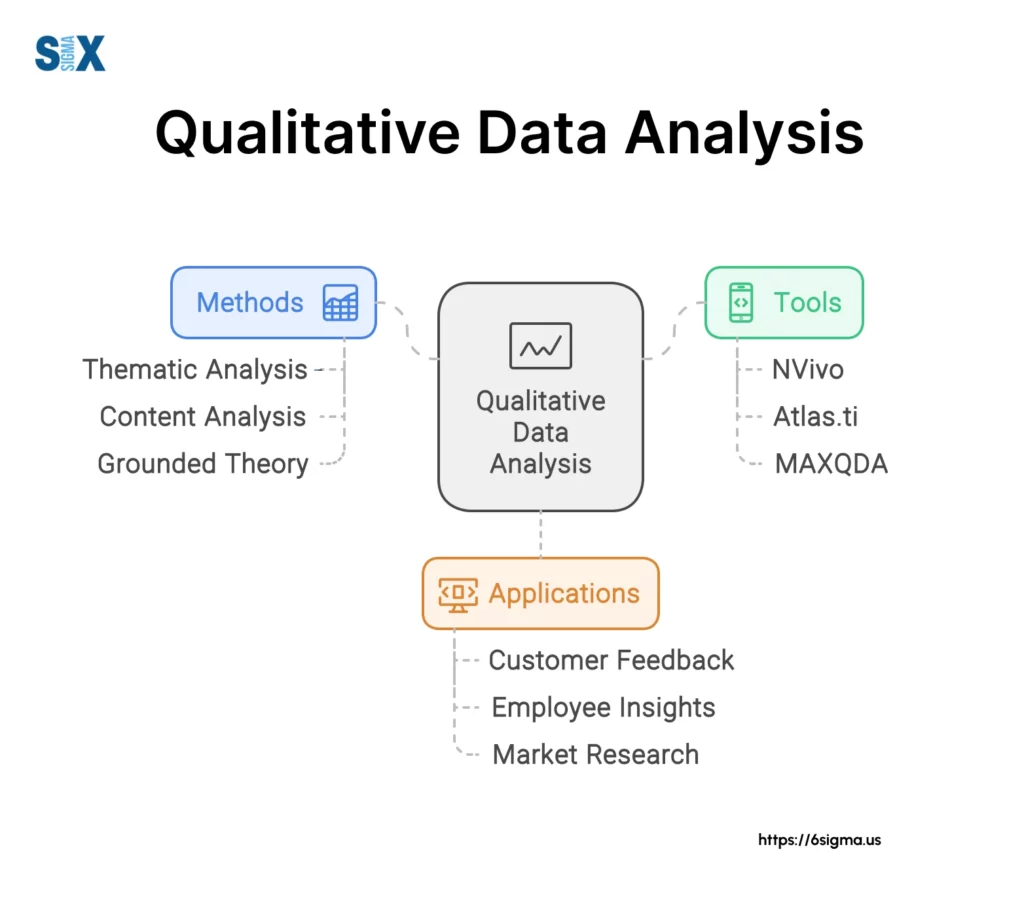
Importance of Qualitative Research Methods
The importance of qualitative research methods cannot be overstated. In my work with companies like 3M, Dell, and Intel, I’ve seen how qualitative analysis can uncover insights that numbers alone simply can’t reveal.
These methods allow us to understand the ‘why’ behind the ‘what’, providing context and depth to our understanding of complex issues.
Whether it’s improving a manufacturing process or developing a new product, qualitative research methods offer a rich, nuanced perspective that’s invaluable for informed decision-making.
Comparing Qualitative vs Quantitative Analysis
While both qualitative and quantitative analyses are essential tools in a researcher’s arsenal, they serve different purposes.
Quantitative analysis, which I’ve extensively used in Six Sigma projects, deals with numerical data and statistical methods.
It’s excellent for measuring, ranking, and categorizing phenomena. On the other hand, qualitative analysis focuses on the rich, contextual data that can’t be easily quantified.
It’s about understanding meanings, experiences, and perspectives.
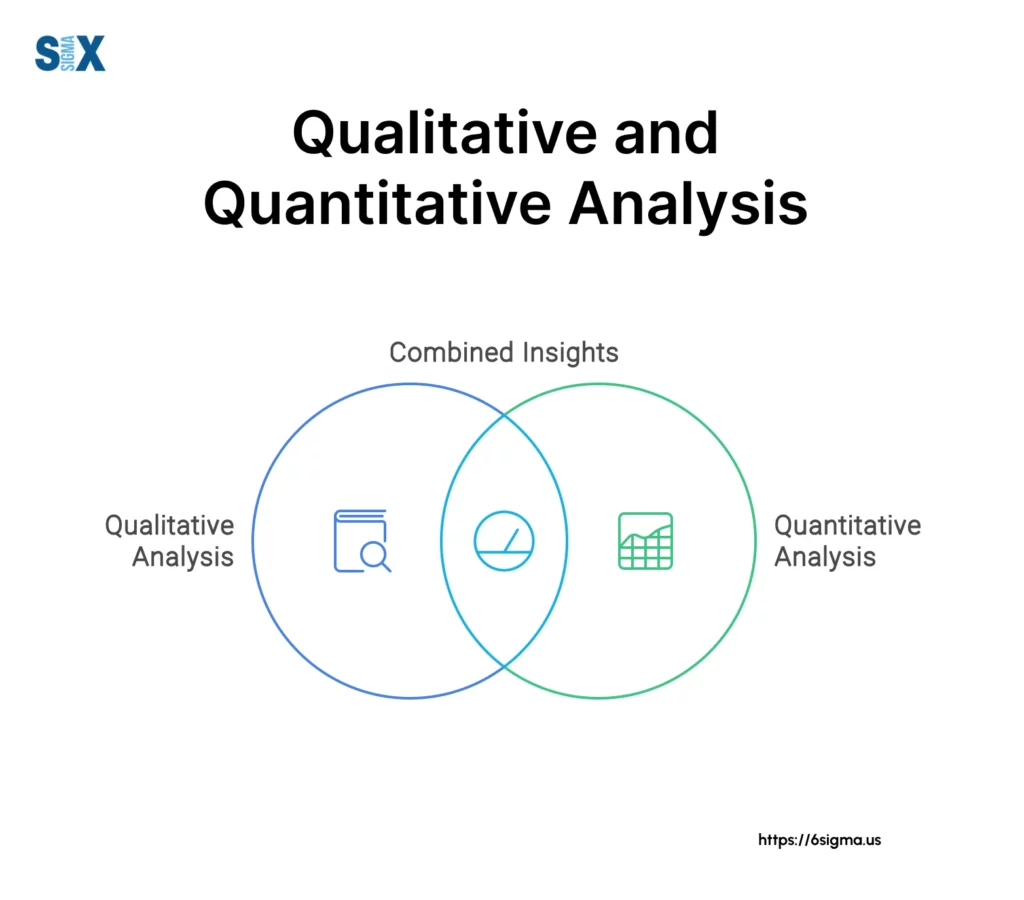
Key Approaches in Qualitative Data Analysis
Explore essential techniques like thematic analysis, grounded theory, content analysis, and discourse analysis.
Understand how each approach offers unique insights into qualitative data interpretation and theory building.
Thematic Analysis Techniques
Thematic analysis is a cornerstone of qualitative data analysis. It involves identifying patterns or themes within qualitative data.
In my workshops on Statistical Thinking and Business Process Charting, I often emphasize the power of thematic analysis in uncovering underlying patterns in complex datasets.
This approach is particularly useful when dealing with interview transcripts or open-ended survey responses.
The key is to immerse yourself in the data, coding it systematically, and then stepping back to see the broader themes emerge.
Grounded Theory Methodology
Grounded theory is another powerful approach in qualitative data analysis. Unlike methods that start with a hypothesis, grounded theory allows theories to emerge from the data itself.
I’ve found this particularly useful in projects where we’re exploring new territory without preconceived notions.
It’s a systematic yet flexible approach that can lead to fresh insights and innovative solutions.
The iterative nature of grounded theory, with its constant comparison of data, aligns well with the continuous improvement philosophy of Six Sigma.
Content Analysis Strategies
Content analysis is a versatile method that can be both qualitative and quantitative.
In my experience working with diverse industries, content analysis has been invaluable in making sense of large volumes of textual data.
Whether it’s analyzing customer feedback or reviewing technical documentation, content analysis provides a structured way to categorize and quantify qualitative information.
The key is to develop a robust coding framework that captures the essence of your research questions.
Discourse Analysis Approaches
Discourse analysis takes a deeper look at language use and communication practices.
It’s not just about what is said, but how it’s said and in what context. In my work on improving communication processes within organizations, discourse analysis has been a powerful tool.
It helps uncover underlying assumptions, power dynamics, and cultural nuances that might otherwise go unnoticed.
This approach is particularly useful when dealing with complex organizational issues or when trying to understand stakeholder perspectives in depth.
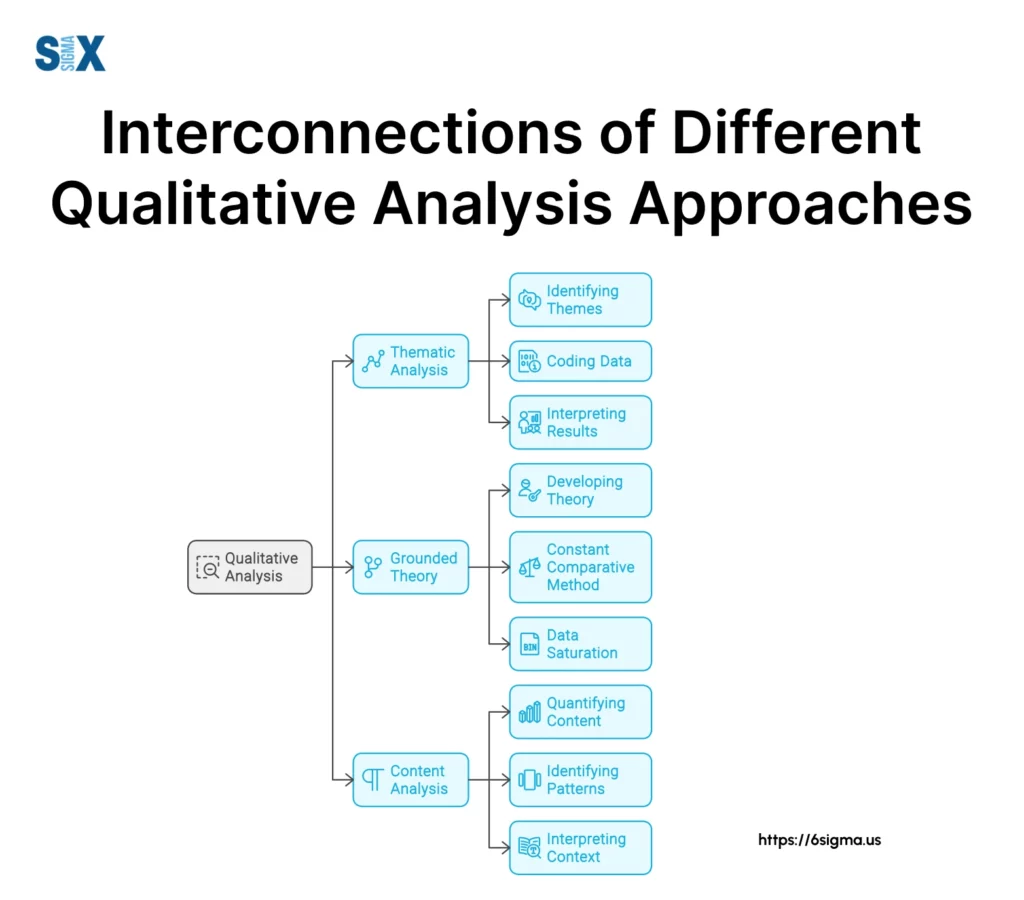
The Qualitative Data Analysis Process
Navigate through data collection, coding techniques, theme development, and interpretation. Learn how to transform raw qualitative data into meaningful insights through systematic analysis.
Data collection methods (interviews, focus groups, observation)
The foundation of any good qualitative analysis lies in robust data collection. In my experience, a mix of methods often yields the best results.
In-depth interviews provide individual perspectives, focus groups offer insights into group dynamics, and observation allows us to see behaviors in their natural context.
When working on process improvement projects, I often combine these methods to get a comprehensive view of the situation.
The key is to align your data collection methods with your research questions and the nature of the information you’re seeking.
Qualitative Data Coding Techniques
Coding is the heart of qualitative data analysis. It’s the process of labeling and organizing your qualitative data to identify different themes and the relationships between them.
In my workshops, I emphasize the importance of developing a clear, consistent coding system.
This might involve open coding to identify initial concepts, axial coding to make connections between categories, and selective coding to integrate and refine the theory.
The goal is to transform raw data into meaningful, analyzable units.
Developing Themes and Patterns
Once your data is coded, the next step is to look for overarching themes and patterns. This is where the analytical magic happens.
It’s about stepping back from the details and seeing the bigger picture. In my work with companies like Motorola and HP, I’ve found that visual tools like mind maps or thematic networks can be incredibly helpful in this process.
They allow you to see connections and hierarchies within your data that might not be immediately apparent in text form.
Data Interpretation and Theory Building
The final step in the qualitative data analysis process is interpretation and theory building.
This is where you bring together your themes and patterns to construct a coherent narrative or theory that answers your research questions.
It’s crucial to remain grounded in your data while also being open to new insights. In my experience, the best interpretations often challenge our initial assumptions and lead to innovative solutions.
Tools and Software for Qualitative Analysis
Discover the power of CAQDAS in streamlining qualitative data analysis workflows. Explore popular tools like NVivo, ATLAS.ti, and MAXQDA for efficient data management and analysis.
Overview of CAQDAS (Computer Assisted Qualitative Data Analysis Software)
Computer Assisted Qualitative Data Analysis Software (CAQDAS) has revolutionized the way we approach qualitative analysis.
These tools streamline the coding process, help manage large datasets, and offer sophisticated visualization options.
As someone who’s seen the evolution of these tools over the past two decades, I can attest to their transformative power.
They allow researchers to handle much larger datasets and perform more complex analyses than ever before.
Popular Tools: NVivo, ATLAS.ti, MAXQDA
Among the most popular CAQDAS tools are NVivo, ATLAS.ti, and MAXQDA.
Each has its strengths, and the choice often depends on your specific needs and preferences. NVivo, for instance, offers robust coding capabilities and is excellent for managing multimedia data.
ATLAS.ti is known for its intuitive interface and powerful network view feature. MAXQDA stands out for its mixed methods capabilities, blending qualitative and quantitative approaches seamlessly.
Ensuring Rigor in Qualitative Data Analysis
Implement strategies like data triangulation, member checking, and audit trails to enhance credibility. Understand the importance of reflexivity in maintaining objectivity throughout the research process.
Data triangulation methods
Ensuring rigor in qualitative analysis is crucial for producing trustworthy results.
Data triangulation is a powerful method for enhancing the credibility of your findings. It involves using multiple data sources, methods, or investigators to corroborate your results.
In my Six Sigma projects, I often employ methodological triangulation, combining interviews, observations, and document analysis to get a comprehensive view of a process or problem.
Member Checking for Validity
Member checking is another important technique for ensuring the validity of your qualitative analysis.
This involves taking your findings back to your participants to confirm that they accurately represent their experiences and perspectives.
In my work with various organizations, I’ve found that this not only enhances the credibility of the research but also often leads to new insights as participants reflect on the findings.
Creating an Audit Trail
An audit trail is essential for demonstrating the rigor of your qualitative analysis.
It’s a detailed record of your research process, including your raw data, analysis notes, and the evolution of your coding scheme.
Practicing Reflexivity
Reflexivity is about acknowledging and critically examining your own role in the research process. As researchers, we bring our own biases and assumptions to our work.
Practicing reflexivity involves constantly questioning these assumptions and considering how they might be influencing our analysis.
Challenges and Best Practices in Qualitative Data Analysis
Address common hurdles such as data saturation, researcher bias, and ethical considerations. Learn best practices for conducting rigorous and ethical qualitative research in various contexts.
Dealing with data saturation
One of the challenges in qualitative research is knowing when you’ve reached data saturation ā the point at which new data no longer brings new insights.
In my experience, this requires a balance of systematic analysis and intuition. It’s important to continuously review and compare your data as you collect it.
In projects I’ve led, we often use data matrices or summary tables to track emerging themes and identify when we’re no longer seeing new patterns emerge.
Overcoming Researcher Bias
Researcher bias is an ever-present challenge in qualitative analysis. Our own experiences and preconceptions can inadvertently influence how we interpret data.
To overcome this, I advocate for a combination of strategies. Regular peer debriefing sessions, where you discuss your analysis with colleagues, can help uncover blind spots.
Additionally, actively seeking out negative cases or contradictory evidence can help challenge your assumptions and lead to more robust findings.
Ethical Considerations in Qualitative Research
Ethical considerations are paramount in qualitative research, given the often personal and sensitive nature of the data.
Protecting participant confidentiality, ensuring informed consent, and being transparent about the research process are all crucial.
In my work across various industries and cultures, I’ve learned the importance of being sensitive to cultural differences and power dynamics.
It’s also vital to consider the potential impact of your research on participants and communities.
Ethical qualitative research is not just about following guidelines, but about constantly reflecting on the implications of your work.
The Future of Qualitative Data Analysis
As we look to the future of qualitative data analysis, several exciting trends are emerging.
The increasing use of artificial intelligence and machine learning in qualitative analysis tools promises to revolutionize how we handle large datasets.
We’re also seeing a growing interest in visual and sensory methods of data collection and analysis, expanding our understanding of qualitative data beyond text.
In conclusion, mastering qualitative data analysis is an ongoing journey. It requires a combination of rigorous methods, creative thinking, and ethical awareness.
As we move forward, the field will undoubtedly continue to evolve, but its fundamental importance in research and decision-making will remain constant.
For those willing to dive deep into the complexities of qualitative data, the rewards in terms of insights and understanding are immense.
SixSigma.us offers both Live Virtual classes as well as Online Self-Paced training. Most option includes access to the same great Master Black Belt instructors that teach our World Class in-person sessions. Sign-up today!
Virtual Classroom Training Programs Self-Paced Online Training Programs